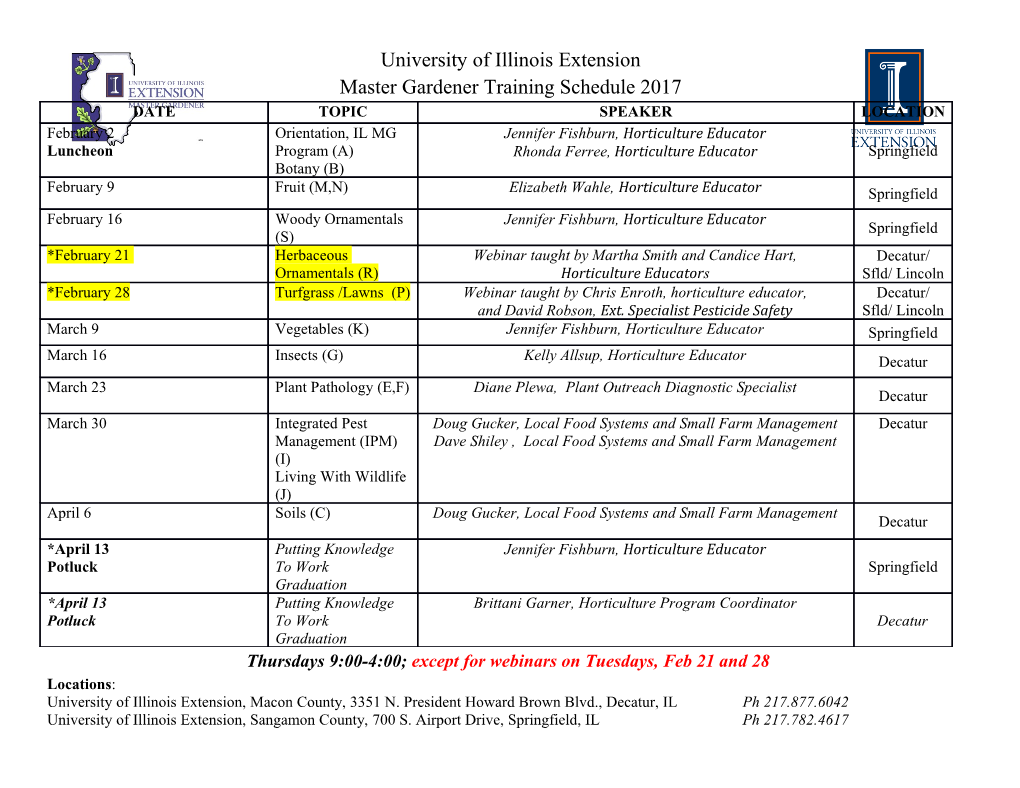
Clinical Trial Laboratory Data Management using the SAS® System Marianne Hack, Covance, Inc., Princeton NJ ABSTRACT In terms of volume, for many clinical trials laboratory process lab data, these standard agreements are data comprises a very large portion of all data collected. adhered to whenever possible on any studies that Add to that the fact that laboratory data is inherently utilize these laboratories. complex, and the management of the data acquisition (electronic and/or data entry), cleaning (synchronization Given that we know what to expect when receiving data of databases), and reporting (unit conversions, files from a particular laboratory data vendor, we can character to numeric conversions, result flagging, etc.) then build standard SAS modules to import and processes can be overwhelming. This paper discusses reformat the vendor data. The end result is that a SAS an approach to building and using an arsenal of data set (see example B) containing data from Vendor specialized SAS program modules in order to A will be very similar to a SAS data set containing data standardize the various processes involved in from Vendor B. These SAS data sets are then ready managing and reporting clinical laboratory data. for the data cleaning stage. DATA CLEANING INTRODUCTION Data review and cleaning of vendor data requires As a CRO (Contract Research Organization), we are additional steps over and above what one might required to be able to work with data from a multitude of ordinarily do to review data-entered laboratory data. sources. Clinical laboratory data is usually integrated Commonly called ‘header cleaning’, this involves into the clinical database via direct data entry or reconciling the patient identifiers from the vendor through importation of electronic data files. Lab data database with the clinical database to ensure rarely arrives ‘analysis-ready’, usually requiring consistency and completeness of data. reformatting, data cleaning, conversions, or flagging. To streamline the processing effort prior to analysis, our How do we accomplish this? The missing link is a table approach has been to reformat the laboratory data as that we create in the clinical database. This linking much as possible into standard data set structures, table is data-entered from the hardcopy lab report that which then allow for usage of standard SAS data review has been sent from the laboratory to the investigator’s and reporting modules. site. This hardcopy report contains all of the test results for a particular patient’s lab sample, and arrives as part of the patient’s Case Report Form (CRF). Selected DATA ACQUISITION (MAKING SENSE OUT OF identifying information from the report is then data- GOBS OF DISSIMILAR DATA) entered along with the other CRF pages. Many clinical trials utilize a ‘Central Laboratory’ for sample analysis. All investigators ship the test samples The data vendor’s own unique sample identifier field is to the same laboratory. The advantages to using a used as the pivot point from which to compare the central laboratory are consistent testing methodology, vendor’s patient identifiers with the clinical database equipment calibration, and result reporting methods as patient identifiers. We have built standard SAS well as consistent data structures on the back end. modules that perform this comparison based on the Data from central laboratories is usually electronically expected data set structures. imported into the clinical database. Some clinical trials may utilize the ‘local’ laboratory DATA DICTIONARY MAPPING scheme in which investigators take the test samples to Most laboratories utilize an in-house coding technique a laboratory in their local area. Data from these to identify each specific laboratory test; each laboratory laboratories may be electronically imported if the has its own method. For example, Vendor A may laboratory is sophisticated enough, or may be data- record hemoglobin as ‘HGB’, whereas Vendor B may entered directly into the database. record it as ‘R3701’. To allow for standard reporting modules, our approach has been to utilize our own in- Trials may also use a combination of central and local house dictionaries. This requires us to set up a laboratories. The challenge is to assimilate the data mapping from the laboratory’s test identifiers to our in- into one coherent stream for cleaning and reporting house test identifiers. This is also done for units of purposes. measurement. Having a standardized set of test and unit identifiers MANAGEMENT OF THE DATA VENDORS facilitates the building of standard data manipulation The first step is to manage the lab data vendors. This and reporting tools. is critical to the development of processes to be described below. We have set up data-transmission agreements with several clinical laboratories that PREPARING DATA FOR ANALYSIS outline a standard data file format and contents (see Creating reports and statistical summaries of laboratory example A). To maximize our ability to efficiently test results is probably the most complex part of dealing with lab data. On any given study there may be comments on certain laboratory test results. These approximately 25 to 30 different tests performed for comments are then collected with the CRF and data- each patient at each study visit where labs are required. entered into the database. It is then necessary to This results in a large amount of data to be match these comments up with the appropriate result summarized. Tests are usually grouped into three main data from the lab. categories (Hematology, Blood Chemistry, and Urinalysis); other specialized groups may be required. Each test category, as well as different tests within REFERENCE RANGE FLAGGING each category, may have its own data handling rules. Each laboratory has its own reference range of what is considered normal values for each test. Any test result Chemistry test results are the easiest to manage. The above the high limit of the range is flagged as ‘High’ results are usually reported in numeric units. Many of and any result below the low limit of the range is the conventional units (i.e., used in the USA) follow the flagged as ‘Low’. Reference ranges are generally set Standard International (S.I.) conventions. by test, unit, sex, age, and effective date, and possibly by racial origin. Hematology data may require a bit more work. For example, the White Blood Cell (WBC) Differential is a ADJUST > OR < VALUES breakdown of the WBC into different cell types. Some Test results that are reported with a greater than (>) or laboratories report the WBC differentials as a percent of less than (<) sign (e.g., > 100), cannot be used in any the whole (%), while others may report them as statistical summaries unless the character value is absolute counts of cells. Depending on the converted to a numeric. Our convention has been to requirements of any statistical analyses, it may be add ‘1’ to the decimal past the significant decimal for necessary to convert the absolute counts into a percent the test. For example, if the test result has one of the whole (or vice versa). significant decimal, we would add a value of ‘0.01’ to the numeric part of the result for use in statistical Urinalysis data is the most complex data type, and summaries. according to many, frequently the least useful! However, deal we must. An issue that arises with urinalysis is the subjective nature of one of the testing UNIT CONVERSIONS methodologies. The urine sample is viewed under a The next step is to attempt to insure that all of each microscope, and the person viewing the sample reports particular test result across the study is reported in the what he/she sees. This may result in a test result that same unit of measurement. This is necessary for consists of words (such as ‘Cloudy’), a range of appropriate summarization of a particular test’s results numbers (such as 5-10 for a count of cells) or a mix of across the study. If multiple laboratories were used for words and numbers (RBC: 5-10). This may seem fine analyzing samples, chances are some unit conversions to a reviewer, but makes for difficult statistical will be required prior to any analyses. Note that the summarization. reference range-flagging step occurs prior to any unit conversions. If unit conversions are necessary, the range limits will be converted as well as the test results. SO WHERE DO WE START? It is more conservative to flag the results prior to Our approach has been to perform all necessary data converting, since the conversion could make a manipulations in what we call a derived data set difference in the flagging. program. For laboratory data, we have a skeleton front- end program that the programmer modifies to fit the study. This front-end program then invokes a main ABSOLUTE HEMATOLOGY CONVERSIONS macro, which in turn invokes smaller specialized As mentioned earlier, it may be necessary to convert macros. Some of the specialized macros are optional, the absolute hematology results. This conversion depending upon parameters set in the front-end requires that the sample has the WBC count program. These steps are described in more detail successfully reported, as it is the basis for converting below. from % of whole to an absolute count or vice versa. CONVERTING CHARACTER RESULTS VISIT SLOTTING / UNSCHEDULED TESTS In order to summarize and report laboratory results, we Laboratory data is often collected at unscheduled need to do our best to convert as much of the character intervals in addition to the protocol-specified visit results to numeric results (where it makes sense to do schedule. The investigator may see a test value that is so). For instance, you probably wouldn’t convert out of range for a patient and decide to re-perform the ‘Cloudy’ to a numeric result (although tests with discrete test prior to the patient’s next scheduled visit.
Details
-
File Typepdf
-
Upload Time-
-
Content LanguagesEnglish
-
Upload UserAnonymous/Not logged-in
-
File Pages5 Page
-
File Size-