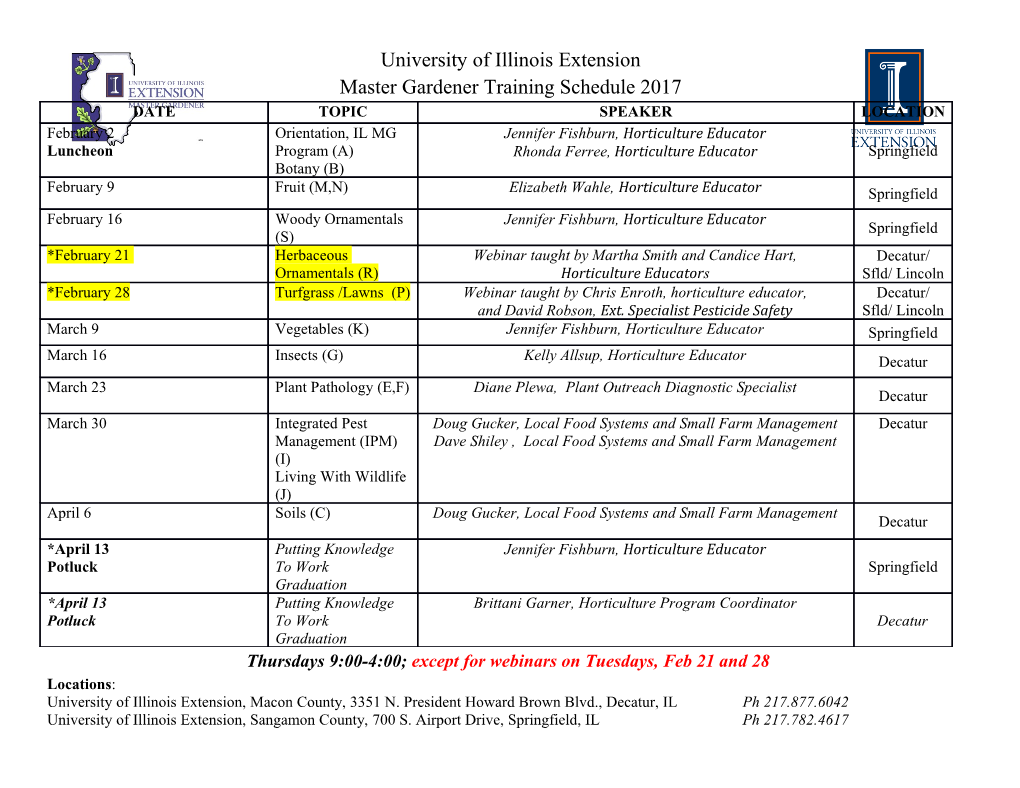
Integrating Apache Arrow and FPGAs on OpenPOWER Johan Peltenburg Delft University of Technology Join the Conversation #OpenPOWERSummit Outline • Serialization overhead in big data frameworks • Apache Arrow in-memory format • An FPGA acceleration framework • Regular expression matching experiment • Conclusion 2 Big Data Analytics Processing Frameworks Landscape • Cluster computing & analytics • Application level languages frameworks: • Java • Hadoop Spark • Scala • Flink Storm • Python • Samza Pandas • R • Drill Impala • MATLAB • … • … • Most run on JVM, some parts in C/C++ • A huge variety of tools and • Countless libraries / extensions languages used 3 Let’s attach an accelerator to a JVM • Data is in run-time objects managed by VM • Where are they? • Allocated by the VM in an “unknown” place • Can be subject to Garbage Collection • What do they look like? • Not standardized • Determined by VM implementation. • For OpenJDK: • Header • Pointer to class • Other bits for monitors, threads, etc… • Fields • We must “serialize” 4 Example: serialize collection of strings • Traverse the collection object reference • Traverse the reference to an array of references • For every string: • Traverse the reference to the string object • Traverse the character array reference • Pay a lot of latency to retrieve small amount of bytes • Many individual copies of short character arrays 5 (De)serialization 6 Serialization throughput TACC POWER8 node with OpenJDK. Small objects (p = 2, a=1, e=16, N=220) 7 Apache Arrow & Fletcher 8 Schema X { Arrow format crash course A: Float (nullable) B: List<Char> C: Struct{ A table: E: Int16 Index A B C F: Double } 0 1.33f beer {1, 3.14} } 1 7.01f is {5, 1.41} Index Data 2 ∅ tasty {3, 1.61} Offset Data 0 1 0 ‘b’ 1 5 1 ‘e’ 2 3 2 ‘e’ Buffers in Index Data Index Offset 3 ‘r’ Index Data memory: 0 0 4 ‘i’ 0 3.14 0 1.33f 1 4 5 ‘s’ 1 1.41 1 7.01f 2 6 2 1.61 2 X 6 ‘t’ Index Valid 3 11 7 ‘a’ 0 1 8 ‘s’ 1 1 9 ‘t’ 2 0 9 10 ‘y’ Fletcher: Arrow and FPGA, general approach 10 Schema X { A: Float (nullable) B: List<Char> A: Fixed length data C: Struct{ E: Int16 (with validity bitmap) F: Double } } Index Data 0 1.33f 1 7.01f 2 X Index Valid 0 1 1 1 2 0 ● User streams in first and last index in the table. ● Internal command stream: ● Column Reader streams the requested rows in order. – First element offset in the data word. – No. valid elements in the data word. ● Response handler aligns and serializes or parallelizes the data. 11 Schema X { A: Float (nullable) B: List<Char> C: Struct{ B: Variable length data E: Int16 (without validity bitmaps) F: Double } } Offset Data 0 ‘b’ 1 ‘e’ 2 ‘e’ 3 ‘r’ 4 ‘i’ 5 ‘s’ Index Offset 6 ‘t’ 0 0 7 ‘a’ 1 4 8 ‘s’ 2 6 9 ‘t’ 12 3 11 10 ‘y’ 12 Schema X { A: Float (nullable) B: List<Char> C: Structs C: Struct{ E: Int16 (without validity bitmaps) F: Double } } Index Data 0 1 1 5 2 3 Index Data 0 3.14 1 1.41 2 1.61 13 Other hardware features ● Parameterizable: Special thanks to: – Data & address widths at host memory interface Jeroen van Straten – Burst lengths, FIFO depths, optional register slices on all streams – No. elements per cyle in output ● Nested lists, lists in structs, structs in list, etc… are supported. ● Not using any vendor IP, but synthesizes in Vivado & Quartus ● Extensive verification through automatic test bench generation ( > 10 000 random schemas tested) – For example: ● Struct(List(List(Struct(Float, List(Struct(Int, Prim(1), String)), List(Boolean)), Int)), Double) – Also varies parameters mentioned above 14 Regular expression matching experiment R=16 different regular expressions per unit AWS EC2 F1: • Virtex Ultrascale+ • N=16 regex units • 256 regexes being matched in parallel POWER8 CAPI: • AlphaData KU3 (Kintex Ultrascale) • N=8 regex units • 128 regex being matched in parallel 15 Results (1/2) AWS EC2 F1 CAPI SNAP 16 AWS EC2 F1 (Intel Xeon) Results (2/2) POWER8+CAPI 17 Conclusion • Serialization may cause significant bottlenecks in big data frameworks • Prevents effective deployment of accelerators in some cases • Apache Arrow can help to alleviate bottlenecks • We created an FPGA interface generation framework for Arrow • Fletcher works with SNAP 18.
Details
-
File Typepdf
-
Upload Time-
-
Content LanguagesEnglish
-
Upload UserAnonymous/Not logged-in
-
File Pages18 Page
-
File Size-