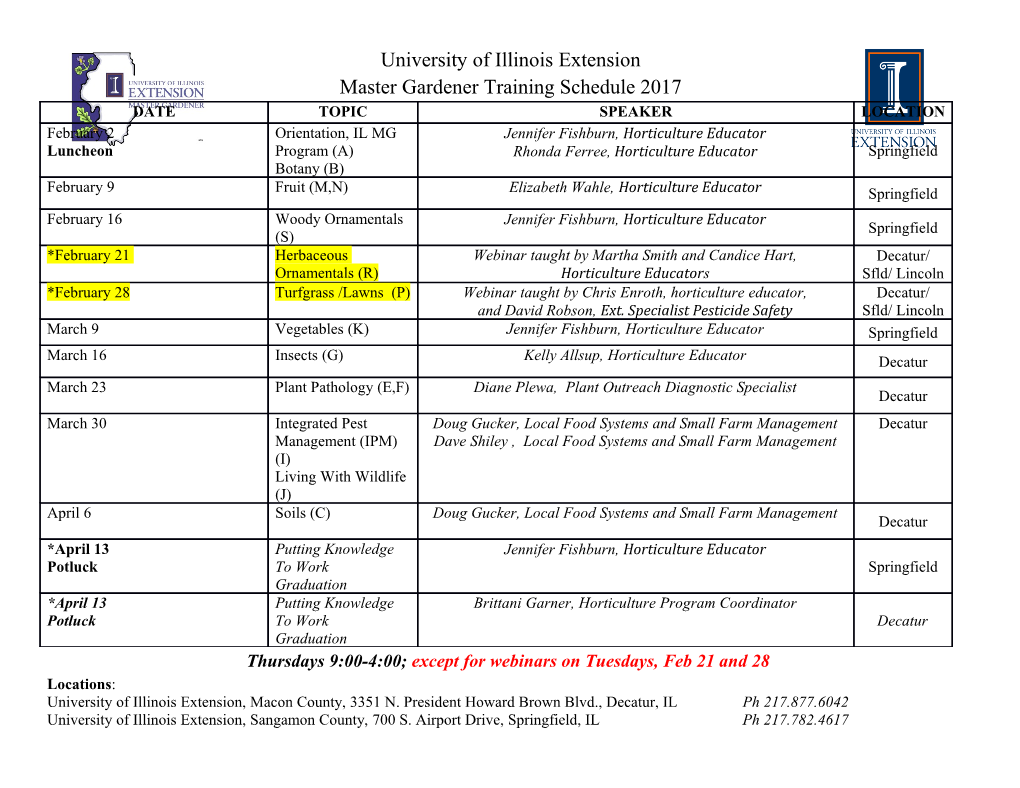
' $ Generalized Linear Models Objectives: ² Systematic + Random. ² Exponential family. ² Maximum likelihood estimation & inference. & % 45 Heagerty, Bio/Stat 571 ' $ Generalized Linear Models ² Models for independent observations Yi, i = 1; 2; : : : ; n. ² Components of a GLM: . Random component Yi » f(Yi; θi;Á) f 2 exponential family & % 46 Heagerty, Bio/Stat 571 ' $ . Systematic component ´i = Xi¯ ´i : linear predictor Xi : (1 £ p) covariate vector ¯ :(p £ 1) regression coe±cient . Link function E(Yi j Xi) = ¹i g(¹i) = Xi¯ g(¢): link function & % 47 Heagerty, Bio/Stat 571 ' $ Generalized Linear Models ² GLMs generalize the standard linear model: Yi = Xi¯ + ²i . Random: Normal distribution 2 ²i »N (0; σ ) . Systematic: linear combination of covariates ´i = Xi¯ . Link: identity function ´i = ¹i & % 48 Heagerty, Bio/Stat 571 ' $ Generalized Linear Models ² GLMs extend usefully to overdispersed and correlated data: . GEE: marginal models / semi-parametric estimation & inference . GLMM: conditional models / likelihood estimation & inference & % 49 Heagerty, Bio/Stat 571 ' $ Exponential Family · ¸ yθ ¡ b(θ) (?) f(y; θ; Á) = exp + c(y; Á) a(Á) θ = canonical parameter Á = ¯xed (known) scale parameter Properties: If Y » f(y; θ; Á) in (?) then, E(Y ) = ¹ = b0(θ) var(Y ) = b00(θ) ¢ a(Á) & % 50 Heagerty, Bio/Stat 571 ' $ Canonical link function: A function g(¢) such that: ´ = g(¹) = θ (canonical parameter) Variance function: A function V (¢) such that: var(Y ) = V (¹) ¢ a(Á) Usually : a(Á) = Á ¢ w Á \scale" parameter w weight & % 51 Heagerty, Bio/Stat 571 ' $ Examples of GLMS: logistic regression y = s=m where s=number of successes / m trials µ ¶ m f(y; θ; Á) = ¼s(1 ¡ ¼)m¡s s · µ ¶¸ y ¢ log( ¼ ) + log(1 ¡ ¼) m = exp 1¡¼ + log 1=m s =) θ = log(¼=(1 ¡ ¼)) b(θ) = ¡ log(1 ¡ ¼) = log[1 + exp(θ)] @ ¹ = b0(θ) = log[1 + exp(θ)] @θ = exp(θ)=[1 + exp(θ)] = ¼ & % 52 Heagerty, Bio/Stat 571 ' $ g(¹) = log[¼=(1 ¡ ¼)] = θ g : logit; log-odds function 1 var(y) = ¼(1 ¡ ¼) ¢ m V (¹) = a(Á) = & % 53 Heagerty, Bio/Stat 571 ' $ ² Poisson regression y = number of events (count) f(y; θ; Á) = ¸y exp(¡¸)=y! = exp [y ¢ log(¸) ¡ ¸ ¡ log(y!)] =) θ = log(¸) b(θ) = ¸ = exp(θ) ¹ = b0(θ) = exp(θ) = ¸ g(¹) = θ = log(¹) g : canonical link is log & % 54 Heagerty, Bio/Stat 571 ' $ ² Poisson regression (continued) var(y) = ¸ V (¹) = a(Á) = ± Other examples: . gamma, inverse Gaussian (MN, Table 2.1) . some survival models (MN, Chpt. 13) & % 55 Heagerty, Bio/Stat 571 ' $ Example: Seizure data (DHLZ ex. 1.6) ² Clinical trial of progabide, evaluating impact on epileptic seizures. ² Data: . age = patient age in years . base = 8-week baseline seizure count (pre-tx) . tx = 0 if assigned placebo; 1 if assigned progabide . Y1;Y2;Y3;Y4 seizure counts in 4 two-week periods following treatment administration ² Models: . linear model: Y4 = age + base + tx + ² . Poisson GLM: log(¹4) = age + base + tx & % 56 Heagerty, Bio/Stat 571 ' $ Example: Seizure data (DHLZ ex. 1.6) linear regression Poisson regression est. s.e. Z est. s.e. Z (Int) -4.97 3.62 -1.37 0.778 0.285 2.73 age 0.12 0.11 1.07 0.014 0.009 1.64 base 0.31 0.03 11.79 0.022 0.001 20.27 tx -1.36 1.37 -0.99 -0.270 0.102 -2.66 ² Q: should we use log(base) for Poisson regression? ² Q: why does inference regarding signi¯cance of TX di®er? & % 57 Heagerty, Bio/Stat 571 • • 4 60 • • 50 • 3 • • • • • • • 40 • • • • • 2 • • • • • y4 • •• • • • • 30 • ••••• • • • • • • ••• •• • • 1 • log(y4 + 0.5) •• • 20 • • • • • • • • 0 10 • • • • • • ••• • • • • • • • ••••••••••• •• • • 0 ••••• • ••• • ••• • •• 20 40 60 80 100 120 140 20 40 60 80 100 120 140 base base 14 15 12 10 10 8 6 5 4 2 0 0 0 20 40 60 0 20 40 60 y4[trt == 0] y4[trt == 1] 58 Heagerty, Bio/Stat 571 Seizure Residuals vs. Fitted • • 20 • • 2.0 1.5 10 •• •• •• • • • • • • 1.0 •• •• • •• • • • • • •• • •• • •• •• • • • • •• • • • • • • • • •• • • • • •• 0.5 • lmfit$resid •• • • • glmfit$resid 0 •• • • • • • ••• • •• • • • • • • • • •• • • •••• • •• •••• • • •• • • • ••• •• •• • •• • • • • • • ••• • •• • • •• • 0.0 • • • •• •• • • • •••• • • •• •• • •• • • •• • ••• • •• ••••• •• •• • −10 • •• −0.5 •• •• • ••• • ••••• • −1.0 ••••• 0 10 20 30 40 10 20 30 40 50 60 lmfit$fitted glmfit$fitted 59 Heagerty, Bio/Stat 571 Seizure Residuals vs. Fitted, using predict() • 4 • 20 • • •• •• •• • 2 • 10 •• •• •• •• •• •• •• • • •• • • • • • • • • • • • • • • • • • • • • • •• •• • •• •• • •• • • •• • • • • •• • • •• •• •• • •• resid(lmfit) • • • • • 0 • resid(glmfit) 0 •• • • • • • • •• • •• • • • •• •• • • • • • •• • •• •• • • ••• • •• •••• • ••• • • • ••• ••• • •••• • • ••• • • • • • • • •• • • ••• • •• • •• • • •• •• ••• •• • •• •• • • • −2 • −10 • •• •• •• • • •• •••• •• •• •• 0 10 20 30 40 1.0 1.5 2.0 2.5 3.0 3.5 4.0 predict(lmfit) predict(glmfit) 60 Heagerty, Bio/Stat 571 ' $ Residual Diagnostics ± Used to assess model ¯t similarly as for linear models ² Q-Q plots for residuals (may be hard to interpret for discrete data ) ² residual plots: ? vs. ¯tted values ? vs. omitted covariates ² assessment of systematic departures ² assessment of variance function & % 61 Heagerty, Bio/Stat 571 ' $ Residual Diagnostics ± Types of residuals for GLMs: 1. Pearson residual y ¡ ¹b rP = pi i V (¹bi) X P 2 2 (ri ) = X 2. Deviance residual (see resid(fit)) p D r = sign(y ¡ ¹b) di X i D 2 (ri ) = D(y; ¹b) 3. Working residual (see fit$resid) W @´bi ri = (yi ¡ ¹bi) = Zi ¡ ´bi @¹bi & % 62 Heagerty, Bio/Stat 571 ' $ Fitting GLMS by Maximum Likelihood Solve score equations: @ Uj(¯) = log L = 0 j = 1; 2; : : : ; p @¯j log-likelihood: · ¸ Xn y ¢ θ ¡ b(θ ) log L = i i i + c(y ;Á) a (Á) i i=1 i X = log Li =) @ log L X @ log L @θ @¹ @´ U (¯) = = i ¢ i ¢ i ¢ i j @¯ @θ @¹ @´ @¯ j i i i i j & % 63 Heagerty, Bio/Stat 571 ' $ @ log Li 1 0 1 = (yi ¡ b (θi)) = (yi ¡ ¹i) @θi ai(Á) ai(Á) µ ¶¡1 @θi @¹i = = 1=V (¹i) @¹i @θi @´i = Xij @¯j Therefore, µ ¶ Xn @¹ U (¯) = X i ¢ [a (Á) ¢ V (¹ )]¡1 (Y ¡ ¹ ) j ij @´ i i i i i=1 i & % 64 Heagerty, Bio/Stat 571 ' $ GLM Information Matrix ² Either form: [In](j; k) = cov[Uj(¯);Uk(¯)] µ ¶ @2 log L = ¡E @¯j@¯k ² Let's consider the second form... & % 65 Heagerty, Bio/Stat 571 ' $ GLM Information Matrix · ¸ @ [In](j; k) = ¡E Uj(¯) @¯k " ½µ ¶ ¾# Xn @ @¹ = ¡E i ¢ [a (Á) ¢ V (¹ )]¡1 (Y ¡ ¹ ) ¯ @¯ i i i i i=1 k j µ ¶ µ ¶ Xn @¹ @¹ = i ¢ [a (Á) ¢ V (¹ )]¡1 i @¯ i i @¯ i=1 j k justify & % 66 Heagerty, Bio/Stat 571 ' $ Score and Information ² In vector/matrix form we have: 0 1 U1(¯) B C B C B U2(¯) C U(¯) = B C B . C @ . A Up(¯) ³ ´ @¹i = @¹i @¹i ::: @¹i @¯ @¯1 @¯2 @¯p @¹i = Xi @´i & % 67 Heagerty, Bio/Stat 571 ' $ Score and Information µ ¶ Xn @¹ T U(¯) = i ¢ [a (Á) ¢ V (¹ )]¡1 (Y ¡ ¹ ) @¯ i i i i i=1 and µ ¶ µ ¶ Xn @¹ T @¹ I = i ¢ [a (Á) ¢ V (¹ )]¡1 i n @¯ i i @¯ i=1 & % 68 Heagerty, Bio/Stat 571 ' $ Fisher Scoring Goal: Solve the score equations U(¯) = 0 Iterative estimation is required for most GLMs. The score equations can be solved using Newton-Raphson (uses observed derivative of score) or Fisher Scoring which uses the expected derivative of the score (ie. ¡In). & % 69 Heagerty, Bio/Stat 571 ' $ Fisher Scoring Algorithm: (0) ² Pick an initial value: ¯b . (j) ² For j ! (j + 1) update ¯b via ³ ´¡1 b(j+1) b(j) b(j) b(j) ¯ = ¯ + In U(¯ ) (j+1) (j) ² Evaluate convergence using changes in log L or k¯b ¡ ¯b k. ² Iterate until convergence criterion is satis¯ed. & % 70 Heagerty, Bio/Stat 571 ' $ Comments on Fisher Scoring: 1. IWLS is equivalent to Fisher Scoring (Biostat 570). 2. Observed and expected information are equivalent for canonical links. 3. Score equations are an example of an estimating function (more on that to come!) 4. Q: What assumptions make E[U(¯)] = 0? P T 5. Q: What is the relationship between In and U iU i ? 6. Q: What is a 1-step approximation to ¢¯(¡i)? & % 71 Heagerty, Bio/Stat 571 ' $ Inference for GLMs Review of asymptotic likelihood theory: 0 1 ¯ (q £ 1) B 1 C B C ¯ = @ ¡¡¡ A = ¡¡¡ ¯2 (p ¡ q £ 1) 0 Goal: Test H0 : ¯2 = ¯2 (1) Likelihood Ratio Test: h i b b b0 0 2 2 log L(¯1; ¯2) ¡ log L(¯1; ¯2) »  (df = p ¡ q) & % 72 Heagerty, Bio/Stat 571 ' $ Inference for GLMs (2) Score Test: 0 1 U 1(¯ ) (q £ 1) B 1 C B C U(¯) = @ ¡¡¡ A = ¡¡¡ U 2(¯2) (p ¡ q £ 1) n o¡1 b0 T b0 b0 2 U 2(¯ ) cov[U 2(¯ )] U 2(¯ ) »  (df = p ¡ q) (3) Wald Test: n o¡1 b 0 T b b 0 2 (¯2 ¡ ¯2) cov(¯2) (¯2 ¡ ¯2) »  (df = p ¡ q) & % 73 Heagerty, Bio/Stat 571 ' $ Measures of Discrepancy There are 2 primary measures: ² deviance ² Pearson's X2 Deviance: Assume ai(Á) = Á=mi (eg. normal: Á; binomial: 1=mi; Poisson: 1) Xn b b log L(¯) = log fi(yi; θi;Á) i=1 ½ ¾ X m = i [y θb ¡ b(θb )] + c (y ;Á) Á i i i i i i & % 74 Heagerty, Bio/Stat 571 ' $ Now consider log L as a function of ¹b, using the relationship b0(θ) = ¹: ½ ¾ X m l(¹b;Á; y) = i [y ¢ θ(¹b ) ¡ b[θ(¹b )] ] + c (y ;Á) Á i i i i i i The deviance is: D(y; ¹b) = 2 ¢ Á ¢ [l(y;Á; y) ¡ l(¹b;Á; y)] X = 2 ¢ mi f yi ¢ [θ(yi) ¡ θ(¹bi)] ¡ i ( b[θ(yi)] ¡ b[θ(¹bi)] ) g & % 75 Heagerty, Bio/Stat 571 ' $ Deviance Deviance generalizes the residual sum of squares for linear models: Model 1 Model 2 0 1 0 1 ¯b (q £ 1) ¯b B 1 C B 1 C B C B C @ ¡¡¡ A ¡¡¡ @ ¡¡¡ A b 0 ¯2 (p ¡ q £ 1) ¯2 ¹b1 ¹b2 & % 76 Heagerty, Bio/Stat 571 ' $ Deviance Linear Model: SSE(Model 2) ¡ SSE(Model 1) » Â2(df = p ¡ q) σ2 GLM: D(y; ¹b ) ¡ D(y; ¹b ) 2 1 » Â2(df = p ¡ q) Á & % 77 Heagerty, Bio/Stat 571 ' $ Examples: 2 (yi¡¹i) 1.
Details
-
File Typepdf
-
Upload Time-
-
Content LanguagesEnglish
-
Upload UserAnonymous/Not logged-in
-
File Pages39 Page
-
File Size-