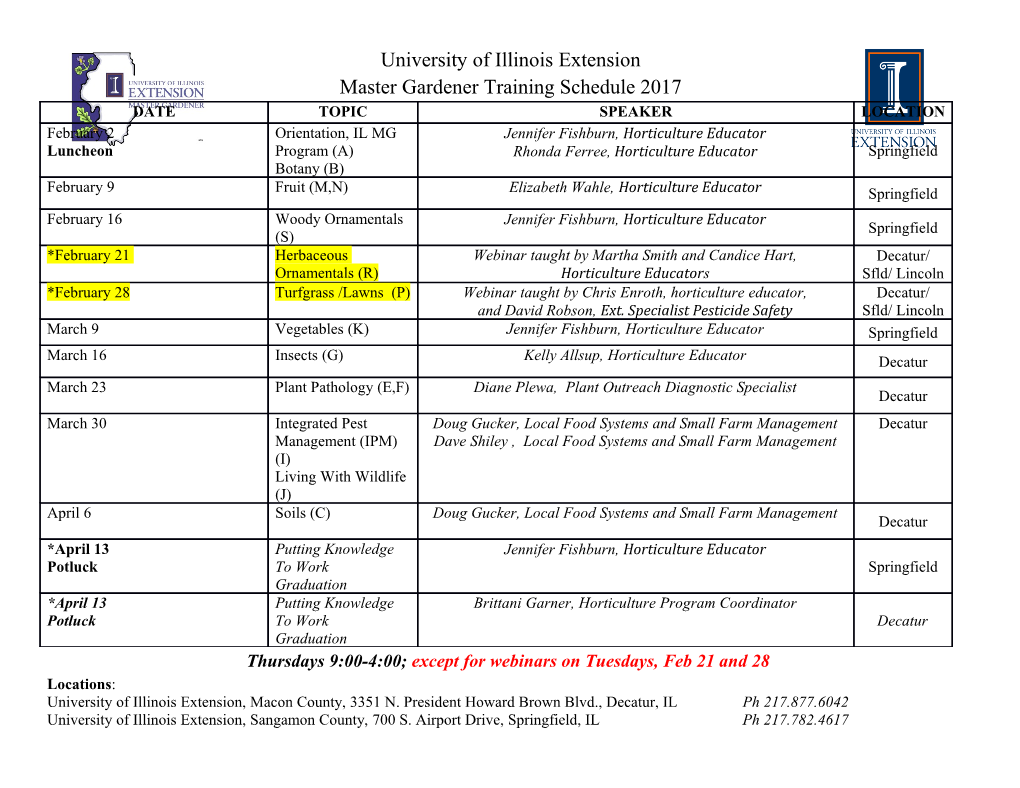
Advanced stochastic processes: Part II Jan A. Van Casteren Download free books at Jan A. Van Casteren Advanced stochastic processes Part II ii Download free eBooks at bookboon.com Advanced stochastic processes: Part II 2nd edition © 2015 Jan A. Van Casteren & bookboon.com ISBN 978-87-403-1116-7 iii Download free eBooks at bookboon.com Advanced stochastic processes: Part II Contents Contents Contents PrefaceTo See Part 1 download: Advanced stochastic processes: Part 1 i PrefaceChapter 1. Stochastic processes: prerequisites 1i 1. Conditional expectation 2 Chapter2. Lemma 1. Stochastic of Borel-Cantelli processes: prerequisites 91 3.1. StochasticConditional processes expectation and projective systems of measures 102 4.2. ALemma definition of Borel-Cantelli of Brownian motion 169 5.3. MartingalesStochastic processes and related and processes projective systems of measures 1710 4. A definition of Brownian motion 16 Chapter5. Martingales 2. Renewal and theory related and processes Markov chains 1735 1. Renewal theory 35 Chapter2. Some 2. additional Renewal theory comments and Markov on Markov chains processes 6135 3.1. MoreRenewal on Browniantheory motion 7035 4.2. GaussianSome additional vectors. comments on Markov processes 7661 5.3. Radon-NikodymMore on Brownian Theorem motion 7870 6.4. SomeGaussian martingales vectors. 7876 5. Radon-Nikodym Theorem 78 Chapter6. Some 3. martingales An introduction to stochastic processes: Brownian motion, 78 Gaussian processes and martingales 89 Chapter1. Gaussian 3. An processes introduction to stochastic processes: Brownian motion, 89 2. BrownianGaussian motion processes and related and processes martingales 9889 3.1. SomeGaussian results processes on Markov processes, on Feller semigroups and on the 89 2. Brownianmartingale motion problem and related processes 11798 4.3. Martingales,Some results submartingales, on Markov processes, supermartingales on Feller semigroups and semimartingales and on the 147 5. Regularitymartingale properties problem of stochastic processes 151117 www.sylvania.com 6.4. StochasticMartingales, integrals, submartingales, Itˆo’sformula supermartingales and semimartingales162 147 7.5. Black-ScholesRegularity properties model of stochastic processes 188151 8.6. AnStochastic Ornstein-Uhlenbeck integrals, Itˆo’sformula process in higher dimensions 197162 9.7. ABlack-Scholes version of Fernique’s model theorem We do not reinvent221188 10.8. An Miscellaneous Ornstein-Uhlenbeck process in higher dimensions 223197 9. A version of Fernique’s theorem the wheel we reinvent221 Index 237 10. Miscellaneous light. 223 IndexChapter 4. Stochastic differential equations 237243 1. Solutions to stochastic differential equationsFascinating lighting offers an infinite243 spectrum of Chapter 4. Stochastic differential equations possibilities: Innovative technologies243 and new 2. A martingale representation theorem markets provide both opportunities 272and challenges. 3.1. GirsanovSolutions transformation to stochastic differential equationsAn environment in which your expertise277243 is in high 2. A martingale representation theorem demand. Enjoy the supportive working272 atmosphere Chapter3. Girsanov 5. Some transformation related results within our global group and benefit 277295from international 1. Fourier transforms career paths. Implement sustainable295 ideas in close cooperation with other specialists and contribute to Chapter2. Convergence 5. Some related of positive results measures influencing our future. Come and join324295 us in reinventing 1. Fourier transforms iii light every day. 295 2. Convergence of positive measures 324 iii Light is OSRAM iv Click on the ad to read more Download free eBooks at bookboon.com Contents Preface i Chapter 1. Stochastic processes: prerequisites 1 1. Conditional expectation 2 2. Lemma of Borel-Cantelli 9 3. Stochastic processes and projective systems of measures 10 4. A definition of Brownian motion 16 5. Martingales and related processes 17 Chapter 2. Renewal theory and Markov chains 35 1. Renewal theory 35 2. Some additional comments on Markov processes 61 3. More on Brownian motion 70 4. Gaussian vectors. 76 Advanced5. Radon-Nikodym stochastic processes: Theorem Part II 78 Contents 6. Some martingales 78 Chapter 3. An introduction to stochastic processes: Brownian motion, Gaussian processes and martingales 89 1. Gaussian processes 89 2. Brownian motion and related processes 98 3. Some results on Markov processes, on Feller semigroups and on the martingale problem 117 4. Martingales, submartingales, supermartingales and semimartingales 147 5. Regularity properties of stochastic processes 151 6. Stochastic integrals, Itˆo’sformula 162 7. Black-Scholes model 188 8. An Ornstein-Uhlenbeck process in higher dimensions 197 9. A version of Fernique’s theorem 221 10. Miscellaneous 223 360° Index 237 Chapter 4. Stochastic differential equations 243 1. Solutions to stochastic differential360° equations 243 thinking. 2. A martingale representation theorem 272 3. Girsanov transformation 277 Chapter 5. Some related results thinking. 295 1. Fourier transforms 295 2. Convergence of positive measures 324 iii 360° thinking . 360° thinking. Discover the truth at www.deloitte.ca/careers Discover the truth at www.deloitte.ca/careers © Deloitte & Touche LLP and affiliated entities. Discover the truth at www.deloitte.ca/careers © Deloitte & Touche LLP and affiliated entities. © Deloitte & Touche LLP and affiliated entities. Discover the truthv at www.deloitte.ca/careersClick on the ad to read more Download free eBooks at bookboon.com © Deloitte & Touche LLP and affiliated entities. Contents Preface i Chapter 1. Stochastic processes: prerequisites 1 1. Conditional expectation 2 2. Lemma of Borel-Cantelli 9 3. Stochastic processes and projective systems of measures 10 4. A definition of Brownian motion 16 5. Martingales and related processes 17 Chapter 2. Renewal theory and Markov chains 35 1. Renewal theory 35 2. Some additional comments on Markov processes 61 3. More on Brownian motion 70 4. Gaussian vectors. 76 5. Radon-Nikodym Theorem 78 6. Some martingales 78 Chapter 3. An introduction to stochastic processes: Brownian motion, Gaussian processes and martingales 89 1. Gaussian processes 89 2. Brownian motion and related processes 98 3. Some results on Markov processes, on Feller semigroups and on the martingale problem 117 4. Martingales, submartingales, supermartingales and semimartingales 147 5. Regularity properties of stochastic processes 151 6. Stochastic integrals, Itˆo’sformula 162 7. Black-Scholes model 188 8. An Ornstein-Uhlenbeck process in higher dimensions 197 9. A version of Fernique’s theorem 221 Advanced10. Miscellaneous stochastic processes: Part II 223 Contents Index 237 Chapter 4. Stochastic differential equations 243 1. Solutions to stochastic differential equations 243 2. A martingale representation theorem 272 3. Girsanov transformation 277 ivChapter 5. Some related results CONTENTS 295 1. Fourier transforms 295 2. Convergence of positive measures 324 3. A taste of ergodic theory iii 340 4. Projective limits of probability distributions 357 5. Uniform integrability 369 6. Stochastic processes 373 7. Markov processes 399 8. The Doob-Meyer decomposition via Komlos theorem 409 Subjects for further research and presentations 423 Bibliography 425 Index 433 We will turn your CV into an opportunity of a lifetime Do you like cars? Would you like to be a part of a successful brand? Send us your CV on We will appreciate and reward both your enthusiasm and talent. www.employerforlife.com Send us your CV. You will be surprised where it can take you. vi Click on the ad to read more Download free eBooks at bookboon.com Advanced stochastic processes: Part II Stochastic differential equations CHAPTER 4 Stochastic differential equations Some pertinent topics in the present chapter consist of a discussion on mar- tingale theory, and a few relevant results on stochastic differential equations in spaces of finite dimension. In particular unique weak solutions to stochastic dif- ferential equations give rise to strong Markov processes whose one-dimensional distributions are governed by the corresponding second order parabolic type differential equation. Essentially speaking this chapter is part of Chapter 1 in [146]. (The author is thankful to WSPC for the permission to include this text also in the present book.) In this chapter we discuss weak and strong solutions to stochastic differential equations. We also discuss a version of the Girsanov transformation. 1. Solutions to stochastic differential equations Basically, the material in this section is taken from Ikeda and Watanabe [61]. In Subsection 1.1 we begin with a discussion on strong solutions to stochastic differential equations, after that, in Subsection 1.2 we present a martingale characterization of Brownian motion. We also pay some attention to (local) exponential martingales: see Subsection 1.3. In Subsection 1.4 the notion of weak solutions is explained. However, first we give a definition of Brownian motion which starts at a random position. 4.1. Definition. Let Ω, F, P be a probability space with filtration Ft t 0. A d-dimensional Brownianp motionq is a almost everywhere continuous adaptedp q ě process B t B1 t ,...,Bd t : t 0 such that for 0 t1 t2 t p q“p p q p qq ě du n ă ă ă ¨¨¨ ă tn and for C any Borel subset of R the following equality holds: ă8 P B t1 B 0 ,...,B tn B 0 C rp p q´ p q p q´ p` qq˘ P s p0,d tn tn 1,xn 1,xn p0,d t2 t1,x1,x2 p0,d t1, 0,x1 “ ¨¨¨ p ´ ´ ´ q¨¨¨ p ´ q p q ż C ż dx1 ...dxn. (4.1) This process is called a d-dimensional Brownian motion with initial distribution d n 1 µ if for 0 t1 t2 tn and every Borel subset of R ` the following equalityă ă holds:㨨¨ă ă8 ` ˘ P B 0 ,B t1 ,...,B tn C rp p q p q p qq P s p0,d tn tn 1,xn 1,xn p0,d t2 t1,x1,x2 p0,d t1,x0,x1 “ ¨¨¨ p ´ ´ ´ q¨¨¨ p ´ q p q ż C ż 243 243 Download free eBooks at bookboon.com Advanced stochastic processes: Part II Stochastic differential equations 244 4. STOCHASTIC DIFFERENTIAL EQUATIONS dµ x dx ...dx . (4.2) p 0q 1 n For the definition of p t,x,y see formula (4.26). By definition a filtration 0,d p q Ft t 0 is an increasing family of σ-fields, i.e.
Details
-
File Typepdf
-
Upload Time-
-
Content LanguagesEnglish
-
Upload UserAnonymous/Not logged-in
-
File Pages202 Page
-
File Size-