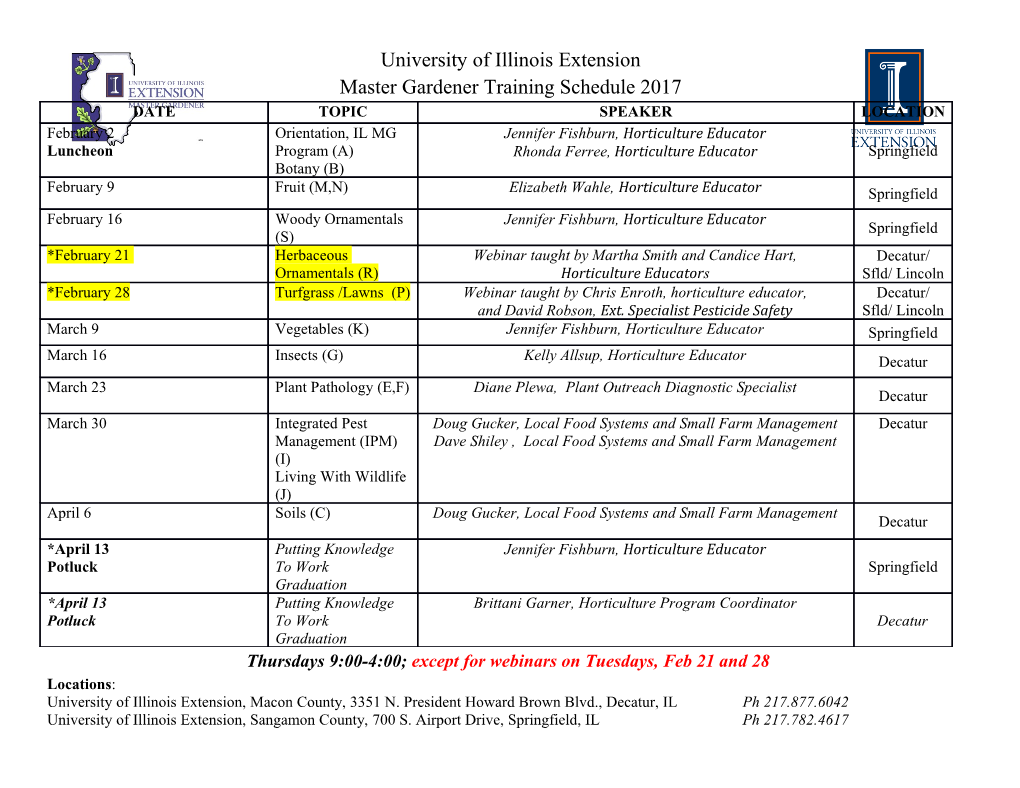
UNIVERSIDAD POLITÉCNICA DE MADRID Facultad de Ciencias de la Actividad Física y del Deporte (INEF) Modelling and Simulation in Game Performances of Basketball Players and Teams in the National Basketball Association Tesis Doctoral D. Shaoliang Zhang 张绍良 Madrid, 2019 UNIVERSIDAD POLITÉCNICA DE MADRID Facultad de Ciencias de la Actividad Física y del Deporte (INEF) MODELLING AND SIMULATION IN GAME PERFORMANCES OF BASKETBALL PLAYERS AND TEAMS IN THE NATIONAL BASKETBALL ASSOCIATION TESIS DOCTORAL D. Shaoliang Zhang Madrid, 2019 DEPARTAMENTO DE CIENCIAS DE LA ACTIVIDAD FÍSICA, DEL DEPORTE Y DEL OCIO Facultad de Ciencias de la Actividad Física y del Deporte (INEF) MODELLING AND SIMULATION IN GAME PERFORMANCES OF BASKETBALL PLAYERS AND TEAMS IN THE NATIONAL BASKETBALL ASSOCIATION AUTOR: D. Shaoliang Zhang DIRECTOR: Dr. Alberto Lorenzo Calvo, Professor en Ciencias de la Actividad Física y del Deporte, Universidad Politécnica de Madrid. Dr. Miguel Ángel Gómez Ruano, Doctor en Ciencias de la Actividad Física y del Deporte, Universidad Politécnica de Madrid. Madrid, 2019 Tribunal nombrado por el Magfco. y Excemo. Sr. Rector de la Universidad Politécnica de Madrid, el día …..de………………………………………de 2019. Presidente: D…………………………………………………………………………… Vocal: D………………………………………………………………………………... Vocal: D………………………………………………………………………………... Vocal: D………………………………………………………………………………... Secretario: D……………………………………………………………………………. Realizando el acto de defensa y lectura de la Tesis el día…….de…………………… de 2019. En……………………………………………………… Calificación…………………………………….…….... EL PRESIDENTE LOS VOCALES EL SECRETARIO “TRAVEL AND CHANGE OF PLACE IMPART NEW VIGOR TO THE M I N D . ” – S E N E C A Abstract The National Basketball Association (NBA) is commonly regarded as the most popular and competitive basketball league in the world. Basketball players and teams respond to the stress of practice and competition, there remains a clear need to use updated sports performance models to inform starting points for player preparation. One of the most common methods of monitoring sports performance is using game-related statistics to evaluate technical and tactical behaviours, as well as the efficiency of players and teams throughout the season. The application of new training and competing interventions from statistical models can optimise training process and enhance technical-tactical and physical development. Purpose: The general aim of the thesis is to model and simulate the game performances of basketball players and teams based on game-related statistics integrating with influencing factors in the National Basketball Association. To achieve the aims of this research, this thesis can be summarized in the five chapters and separated into the following three sections. Firstly, the aim of the first section was to explore the (dis)similarity of game-play characteristics throughout in-season period in the National Basketball Association (Chapter 2). Secondly, the aim of the second section was to group basketball players into similar clusters based on a combination of anthropometric characteristics and playing experience (Chapter 3) or different levels of scoring production (Chapter 4); and then explore the distribution of basketball players from different levels of teams within the obtained clusters. Finally, the aim of the third section was to examine the independent and interactive influences of situational variables on technical and physical performances according to playing position (Chapter 5 and 6). Methods: Thirteen performance-related indicators of all the 1230 games of regular season I 2016–2017 in the National Basketball Association were analysed by Non-metric multidimensional scaling (Chapter 2). Twenty performance-related indicators from 699 balanced games of 2015-2016 regular season were analysed using a two-step cluster model and a discriminant analysis (Chapter 3). Thirteen performance-related indicators of all the 1230 games of regular season 2015–2016 were analysed by using a two-step cluster model, a discriminant analysis, and K-nearest neighbour analysis (Chapter 4). Seventeen performance- related indicators of all the 699 matches of regular season 2015–2016 were performed using magnitude-based inferences (Chapter 5). Twenty performance-related indicators of all the 692 balanced games of regular season 2016–2017 in the National Basketball Association were conducted by decision tree algorithm (exhaustive CHAID) and magnitude-based inferences (Chapter 6). Study 1: The two-dimensional multivariate matrix showed multivariate team profiles generally presented similarity while the beginning and ending of the season (October and April) showed relatively dissimilarity. Although each team presents unique paths throughout in-season period, the dominant teams in the NBA may uncover the similar game styles. In addition, the game-play of the teams evolves into effective interactions in terms of offence and defence as the competition progresses whilst presenting an increased trend in the number of three-point field goals throughout in-season period. Study 2: The clustering process allowed identifying five different player profiles: Top height and weight (HW) with low experience, TopHW-LowE; Middle HW with middle experience, MiddleHW-MiddleE; Middle HW with top experience, MiddleHW-TopE; Low HW with low experience, LowHW-LowE; Low HW with middle experience, LowHW-MiddleE. Discriminant analysis showed that TopHW-LowE group was highlighted by two-point field II goals made and missed, offensive and defensive rebounds, blocks, and personal fouls; whereas the LowHW-LowE group made fewest passes and touches. The players from weak teams were mostly distributed in LowHW-LowE group, whereas players from strong teams were mainly grouped in LowHW-MiddleE group; and players that participated in the finals were allocated in the MiddleHW-MiddleE group. Study 3: In the first step, the obtained results provided an automatically determined solution with two clusters, with a good silhouette measure of cohesion and separation (average silhouette=0.7). A new categorical variable was saved in the database with these classification results. For designation purposes, one of the clusters was labelled as “lower-scoring players” and gathered 258 players (53%) averaging 0.300.06 points per minute (range 0-0.38) and the other cluster was labelled as “higher-scoring players”, gathering 230 players (47%) that averaged 0.490.09 points per minute (range 0.39-0.85). In the second step, the obtained discriminant functions were all significant (p0.001) with chi-square values of 119.7 for guards, 105.9 for forwards and 42.8 for centres. There were variables achieving high discriminant status for all positions, such as the free-throws and turnovers, and more moderate variables, such as the assists and the defensive rebounds. Interestingly, distance covered and running speed were the only variables with no substantial discriminant status. In addition, the guards’ discriminant variables were related to field-goals (two and three-point), the forwards to free throws, and the centres to three-point field goals, touches and steals. Study 4: As could be expected, results showed that players’ technical and physical performances differed between strong and weak teams. In technical aspect, forwards and centres from strong teams made more three point field goals, but fewer two-point field goals, than their counterparts from weak teams. Interestingly, forwards and guards from strong III teams covered shorter distances and lower speeds than their peers from weak teams. In addition, the three point field goals presented high variability compared with other performance indicators. Game location generally had no significant impact on the players’ performance. Guards exhibited relatively lower variability in technical and physical variables in comparison with forwards and centres. Study 5: Our study indicated that defensive rebounds, blocked shots, and assists determined between winning and losing games for strong teams while defensive rebounds and turnovers were the key performance indicators for weak teams. Subsequently, in strong vs strong games, players from winning teams in home games ran slower than their peers from losing teams, whereas an opposite trend was found in away games. In strong vs weak games, players from winning teams in home games covered more distance and ran faster than their peers from losing teams. In weak vs weak games, effective defence played an important role in winning the games. Conclusions: A similarity of team profiles was presented in the middle of the regular season while a relative dissimilarity was discovered at the beginning and end of the season. Besides, defensive rebounds were the common KPIs that discriminated between winning and losing games for strong and weak teams in the current NBA. Further, three point field goals made and assists showed increased trend as the competition progressed whilst LowHW-MiddleE players, high-scoring guards, and forwards and centres from strong teams have better performance in three point field goals. Importantly, game performance was influenced by the situational variables, either independently or interactively. Hence, this thesis emphasized the need to consider the potential interactive effects of situational variables during the assessment of tactical, technical and physical performances in the future research. IV Declaration I, Shaoliang Zhang, declare that the Doctor of Philosophy thesis titled “Modelling and simulation in game performances of
Details
-
File Typepdf
-
Upload Time-
-
Content LanguagesEnglish
-
Upload UserAnonymous/Not logged-in
-
File Pages213 Page
-
File Size-