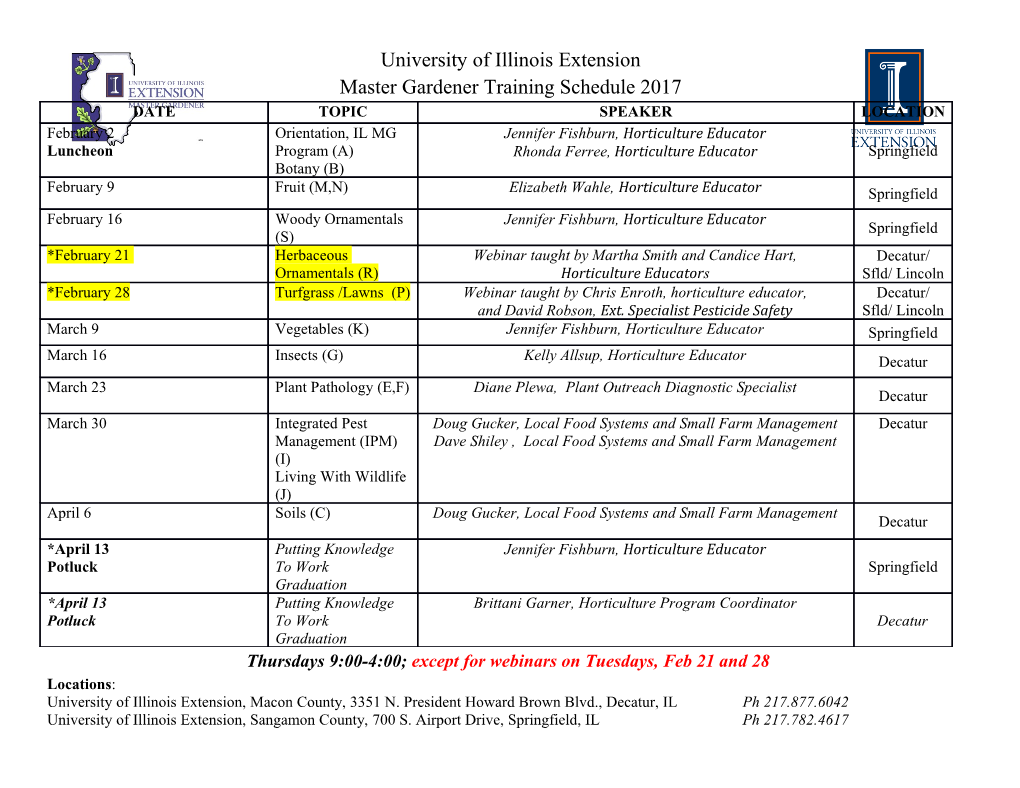
A Framework for Semantic Similarity Measures to enhance Knowledge Graph Quality Zur Erlangung des akademischen Grades Doktor der Ingenieurwissenschaften der Fakultät für Wirtschaftswissenschaften Karlsruher Institut für Technologie (KIT) genehmigte Dissertation von Dipl.-Ing. Ignacio Traverso Ribón Tag der mündlichen Prüfung: 10. August 2017 Referent: Prof. Dr. York Sure-Vetter Korreferent: Prof. Dr. Maria-Esther Vidal Serodio Abstract Precisely determining similarity values among real-world entities becomes a building block for data driven tasks, e.g., ranking, relation discovery or integration. Semantic Web and Linked Data initiatives have promoted the publication of large semi-structured datasets in form of knowledge graphs. Knowledge graphs encode semantics that describes resources in terms of several aspects or resource characteristics, e.g., neighbors, class hierarchies or attributes. Existing similarity measures take into account these aspects in isolation, which may prevent them from delivering accurate similarity values. In this thesis, the relevant resource characteristics to determine accurately similarity values are identified and considered in a cumulative way in a framework of four similarity measures. Additionally, the impact of considering these resource characteristics during the computation of similarity values is analyzed in three data-driven tasks for the enhancement of knowledge graph quality. First, according to the identified resource characteristics, new similarity measures able to combine two or more of them are described. In total four similarity measures are presented in an evolutionary order. While the first three similarity measures, OnSim, IC-OnSim and GADES, combine the resource characteristics according to a human defined aggregation function, the last one, GARUM, makes use of a machine learning regression approach to determine the relevance of each resource characteristic during the computation of the similarity. Second, the suitability of each measure for real-time applications is studied by means of a theoretical and an empirical comparison. The theoretical comparison consists on a study of the worst case computational complexity of each similarity measure. The empirical comparison is based on the execution times of the different similarity measures in two third-party benchmarks involving the comparison of semantically annotated entities. Ultimately, the impact of the described similarity measures is shown in three data-driven tasks for the enhance- ment of knowledge graph quality: relation discovery, dataset integration and evolution analysis of annotation datasets. Empirical results show that relation discovery and dataset integration tasks obtain better results when considering semantics encoded in semantic similarity measures. Further, using semantic similarity measures in the evolution analysis tasks allows for defining new informative metrics able to give an overview of the evolution of the whole annotation set, instead of the individual annotations like state-of-the-art evolution analysis frameworks. i Acknowledgements Even when only my name is written in the cover of this thesis, this document would not exist without the help of several people: Without listing everybody by name, I wish to thank these important persons in my life. I would like to express my profound gratitude to Prof. Dr. Rudi Studer and Prof. Dr. York Sure-Vetter for allowing me to work in such a good environment and for their support and supervision during my PhD. I am also proud of having Prof. Dr. Maria-Esther Vidal as co-advisor. Thanks to her guidance and support I learn how to be more confident and trust into my own work. I would like to name Stefan Zander and Suad Sejdovic as two of my best friends in Karlstuhe, who strongly supported me during my time at FZI and my beginning in Germany. Both of them are unconditional colleagues and friends, always available for helping with any kind of issue. Further, a deep thank you for Heike Döhmer and Bernd Ziesche for teaching me how does FZI works and how to speak and write German respectively. Thanks also to all the people external to FZI and AIFB who helped me during my life and made possible that I am writing these lines today. Particularly, I would like to express my gratitude to Laura Padilla, who accompanied me during the last five years in the bad and good moments. Finally, I want to thanks my parents because thanks to their sacrifice, work, education and support from the distance I am here today. Thank you very much / Muchas gracias a todos. Karlsruhe, August 10, 2017 Ignacio Traverso Ribón iii Contents 1 Introduction ................................................ 1 1.1 Motivation . 1 1.2 Research Questions and Contributions . 2 1.3 Running Example . 3 1.4 Previous Publications . 4 1.5 Guide to the Reader . 5 2 Foundations ................................................ 7 2.1 Similarity Measure . 7 2.1.1 Triangular Norm . 8 2.2 Knowledge Graphs . 9 2.2.1 RDF: Resource Description Framework . 10 2.2.2 RDF Schema and OWL . 10 2.2.3 Annotation Graph . 12 2.2.4 Knowledge Bases . 12 2.2.5 SPARQL . 13 2.3 Graph Partitioning . 14 2.4 Bipartite Graph . 15 2.4.1 1-1 Maximum Weighted Perfect Matching . 15 2.5 Comparison of Sets . 16 3 Related Work ............................................... 19 3.1 Taxonomic Similarity Measures . 19 3.1.1 Dps .............................................. 19 3.1.2 Dtax .............................................. 19 3.2 Path Similarity Measures . 20 3.2.1 PathSim . 20 3.2.2 HeteSim . 21 3.2.3 SimRank . 21 3.3 Information Content Similarity Measures . 22 3.3.1 Resnik . 22 3.3.2 Redefinitions of Resnik’s Measure . 23 3.3.3 DiShIn . 23 3.4 Attribute Similarity Measures . 23 3.5 Combined Similarity Measures . 25 3.5.1 GBSS . 25 3.6 Relation Discovery . 25 3.7 Evolution of Annotation Graphs . 26 3.8 Knowledge Graph Integration . 27 v Contents 4 Semantic Similarity Measure Framework .............................. 29 4.1 Introduction . 29 4.2 Semantic Similarity Measures on Ontologies: OnSim and IC-OnSim . 30 4.2.1 OnSim . 30 4.2.2 IC-OnSim . 37 4.2.3 Theoretical Properties . 39 4.2.4 Empirical Properties . 42 4.3 Semantic Similarity Measures on Graph Structured Data: GADES . 48 4.3.1 Motivating Example . 48 4.3.2 GADES Architecture . 49 4.3.3 Theoretical Properties . 52 4.3.4 Empirical Properties . 54 4.4 Automatic Aggregation of Individual Similarity Measures: GARUM . 57 4.4.1 Motivating Example . 58 4.4.2 GARUM: A Machine Learning Based Approach . 58 4.4.3 Evaluation . 60 4.5 Conclusions . 62 5 Semantic based Approaches for Enhancing Knowledge Graph Quality ........... 63 5.1 Introduction . 63 5.2 Relation Discovery in Knowledge Graphs: KOI . 64 5.2.1 Motivating Example . 64 5.2.2 Problem Definition . 65 5.2.3 The KOI Approach . 66 5.2.4 Empirical Evaluation . 70 5.3 Evolution of Annotation Datasets: AnnEvol . 73 5.3.1 Motivating Example . 74 5.3.2 Evolution of Ontology-based Annotated Entities . 76 5.4 Integration of Graph Structured Data: Fuhsen . 84 5.4.1 Motivating Example . 84 5.4.2 FuhSen: A Semantic Integration Approach . 86 5.4.3 Empirical Evaluation . 86 5.4.4 Integration of DBpedia and Wikidata Molecules . 89 5.5 Conclusions . 90 6 Conclusions and Future Work ..................................... 93 6.1 Discussion of Contributions . 93 6.2 Overall Conclusions . 96 6.3 Outlook . 96 A Appendix: Proof of Metric Properties for GADES ......................... 99 vi 1 Introduction Identity and Difference are terms whose meaning have been occupying the thoughts of philosophers throughout history. Aristotle formulated the Law of Identity in logic and this law is still valid today. The usual formulation is A ≡ A, which means that every thing is identical to itself. Two things are considered identical if they share completely the same properties. In contrary case, they are different. This binary relation is not enough for the understanding of the human being. Humans are able to estimate the degree of difference between two things in a fuzzy way. The.
Details
-
File Typepdf
-
Upload Time-
-
Content LanguagesEnglish
-
Upload UserAnonymous/Not logged-in
-
File Pages134 Page
-
File Size-