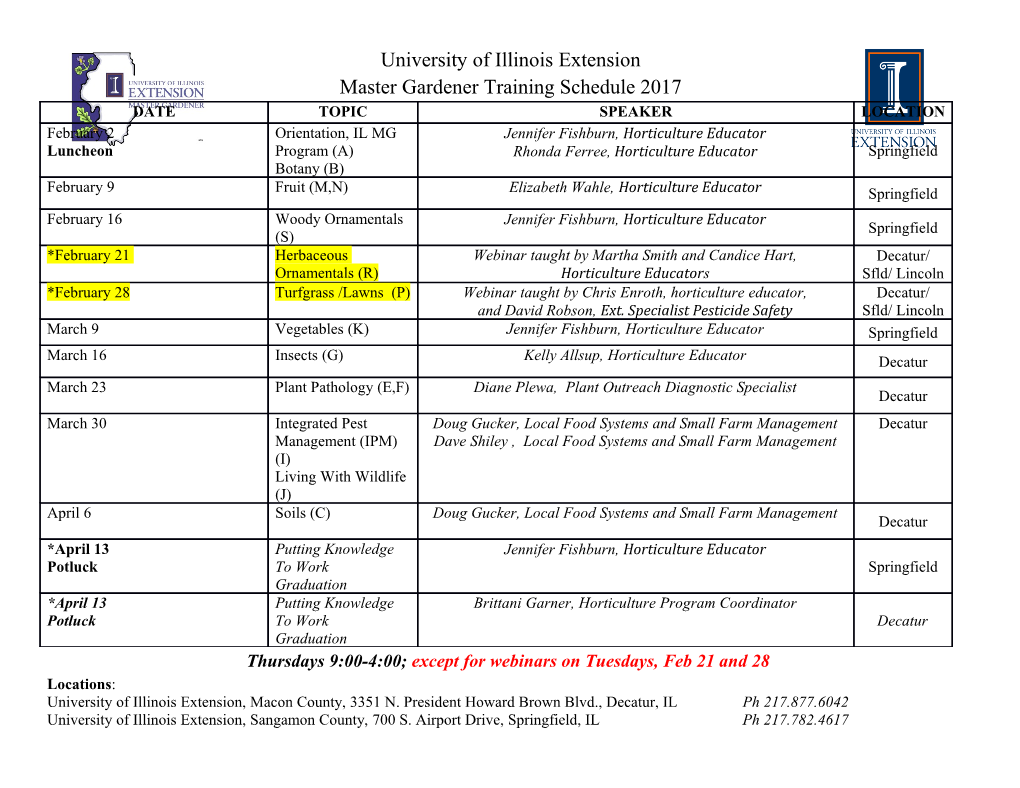
PART 7: INVARIANT MEASURES: ERGODICITY AND EXTREMALITY We discuss the invariant measures in zero-range processes. To determine \all" of them, since the set of invariant measures is a convex set, one tries to prescribe the extreme points of this set. If an invariant measure is known to be an extreme point, the process run under it has interesting ergodic properties. In this subject, many things are known, but there are several open questions. 1. Invariant measures for zero-range processes We say that a probability measure µ on Ω is an invariant measure if Z Z Ptfdµ = fdµ for all bounded Lipschitz functions f 2 L. Let I denote the set of invariant mea- sures. To simplify the discussion, we will assume that p is translation-invariant. How- ever, a rich theory exists when p is not translation-invariant which are remarked upon in the Notes section. Recall the discussion of invariant measures for the zero-range process on a torus d TN . We extend these notions and define marginal ( 1 αk Z(α) g(k)! for k ≥ 1 µ¯α(k) = 1 Z(α) for k = 0 for 0 ≤ α < lim inf g(k). Correspondingly, define Y ν¯α = µ¯α: d x2Z As before ρ = ρ(α) = Eµ¯α [η(0)] is a strictly increasing function of α, and hence the inverse exists. We then define νρ =ν ¯α(ρ) ∗ for ρ < ρ := limα"lim inf g(k) ρ(α). Our first result is that these measures are invariant. ∗ Theorem 1.1. For ρ < ρ , we have νρ 2 I. Moreover, these measures fully charge Ω0, that is 0 νρ(Ω ) = 1: (1.1) This is seen by integrating kηk, X X Eνρ kηk = Eνρ [η(x)]β(x) = ρ β(x) d d x2Z x2Z and noting that X X X p(n)(x; 0) β(x) = = 2 (1.2) 2n d d x2Z x2Z n≥0 1 2 PART 7 given p(n)(x; 0) = p(n)(0; −x) by translation-invariance of p. Hence, kηk < 1 a.s. under νρ. 2. Proof of Theorem 1.1 We first give a generator characterization of invariance of a measure. Proposition 2.1. Let µ be a probability measure on Ω such that Eµ[kηk] < 1. Then, the following are equivalent: (1) R Lfdµ = 0 for all f 2 L (2) µ 2 I. In what follows, we will only use that \(1) implies (2)", and so we will prove this part of the result. Proof. For f 2 L and η 2 Ω0, we have X x;y jLPsf(η)j ≤ a0 η(x)p(x; y) Psf(η ) − Psf(η) d x;y2Z 4a0s ≤ 3a0c(f)e kηk: 4a0s The inequalities use that the Lipschitz bound on g, and that c(Psf) ≤ 3e c(f) from Theorem 2.4 in Part 6. When (1) holds, for f 2 L which is bounded, Z h i Z h Z t i Ptf − f dµ = LPsfds dµ. 0 Since Psf 2 L, and the bound given above, we may interchange the integrals by Fubini's theorem. Also, by (1), we have therefore Z h Z t i Z t h Z i LPsfds dµ = LPsfdµ ds = 0 0 0 and so may conclude (2). Exercise 2.2. To prove the converse, \(2) implies (1)", we refer the reader to [1][Lemma 2.9]. Lemma 2.3 (Finite set invariance). Suppose that p is a transition probability on A = fx : jx j ≤ n; 1 ≤ i ≤ dg which is doubly stochastic: P p(x; y) = 1 for n i x2An all y 2 An. Then, νρ is invariant for the zero-range process on An. Proof. We need only show that Eνρ [Lf(η)] = 0 for all functions f. Recall X Lf(η) = g(η(x))p(x; y)f(ηx;y) − f(η): x;y2An Since x;y Eνρ g(η(x))f(η ) = α(ρ)Eνρ f(η + δy) = Eνρ g(η(y))f(η) ; we have that X x;y X Eνρ g(η(x))p(x; y)f(η ) = p(x; y)Eνρ g(η(y))f(η) x;y2An x;y2An X = Eνρ g(η(y))f(η) : y2An PART 7 3 On the other hand, clearly, X X p(x; y)Eνρ g(η(x))f(η) = Eνρ g(η(x))f(η) : x;y2An x2An Hence, combining these estimates, Eνρ [Lf] = 0. Proof of Theorem 1.1. For a transition probability p on Zd, define the `trunca- tion' (different than in the previous Part 6), 8 1 if x = y 62 A < n p (x; y) = p(x; y) + Q−1 P p(x; z) P p(z; y) if x; y 2 A n n z62An z62An n : 0 otherwise where X X X X Qn = p(z; y) = 1 − p(z; y) = p(z; y): z2An;y62An z2An z;y2An y2An;z62An P d We have that (A) pn is a transition probability: y pn(x; y) = 1 for all x 2 Z . If x 62 A , it is trivial. If x 2 A , noting that Q = P p(z; y), we have n n n z62An;y2An P p (x; y) = P p (x; y) = 1. Moreover, p has no transitions from A to its y n y2An n n n complement. P d In addition, (B) x pn(x; y) = 1 for all y 2 Z : If y 62 An, the claim is trivial. If y 2 An, write X X −1 X X X pn(x; y) = p(x; y) + Qn p(x; z) p(z; y) = p(x; y): x x2An x2An;z62An z62An x The last quantity equals 1 if p is doubly-stochastic which is the case if p is translation- invariant. d Also, (C) pn ! p for all x; y 2 Z . Eventually, x; y 2 An. The claim follows as Q−1 P p(x; y) ≤ 1. n z62An From Lemma 2.3, as nothing moves off An, we have that Eν [Lnf] = 0 for f 2 L where Ln is the generator for the zero-range process on An according to transition probability pn. Therefore, to show Eνρ [Lf] = 0, it is enough to show Eνρ jLnf − Lfj ! 0: It is straightforward to write X X Eνρ jLnf − Lfj ≤ a0c(f)Eνρ η(x) jp(x; y) − pn(x; y)j β(x) + β(y) d x2Z y6=x = I1 + I2 corresponding to the two factors β(x) and β(y). We bound I1 by dominated convergence. We evaluate I1 as X X ρ β(x) jp(x; y) − pn(x; y)j: x y6=x For fixed x, eventually x 2 An for large n, and so X X X X jp(x; y) − pn(x; y)j ≤ jp(x; y) − pn(x; y)j + p(x; y) = 2 p(x; y) y y2An y62An y62An P using the form of Qn. Hence, since x β(x) < 1 and the last display vanishes as n " 1 for each x, by dominated convergence I1 vanishes as n " 1. 4 PART 7 We now bound I2 in a similar manner. Write I2 as X X X X ρ β(y)jp(x; y) − pn(x; y)j = ρ β(y) jp(x; y) − pn(x; y)j: x y6=x y x6=y For each y eventually y 2 An. Then, using a form of Qn, we have that X X jp(x; y) − pn(x; y)j ≤ 2 p(x; y) x6=y x62An P which vanishes as p is doubly-stochastic. Hence, as y β(y) < 1, I2 vanishes by dominated convergence. 3. Extremality, Harmonicity and Ergodicity First, what does it mean to be extremal? Let Σ be a space with Borel sets B. Let Q be an invariant proability measure on Σ for the Markov process η(t). Let PQ, as before, be the probability on the path space with initial distribution Q. Let Tt be the process semigroup. Definition 3.1. We say Q is an extremal invariant measure if the the following property holds. When for 0 < < 1 and invariant probability measures Q1 and Q2 we have Q = Q1 + (1 − )Q2, then Q = Q1 = Q2. We note if the process has only one invariant measure Q, then of course it is extremal. The import of the definition comes when the process is reducible in some way. For instance, in finite state Markov chains, with two irreducible components C1 and C2, the extreme invariant measures are exactly the unique invariant measures supported on C1 and C2 respectively. One might ask why is it useful to know when an invariant measure is extremal. It turns out there is an interesting connection with harmonic functions and shift- ergodicity. Lemma 3.2. The space L2(Q) can be decomposed into the direct sum of 2 c 2 H0 = fg 2 L (Q): Ttg = g; t ≥ 0g; and H0 = fTth − h : h 2 L (Q); t ≥ 0g: c Proof. Let f be perpendicular to all functions in H0. Then, hf; Tth − hi = 0 for all ∗ h and t ≥ 0. This means Tt f = f or Ttf = f for all t ≥ 0. Hence, f 2 H0. 2 Since Tt is a contraction on L (Q), we have the following ergodic theorem due to Von Neumann. Proposition 3.3. We have for f 2 L2(Q) that Z t 1 ^ Tsf ds ! f t 0 2 ^ 2 converges in L (Q) to f 2 L (Q) which is a projection of f onto the subspace H0.
Details
-
File Typepdf
-
Upload Time-
-
Content LanguagesEnglish
-
Upload UserAnonymous/Not logged-in
-
File Pages8 Page
-
File Size-