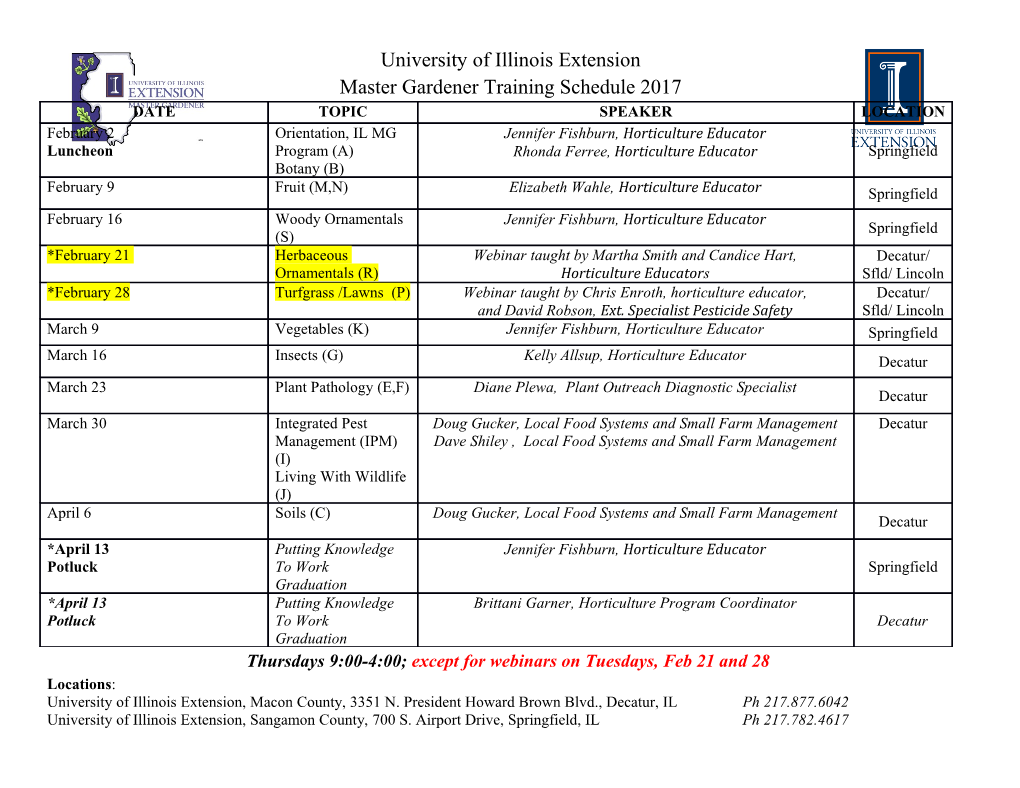
Approximation theory in neural networks Yanhui Suy yanhui [email protected] March 30, 2018 Outline Functions Functionals Operators Bounds Optimal Outline 1 Approximation of functions by a sigmoidal function 2 Approximations of continuous functionals by a sigmoidal function 3 Universal approximation by neural networks with arbitrary activation functions 4 Universal approximation bounds for superpositions of a sigmoidal function 5 Optimal approximation with sparsely connected deep neural networks Outline Functions Functionals Operators Bounds Optimal Outline 1 Approximation of functions by a sigmoidal function 2 Approximations of continuous functionals by a sigmoidal function 3 Universal approximation by neural networks with arbitrary activation functions 4 Universal approximation bounds for superpositions of a sigmoidal function 5 Optimal approximation with sparsely connected deep neural networks Outline Functions Functionals Operators Bounds Optimal Outline 1 Approximation of functions by a sigmoidal function 2 Approximations of continuous functionals by a sigmoidal function 3 Universal approximation by neural networks with arbitrary activation functions 4 Universal approximation bounds for superpositions of a sigmoidal function 5 Optimal approximation with sparsely connected deep neural networks Outline Functions Functionals Operators Bounds Optimal Outline 1 Approximation of functions by a sigmoidal function 2 Approximations of continuous functionals by a sigmoidal function 3 Universal approximation by neural networks with arbitrary activation functions 4 Universal approximation bounds for superpositions of a sigmoidal function 5 Optimal approximation with sparsely connected deep neural networks Outline Functions Functionals Operators Bounds Optimal Outline 1 Approximation of functions by a sigmoidal function 2 Approximations of continuous functionals by a sigmoidal function 3 Universal approximation by neural networks with arbitrary activation functions 4 Universal approximation bounds for superpositions of a sigmoidal function 5 Optimal approximation with sparsely connected deep neural networks Outline Functions Functionals Operators Bounds Optimal Outline 1 Approximation of functions by a sigmoidal function 2 Approximations of continuous functionals by a sigmoidal function 3 Universal approximation by neural networks with arbitrary activation functions 4 Universal approximation bounds for superpositions of a sigmoidal function 5 Optimal approximation with sparsely connected deep neural networks Outline Functions Functionals Operators Bounds Optimal Basic Notations 1 d: dimension of input layer; 2 L: number of layers; 3 Nl: number of neuros in the lth layers, l = 1; ··· ;L; 4 ρ : R ! R: activation function; N N 5 Wl : R l−1 ! R l ; 1 ≤ l ≤ L, x ! Alx + bl; 6 (Al)ij; (bl)i: the networks weights; Definition 1 d L A map Φ: R ! R given by d Φ(x) = WLρ(WL−1ρ(··· ρ(W1(x)))); x 2 R ; is called a neural network. Outline Functions Functionals Operators Bounds Optimal Basic Notations Outline Functions Functionals Operators Bounds Optimal A classical result of Cybenko 1; x ! +1 We say the σ is sigmoidal if σ(x) ! : 0; x ! −∞ A classical result on approximation of neural networks is: Theorem 2 (Cybenko [6]) Let σ be any continuous sigmoidal function. Then finite sums of the form N X G(x) = αjσ(yj · x + θj) (1) j=1 are dense in C(Id). In [5], T.P. Chen, H. Chen and R.W. Liu gave a constructive proof which only assume that σ is bounded sigmoidal function. Outline Functions Functionals Operators Bounds Optimal Outline 1 Approximation of functions by a sigmoidal function 2 Approximations of continuous functionals by a sigmoidal function 3 Universal approximation by neural networks with arbitrary activation functions 4 Universal approximation bounds for superpositions of a sigmoidal function 5 Optimal approximation with sparsely connected deep neural networks Outline Functions Functionals Operators Bounds Optimal Approximations of continuous functionals on Lp space Theorem 3 (Chen and Chen [3]) Suppose that U is a compact set in Lp[a; b](1 < p < 1), f is a continuous functional defined on U, and σ(x) is a bounded sigmoidal function, then for any " > 0, there exist h > 0, a positive integer m, m + 1 points a = x0 < x1 < ··· < xm = b, xj = a + j(b − a)=m, j = 0; 1; ··· ; m, a positive integer N and constants ci, θi, ξi;j, i = 1; ··· ;N, j = 0; 1; ··· ; m, such that N 0 m 1 Z xj +h X X 1 f(u) − ciσ @ ξi;j u(t)dt + θiA < " 2h i=1 j=0 xj −h holds for all u 2 U. Here it is assumed that u(x) = 0, if x2 = [a; b]. Outline Functions Functionals Operators Bounds Optimal Approximations of continuous functionals on C[a; b] Theorem 4 (Chen and Chen [3]) Suppose that U is a compact set in C[a; b], f is a continuous functional defined on U, and σ(x) is a bounded sigmoidal function, then for any " > 0, there exist m + 1 points a = x0 < ··· < xm = b, a positive integer N and constants ci, θi, ξi;j, i = 1; ··· ;N, j = 0; 1; ··· ; m, such that for any u 2 U, 0 1 N m X X f(u) − ciσ @ ξi;ju(xj) + θiA < " i=1 j=0 Outline Functions Functionals Operators Bounds Optimal An example in dynamical system Suppose that the input u(x) and the output s(x) = G(u(x)) satisfies ds(x) = g(s(x); u(x); x); s(a) = s dx 0 where g satisfies Lipschitz condition, then Z x (Gu)(x) = s0 + g((Gu)(t); u(t); t)dt: a It can be shown that G is a continuous functional on C[a; b]. If the input set U ⊂ C[a; b] is compact, then the output at a specified time d can be approximated by N 0 m 1 X X ciσ @ ξi;ju(xi) + θiA : i=1 j=1 Outline Functions Functionals Operators Bounds Optimal Outline 1 Approximation of functions by a sigmoidal function 2 Approximations of continuous functionals by a sigmoidal function 3 Universal approximation by neural networks with arbitrary activation functions 4 Universal approximation bounds for superpositions of a sigmoidal function 5 Optimal approximation with sparsely connected deep neural networks Outline Functions Functionals Operators Bounds Optimal Approximation by Arbitrary Functions Definition 5 If a function g : R ! R satisfies that all the linear combinations of the form N X cig(λix + θi); λi; θi; ci 2 R; i = 1; ··· ;N i=1 are dense in every C[a; b], then g is called a Tauber-Wiener (TW) function. Theorem 6 0 (Chen and Chen [4]) Suppose that g(x) 2 C(R) \S (R), then g 2 (TW) if and only if g is not a polynomial. Outline Functions Functionals Operators Bounds Optimal Approximation by Arbitrary Functions Theorem 7 d (Chen and Chen [4]) Suppose that K is a compact set in R , U is a compact set in C(K), g 2 (TW), then for any " > 0, there are a d positive integer N, θi 2 R, !i 2 R , i = 1; ··· ;N, which are all independent of f 2 U and constants ci(f) depending on f, i = 1; ··· ;N, such that N X f(x) − ci(f)g(!i · x + θi) < " i=1 holds for all x 2 K, f 2 U. Moreover, every ci(f) is a continuous functional defined on U. Outline Functions Functionals Operators Bounds Optimal Approximation to functionals by Arbitrary Functions The following theorem can be viewed as a generalization of Theorem 4 of sigmoidal function case. Theorem 8 (Chen and Chen [4]) Suppose that g 2 (TW), X is a Banach space, K ⊂ X is a compact set, V is a compact set in C(K), f is a continuous functional defined on V . Then for any " > 0, there are positive integers N, m points x1; ··· ; xk 2 K, and constants ci; θi; ξij 2 R, i = 1; ··· ; N; j = 1; ··· ; m, such that 0 1 N m X X f(u) − cig @ ξiju(xj) + θiA < " i=1 j=1 holds for all u 2 V . Outline Functions Functionals Operators Bounds Optimal Approximation to operators by Arbitrary Functions Theorem 9 (Chen and Chen [4]) Suppose that g 2 (TW), X is a Banach d space, K1 ⊂ X, K2 ⊂ R are two compact sets. V is a compact set in C(K1), G is a nonlinear continuous operators, which maps V to C(K2). Then for any " > 0, there are a positive integers k k d M; N; m, constants ci ; ζk; ξij 2 R, points !k 2 R , xj 2 K1, i = 1; ··· ; M; k = 1; ··· ;N, j = 1; ··· ; m, such that 0 1 M N m X X k X k k G(u)(y) − ci g @ ξiju(xj) + θi A · g(!k · y + ζk) < " i=1 k=1 j=1 holds for all u 2 V , y 2 K2. Outline Functions Functionals Operators Bounds Optimal Outline 1 Approximation of functions by a sigmoidal function 2 Approximations of continuous functionals by a sigmoidal function 3 Universal approximation by neural networks with arbitrary activation functions 4 Universal approximation bounds for superpositions of a sigmoidal function 5 Optimal approximation with sparsely connected deep neural networks Outline Functions Functionals Operators Bounds Optimal Basic notations 1 F~(d!) = eiθ(!)F (d!): the Fourier distribution (i.e. d complex-valued measure) of a function f(x) on R , and Z f(x) = ei!·xF~(d!) (2) d 2 B: bounded set in R that contains f0g 3 ΓB: the set of functions f on B for which the representation (2) holds for x 2 B for some complex-valued measure F~(d!) for which R j!jF (d!) is finite. 4 ΓC;B: the set of all functions f in ΓB such that for some F~ representing f on B Z j!jBF (d!) ≤ C where j!jB = supx2B j! · xj. Outline Functions Functionals Operators Bounds Optimal Universal approximation bounds Theorem 10 (Barron [1]) For every function f in ΓC;B, every sigmoidal function σ, every probability measure µ, and every n ≥ 1, there exists a linear combination of sigmoidal functions fn(x), such that Z 2 2 (2C) (f¯(x) − fn(x)) µ(dx) ≤ B n where f¯(x) = f(x) − f(0).
Details
-
File Typepdf
-
Upload Time-
-
Content LanguagesEnglish
-
Upload UserAnonymous/Not logged-in
-
File Pages43 Page
-
File Size-