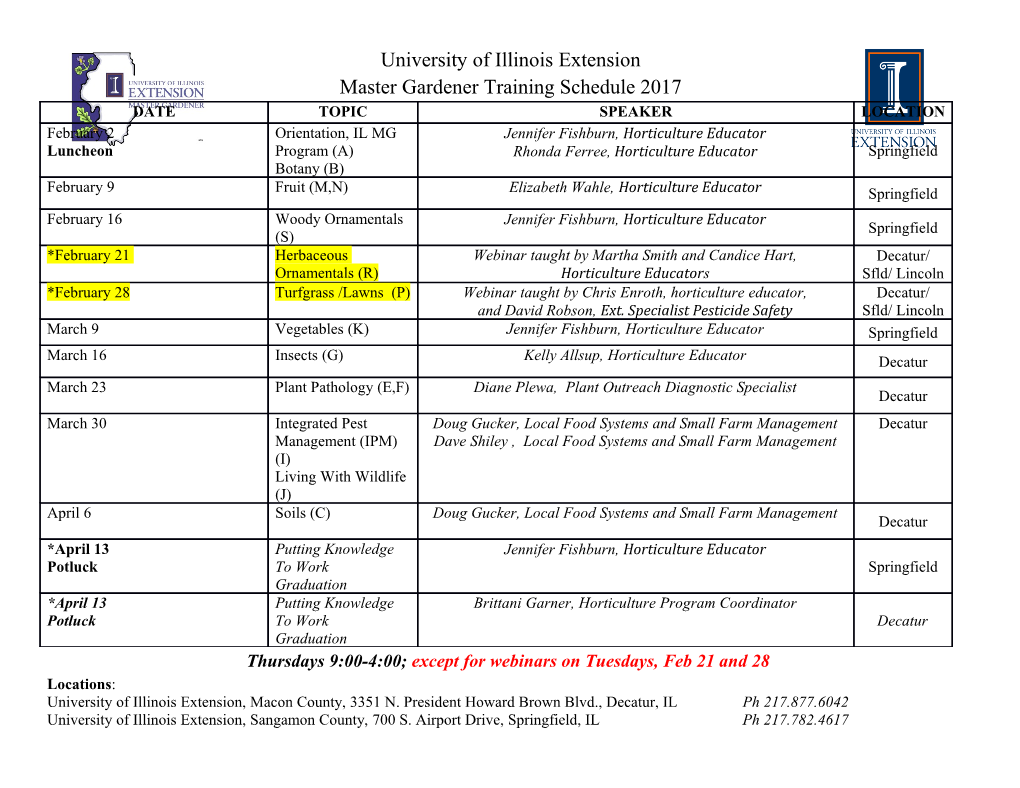
EGS-AGU-EUG Joint Assembly, Nice, France, 6 - 11 April 2003 Session: Hydrological Sciences HS12 Rainfall modelling: scaling and non-scaling approaches AA CASECASE STUDYSTUDY OFOF SPATIALSPATIAL--TEMPORALTEMPORAL RAINFALLRAINFALL DISAGGREGATIONDISAGGREGATION ATAT THETHE TIBERTIBER RIVER,RIVER, ITALYITALY Paola Fytilas1 , Demetris Koutsoyiannis2, Francesco Napolitano1 1 Department of Hydraulics, University of Rome “La Sapienza”, 2 Department of Water Resources, National Technical University of Athens with hourly data used Aniene River Catchment-Tiber River-Central Italy Anie ne F. F. A ni ene io r iv b m S i . Raingauges T • F. Aniene :January 1994-Decemberinin thethe generationgeneration phasephase 1999 F. Ani ene Raingauges with hourly data used to F. Aniene • evaluate the effectiveness of the methodology Raingauges with daily data only StudyStudy Area:Area • DataData periodperiod 1.TIVOLI 2.L UNGHEZZA 8.ROMA ACQUA ACETOSA 7.PANTANO BORGHESE 3. FRASCATI 5.ROMA FLAMINIO 4.PONTE SALARIO 6.ROMA MACAO TheThe MethodologyMethodology Observed DAILY data at Observed several points HOURLY data at several points Marginal statistics (d) Marginal statistics (h) Temporal Correlation(d) Temporal Correlation(h) Spatial Correlation (d) Spatial Correlation (h) Spatial-temporal Multivariate Simplified COUPLING Rainfall model or Point rainfall model TRANSFORMATION empirical expression AR(1) disaggregation for cross-correlation coefficients Synthetic Hourly Synthetic Hourly Data at several Data at several points points (not consistent (consistent with daily with daily amounts) amounts) ParameterParameter EstimationEstimation Essential statistics to preserve in the generated hourly series : 1.the means, variances and coefficients of skewness; 2.the temporal correlation structure (autocorrelations); 3.the spatial correlation structure (lag zero cross-correlations); and 4.the proportions of dry intervals. Daily time scale:estimated directly using the data set available for all raingauges Hourly time scale:All the statistics, including the cross-correlations coefficients between gages 1,2,3 can be estimated directly from the data set available at these locations. The unknown cross-correlation coefficients at hourly level were estimated indirectly using the empirical relationship: m (rij)h = (rij)d PreservationPreservation ofof marginalmarginal statisticsstatistics Mean Proportion dry Standard deviation 0.16 0.92 0.76 historical 0.74 0.16 value used on disaggregation 0.90 0.15 synthetic 0.88 0.72 0.15 0.86 0.70 0.14 0.84 0.68 0.66 Mean 0.14 0.82 0.13 0.64 Proportion dry 0.80 0.13 0.62 0.78 Standard deviation 0.12 0.76 0.60 0.12 12345678 0.58 12345678 12345678 raingauges raingauges raingauges Skewness Maximum hourly rainfall depths Lag1 autocorrelation coefficients 0.70 14.00 25 0.60 12.00 20 0.50 10.00 15 0.40 8.00 0.30 c 6.00 10 Skewness 0.20 4.00 valueMaximum 5 Lag1 autocorrelation 0.10 2.00 0 0.00 0.00 12345678 12345678 12345678 raingauges raingauges raingauges PreservationPreservation ofof crosscross correlationcorrelation coefficientscoefficients Lag0 cross-correlation coefficients-gauge 1 Lag0 cross-correlation coefficients-gauge 2 Lag0 cross-correlation coefficients-gauge 3 1.00 1.00 1.00 0.90 0.90 0.90 0.80 0.80 0.80 0.70 0.70 0.70 0.60 0.60 0.60 0.50 0.50 0.50 0.40 0.40 0.40 0.30 0.30 0.30 cross-correlations 0.20 cross-correlations 0.20 cross-correlations 0.20 0.10 0.10 0.10 0.00 0.00 0.00 12345678 12345678 12345678 raingages raingages raingauges Lag0 cross-correlation coefficients-gauge 4 Lag0 cross-correlation coefficients-gauge 5 Lag0 cross-correlation coefficients-gauge 6 1.00 1.00 1.00 0.90 0.90 0.90 0.80 0.80 0.80 0.70 0.70 0.70 0.60 0.60 0.60 0.50 0.50 0.50 0.40 0.40 0.40 0.30 0.30 0.30 cross-correlations 0.20 cross-correlations 0.20 cross-correlations 0.20 0.10 0.10 0.10 0.00 0.00 0.00 12345678 12345678 12345678 raingauges raingauges raingauges PreservationPreservation ofof autocorrelationautocorrelation coefficientscoefficients Ponte Salario Roma Flaminio 1.00 1.00 0.90 0.90 H5 S5 Markov 0.80 H4 S4 Markov 0.80 0.70 0.70 0.60 0.60 0.50 0.50 0.40 0.40 0.30 0.30 Autocorrelation Autocorrelation 0.20 0.20 0.10 0.10 0.00 0.00 012345678910 012345678910 Lag Lag Roma Macao 1.00 0.90 0.80 H6 S6 Markov 0.70 0.60 0.50 0.40 Autocorrelation 0.30 0.20 0.10 0.00 012345678910 Lag PreservationPreservation ofof probabilityprobability distributiondistribution functionsfunctions atat gaugegauge PontePonte SalarioSalario Hourly rainfall depths 10 Historical Simulated Length of dry intervals 1 250 200 hourly rainfall depth [mm/h] Historical 0.1 Simulated 0.7 0.8 0.9 0.95 0.99 0.999 0.9999 150 Non exceedence probability 100 50 length of dry intervals [h] intervals dry of length 0 0.001 0.01 0.1 1 Exceedence probability PreservationPreservation ofof historicalhistorical hyetographshyetographs Ponte Salario Roma Flaminio 6 6 5 5 H4 H5 4 S4 4 S5 3 3 2 2 1 1 hourly rainfall depths [mm] hourly rainfall depths [mm] depths rainfall hourly 0 0 3/12/1998 3/12/1998 4/12/1998 4/12/1998 5/12/1998 5/12/1998 3/12/1998 3/12/1998 4/12/1998 4/12/1998 5/12/1998 5/12/1998 0:00 12:00 0:00 12:00 0:00 12:00 0:00 12:00 0:00 12:00 0:00 12:00 Roma Macao 6 5 H6 4 S6 3 2 1 hourly rainfall depths[mm] 0 3/12/1998 3/12/1998 4/12/1998 4/12/1998 5/12/1998 5/12/1998 0:00 12:00 0:00 12:00 0:00 12:00.
Details
-
File Typepdf
-
Upload Time-
-
Content LanguagesEnglish
-
Upload UserAnonymous/Not logged-in
-
File Pages9 Page
-
File Size-