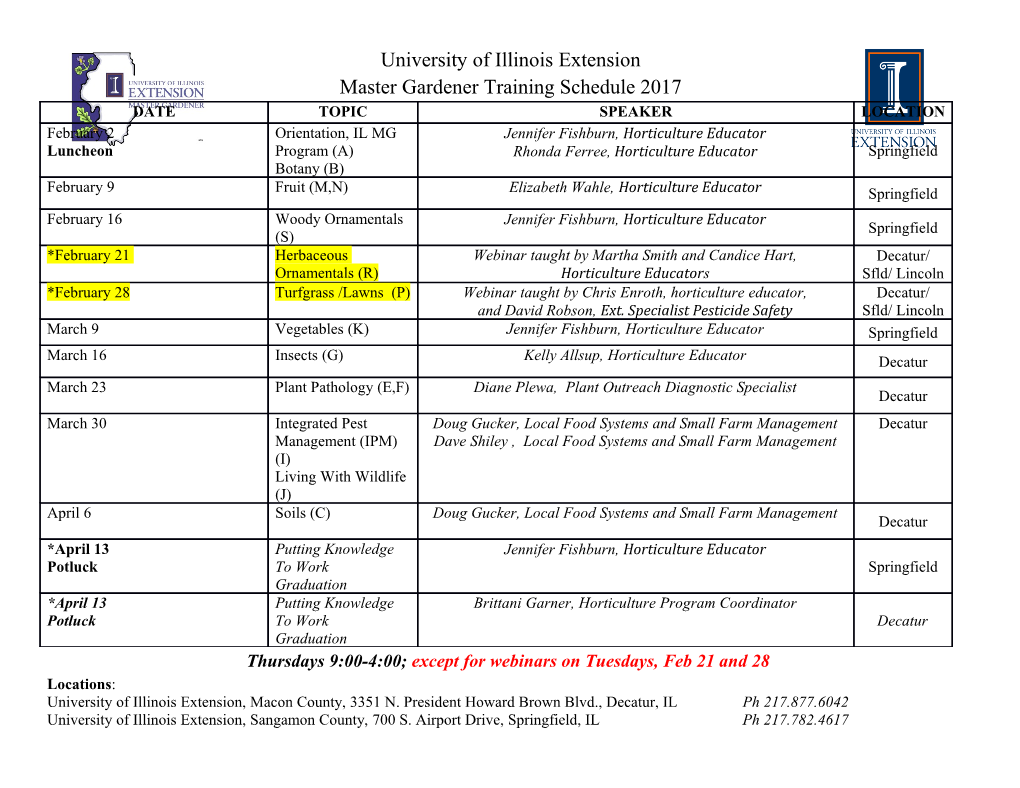
The 40th Asian Conference on Remote Sensing (ACRS 2019) October 14-18, 2019 / Daejeon Convention Center(DCC), Daejeon, Korea WeA4-2 Applications of Remote Sensing Indices for Property Price Estimation Sihao Ge(1),Shih-Yuan Lin(2) NCCU,NO.64, Sec.2, ZhiNan Rd., Wenshan, Taipei 11605, Taiwan 1 Postgraduate 2 Associate Professor Email:[email protected] ;[email protected] Abstract: In recent years, urbanization has been developed rapidly in China. Together with the trend the housing also increases significantly. Prosperous real estate market in Hangzhou City located in Zhejiang Province is a typical example and hence was selected as the study area. A total of 22,000 housing transaction records occurred covered from 2015 to 2019 were collected through property agent websites. The attributes include house price, house type, house area, orientation, building style, elevator, decoration, built era and sold time. Based on the data collected, the author aimed to establish a regression model to estimate the property price. To achieve this, in addition to the attributes listed above, the author also collected environmental factors extracted from multiple remote sensing images. The index obtained from the remote sensing data was put into the hedonic price model to perform the valuation operation. Key words: remote sensing, hedonic price model 1 Introduction Housing prices in China is s major social problems. In the past 30 years, China's real estate has developed rapidly. Hangzhou, as a big city in China, has also encountered high housing prices. With the improvement of the quality of life in China, people will pay more attention to the living environment. Therefore, the factors affecting housing prices are not only the population, economy, transportation, etc., but also the visual aspects such as landscape and greening. The distribution of house prices to be more time-sensitive and easy to interpret can be achieved through a combination of GIS (Geographic Information System) and RS (Remote Sensing). GIS can make the housing price data, RS technology can guarantee the timeliness of the data, and the combination of GIS and RS can make the housing price estimation more readable and time-sensitive, so that the public and the government can understand the price situation. The method of combining housing prices with images can be started as early as Forster (1983) in Sydney. He looks at the size of house through Landsat MSS, the results are predicted and displayed the same statistical results. The most important variable in this study is the number of rooms. Rossetti (2013) sees the price and postcode’s relationship through the enhanced vegetation index (EVI) indicator in Australia, area with high EVI index the house price is also higher. Franco et al. (2018) 1 In the study of Lisbon, the use of aerial camera image, combined with NDVI and SVM classify tree canopy technology combined with two-bedroom apartment price data estimates, prove that the canopy has a positive impact on housing prices. Jiao et al. (2017) Using Wuhan as a research area, Landsat ETM+ imagery was also used in conjunction with Wuhan's collective residential transaction price. The results showed that TEI and VCI showed House prices are negatively affected. 2 Research area and research data Hangzhou is a large city in eastern China. The resident population in 2018 is 9.8 million, and the urbanization rate of the resident population is 77.4%, close to 80% of the developed countries' standards. This research area including Shangcheng District, Xiacheng District, Jianggan District, Gongshu District, Xihu District, Binjiang District, Xiaoshan District and Yuhang District. Figure 1. Eight districts in Hangzhou City Many researchers have used Hangzhou as a sample to study the distribution of house prices with different methods and influencing factors. Fu Yi (2006) and Wen Haizhen et al. (2010) used the hedonic method to analyze the distribution of housing prices in Hangzhou. They all think distance to the west lake is the most important factor, which far away from the west lake the housing price is lower. Mao Fengqi et al. (2014) puts his attention on the key schools, they found middle school is the most important school, and kindergarten least influence. Lianjia is one of China’s biggest second-hand housing transaction intermediary, in which the Lianjia and their partial equity company hold Hangzhou second-hand house transaction market 37 % (Hangzhou real estate economic industry management service platform). This research got about 22000 transaction information, ranging from 2015 to 2019. In order to unify the calculation, this study excluded single-family houses such as bungalows, villas, and townhouses, and only selected collective houses as samples. 2 This study use Amap web API server to geocoding, and the data we also use coordinate transform from GCJ-02 (Chinese local coordinate system) to WGS-84. The telemetry image data was from Landsat 8, and 16 cloudless images with time periods from 2015 to 2019 were selected. Road data comes from an open street map. OSM has complete information on major cities in China and can be of help with this research. Points of interest (POI) come from China's largest POI database company amap (Gao De map), the number of POIs in the study area is about 1 million, including restaurants, shopping centers, parks and other different types of POI. Figure 2. Distribution of price of property transaction. 3 Research method This study uses traditional spatial interpolation and OLS methods combined with remote sensing image to compare. Test and control group introduction: test group add telemetry data to get results to regressing calculation, control group use none RS data to perform regression calculations, one of control group adopt OLS methods, another one adopt spatial interpolation. The research characteristic variables explain 4 variable categories, building structure variables (house area, house age, elevator, bedroom, dining room and living room), distance variables (to the west lake, to city center, to CBD), location variables (bus station, metro station, elementary and secondary schools) and ecological variable (park) Table 1 Characteristic variables proposed to be applied in the model. Characteristic variables Description expected results House age House completion time - House area The size of house area(m2) + Bedroom Numbers of bedroom + 3 Living room and dining Numbers of living room and dining + room room elevator No elevator (0), elevator (1) Distance to city center In this research city center is - Government seat Distance to west lake - Famous Scenic,World Heritage Distance to CBD - Bus station - Metro station - Elementary and + secondary schools park + 4 Expected results The results of the study show that the addition of satellite imagery is more accurate and more time-efficient than the general OLS calculation and geographic interpolation calculations. The biggest factor affecting house prices is not the distance to the West Lake, because the Yuhang and Xiaoshan districts are far from the West Lake and old urban district. The research hopes to provide some reference help for the government and the people facing the housing price issue in Hangzhou, also want to as a reference for combine telemetry images and house price data research. 5 References Danlin Yu and Changshan Wu (2006).Incorporating Remote Sensing Information in Modeling House Values a Regression Tree Approach. Photogrammetric Engineering & Remote Sensing, 72(02), 129–138. Forster, B. (1983). Some urban measurements from Landsat data. Photogrammetric Engineering and Remote Sensing, 49(12), 1693–1707. Fu Yi (2006). A study on spatial variation of Hangzhou housing prices based on hedonic price model. Master's thesis, Zhejiang University, Hangzhou. Jorge E. Patino, Juan C. Duque. (2013). A review of regional science applications of satellite remote sensing in urban settings.Computers, Environment and Urban Systems 37, 1–17. Jensen, R., Gatrell, J., Boulton, J., Harper, B. 2004. Using Remote Sensing and Geographic Information Systems to Study Urban Quality of Life and Urban Forest Amenities. Ecology and Society 9(5): 5. Joe Rossetti (2013).Valuation of Australia’s Green Infrastructure: Hedonic Pricing Model Using the Enhanced Vegetation Index. Master’s thesis, Monash University, Melbourne. 4 Limin Jiao, Gang Xu, Jianfei Jin, Ting Dong, Jiafeng Liu, Yanxi Wu,Boen Zhang (2017).Remotely sensed urban environmental indices and their economic implications. Habitat International67, 22-32. Liu Wenlong, Zhang Xuehua, Huang Changyang (2010). The Influence Factors of Hangzhou's Residential Real Estate Prices. Engineering economy, 5, 54-59. Paul Hindsley & Stuart E. Hamilton & O. Ashton Morgan (2013). Paul Hindsley & Stuart E. Hamilton & O. Ashton Morgan. J Real Estate Finan Econ, 47,489–505. Sofia F. Francoa, Jacob L. Macdonald (2018). Measurement and valuation of urban greenness: Remote sensing and hedonic applications to Lisbon, Portugal. Regional Science and Urban Economics, 72,156-180. Wei Lia, Jean-Daniel M. Saphoresbcd , Thomas W. Gillespie(2015). A comparison of the economic benefits of urban green spaces estimated with NDVI and with high- resolution land cover data. Landscape and Urban Planning, 133,105-117. Wen Hai-Zhen, Zhang Ling, Peng Lu-feng (2010). Spatial Variation of Housing Prices in Hangzhou City: Two-Dimensional Analysis Based on Hedonic Prices. China Land Science, 24(2), 51-56. 毛丰付,罗刚飞,潘加顺(2014)。优质教育资源对杭州学区房价格影响研 究。城市与环境研究 2014 年第 1 卷,第 2 期,53-64。 李畅,汪笛,董婧,江畅(2017)。基于夜间灯光强度的城市住宅平均价格预 测。华中师范大学,专利,武汉。 5.
Details
-
File Typepdf
-
Upload Time-
-
Content LanguagesEnglish
-
Upload UserAnonymous/Not logged-in
-
File Pages5 Page
-
File Size-