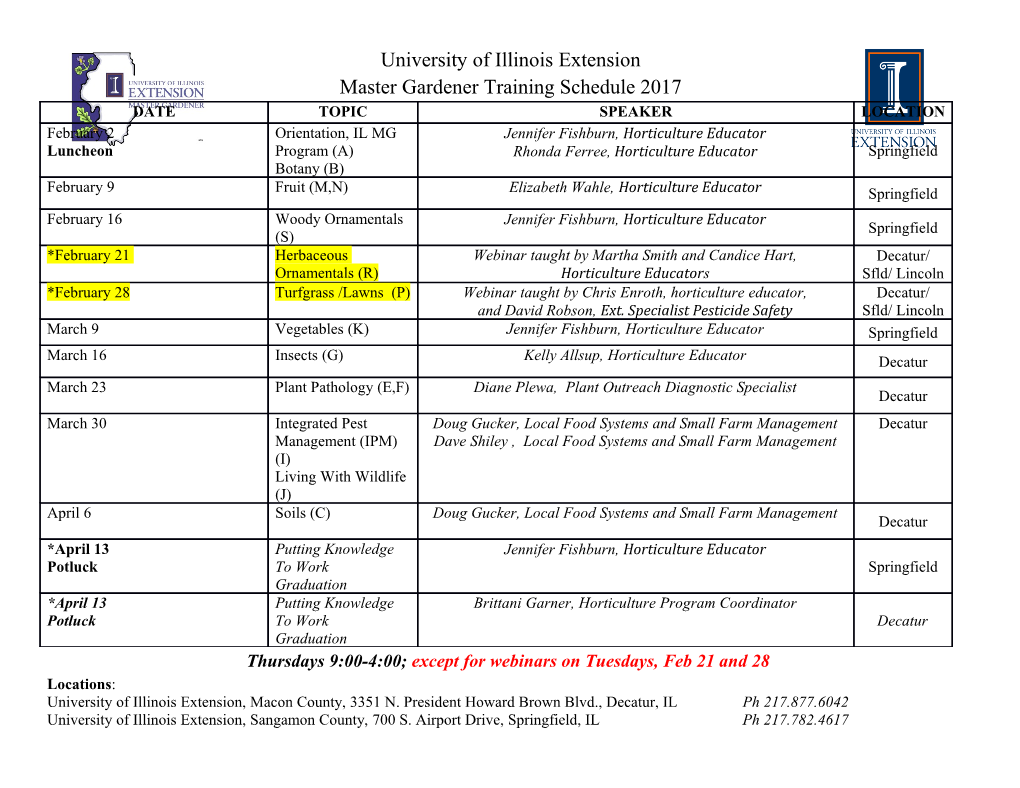
Technical Report Documentation Page 1. Report No. 2. Government Accession No. 3. Recipient's Catalog No. SWUTC/12/476660-00079-1 4. Title and Subtitle 5. Report Date FREE-FLOATING CARSHARING SYSTEMS: August 2012 INNOVATIONS IN MEMBERSHIP PREDICTION, MODE 6. Performing Organization Code SHARE, AND VEHICLE ALLOCATION OPTIMIZATION METHODOLOGIES 7. Author(s) 8. Performing Organization Report No. Katherine Kortum, Randy Machemehl 476660-00079-1 9. Performing Organization Name and Address 10. Work Unit No. (TRAIS) Center for Transportation Research University of Texas at Austin 11. Contract or Grant No. 1616 Guadalupe Street, Suite 4.200 DTRT07-G-0006 Austin, Texas 78701 12. Sponsoring Agency Name and Address 13. Type of Report and Period Covered Southwest Region University Transportation Center Texas A&M Transportation Institute Texas A&M University System 14. Sponsoring Agency Code College Station, Texas 77843-3135 15. Supplementary Notes Supported by revenues from the US Department of Transportation. 16. Abstract Free-floating carsharing systems are among the newest types of carsharing programs. They allow one-way rentals and have no set “homes” or docks for the carsharing vehicles; instead, users are permitted to drive the vehicles anywhere within the operating zone and leave the vehicle in a legal parking space. Compared to traditional carsharing operations, free-floating carsharing allows much greater spontaneity and flexibility for the user. However, it leads to additional operational challenges for the program. This report provides methodologies for some of these challenges facing both free-floating and traditional carsharing programs. First, it analyzes cities with carsharing to determine what characteristics increase the likelihood of the city supporting a successful carsharing program; high overall population, small household sizes, high transit use, and high levels of government employment all make the city a likely carsharing contender. Second, in terms of membership prediction, several modeling alternatives exist. All of the options find that the operating area is of key importance, with other factors (including household size, household densities, and proportion of the population between ages 20 and 39) of varying importance depending on the modeling technique. Third, carsharing trip frequencies and mode share are of value to both carsharing and metropolitan planning organizations, and this report provides innovative techniques to determine the number of trips taken and the share of total travel completed with carsharing (both free-floating and traditional). Fourth and finally, an original methodology for optimizing the vehicle allocation issue for free-floating carsharing organizations is provided. The methodology takes a user input for the total number of vehicles and returns the allocations across multiple demand periods that will maximize revenue, taking into account the cost of reallocating vehicles between demand periods. 17. Key Words 18. Distribution Statement Carsharing; Cars2Go; Binary Logit No restrictions. This document is available to the public through Metropolitan Modeling; Mode Share NTIS: Modeling National Technical Information Service 5285 Port Royal Road Springfield, Virginia 22161 19. Security Classif.(of this report) 20. Security Classif.(of this page) 21. No. of Pages 22. Price Unclassified Unclassified 123 Form DOT F 1700.7 (8-72) Reproduction of completed page authorized FREE-FLOATING CARSHARING SYSTEMS: INNOVATIONS IN MEMBERSHIP PREDICTION, MODE SHARE, AND VEHICLE ALLOCATION OPTIMIZATION METHODOLOGIES By Katherine Kortum Graduate Research Assistant The University of Texas at Austin and Randy Machemehl, P.E. Professor The University of Texas at Austin Report SWUTC/12/476660-00079-1 Project 476660-00079 Performed in cooperation with the Southwest Region University Transportation Center Center for Transportation Research The University of Texas at Austin 1616 Guadalupe Street, Suite 4.200 Austin, Texas 78701 August 2012 iv ABSTRACT Free-floating carsharing systems are among the newest types of carsharing programs. They allow one-way rentals and have no set “homes” or docks for the carsharing vehicles; instead, users are permitted to drive the vehicles anywhere within the operating zone and leave the vehicle in a legal parking space. Compared to traditional carsharing operations, which require the user to bring the vehicle back to its assigned parking space before being able to end the rental, free-floating carsharing allows much greater spontaneity and flexibility for the user. However, it leads to additional operational challenges for the program. This report provides methodologies for some of these challenges facing both free-floating and traditional carsharing programs. First, it analyzes cities with carsharing to determine what characteristics increase the likelihood of the city supporting a successful carsharing program; high overall population, small household sizes, high transit use, and high levels of government employment all make the city a likely carsharing contender. Second, in terms of membership prediction, several modeling alternatives exist. All of the options find that the operating area is of key importance, with other factors (including household size, household densities, and proportion of the population between ages 20 and 39) of varying importance depending on the modeling technique. Third, carsharing trip frequencies and mode share are of value to both carsharing and metropolitan planning organizations, and this report provides innovative techniques to determine the number of trips taken and the share of total travel completed with carsharing (both free-floating and traditional). Fourth and finally, an original methodology for optimizing the vehicle allocation issue for free-floating carsharing organizations is provided. The methodology takes a user input for the total number of vehicles and returns the allocations across multiple demand periods that will maximize revenue, taking into account the cost of reallocating vehicles between demand periods. v vi EXECUTIVE SUMMARY Carsharing, the rental of a vehicle by the minute or the hour, is a concept that is decades old, but it has become much more common in recent years. At the end of 2009, the carsharing organization Car2Go, run by Daimler Auto Group, began operating in Austin. Using data provided by Car2Go about its members and its rentals, this report has created new methodologies to understand the membership and usage of this new style of free-floating carsharing system. Exploratory analysis of the data shows that Car2Go members are concentrated in the central part of the city, an entirely expected finding. More interestingly, in the zip code corresponding to downtown Austin, one in six residents is currently a Car2Go member. High proportions of members can also be found in the neighborhoods around the university and just outside of downtown. Members tend to join at much higher rates when Car2Go puts on a promotional activity, with significant spikes in membership occurring when the program opened to the public and when the free membership period was about to expire. As the membership has grown more diverse than the initial City of Austin employees who made up the pilot test group, the vehicles have been used during larger parts of both the day and the week; weekend and after-work rentals were nearly non-existent in the program’s initial months, but now carsharing is just as likely to occur on Saturday as Tuesday and it also occurs at all hours of the day and night. As members have grown more comfortable with the service, they have also become more efficient in their use of the service, taking advantage of the one-way option. A study of metropolitan areas examines objective characteristics that make a city likely to have a successful carsharing program. Unsurprisingly, population is a key factor, as larger cities are more likely to have carsharing services than are small cities. Smaller average household sizes and larger fractions of the population commuting via transit also make a city more amenable to carsharing. Most interestingly, increasing numbers of government workers in a metropolitan area also increase the likelihood of carsharing succeeding; this may be connected to the many governmental agencies contracting with carsharing services to reduce their own fleet sizes and the associated costs of owning the vehicles. Ethnicity, median age, and household income, all of which have appeared as significant variables in previous carsharing literature, did not prove to be statistically significant in this analysis. Membership prediction is of great importance to carsharing organizations as they plan their operational characteristics. Several alternatives to this prediction are possible, including two-step models and a one-step model. In the two-step version, binary logit modeling determines which census blocks are likely to contain any members, and either linear regression or logit modeling estimates the proportion of those blocks that are members. The preferred alternative, however is a one-step Heckman sample selection model, which correlates the errors in the two dependent variables (binary membership and continuous membership proportions). The Heckman sample selection model shows that the geofence location is a vital factor in determining membership, as are household size, fraction of commuters using transit, age, race, and proportion of 20-39 year olds living in an area. vii Mode share modeling is another innovative
Details
-
File Typepdf
-
Upload Time-
-
Content LanguagesEnglish
-
Upload UserAnonymous/Not logged-in
-
File Pages123 Page
-
File Size-