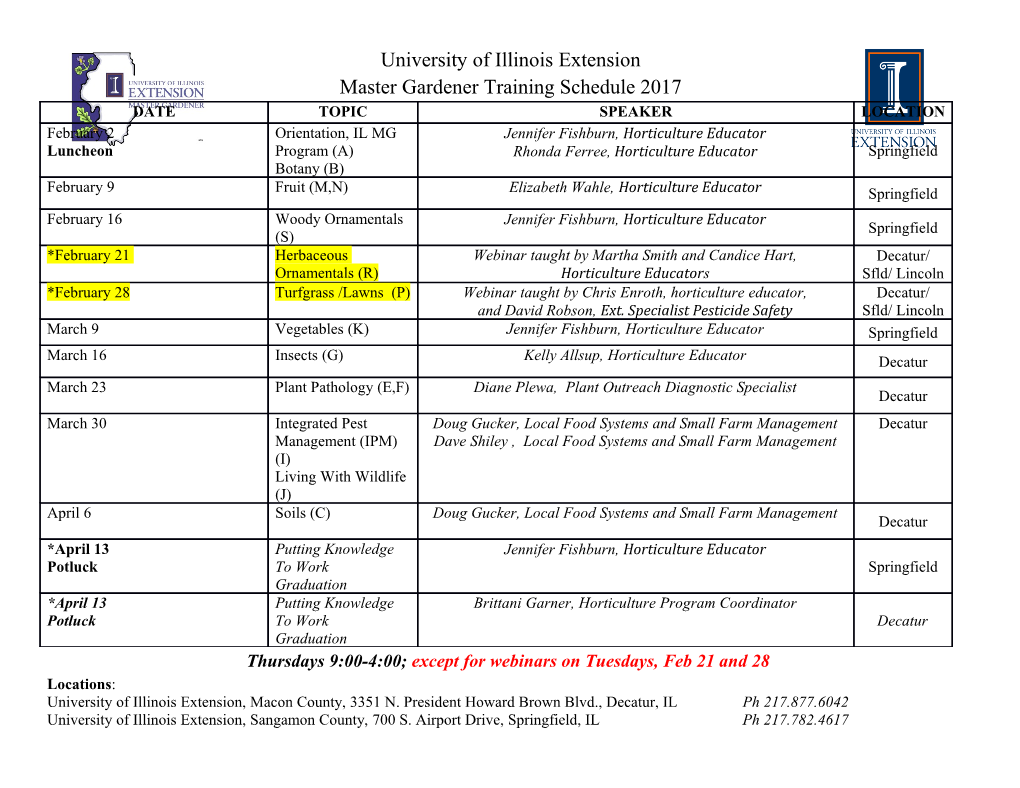
Motivation: Moore’s Law • Every two years: – Double the number of transistors CprE 488 – Embedded Systems Design – Build higher performance general-purpose processors Lecture 8 – Hardware Acceleration • Make the transistors available to the masses • Increase performance (1.8×↑) Joseph Zambreno • Lower the cost of computing (1.8×↓) Electrical and Computer Engineering Iowa State University • Sounds great, what’s the catch? www.ece.iastate.edu/~zambreno rcl.ece.iastate.edu Gordon Moore First, solve the problem. Then, write the code. – John Johnson Zambreno, Spring 2017 © ISU CprE 488 (Hardware Acceleration) Lect-08.2 Motivation: Moore’s Law (cont.) Motivation: Dennard Scaling • The “catch” – powering the transistors without • As transistors get smaller their power density stays melting the chip! constant 10,000,000,000 2,200,000,000 Transistor: 2D Voltage-Controlled Switch 1,000,000,000 Chip Transistor Dimensions 100,000,000 Count Voltage 10,000,000 ×0.7 1,000,000 Doping Concentrations 100,000 Robert Dennard 10,000 2300 Area 0.5×↓ 1,000 130W 100 Capacitance 0.7×↓ 10 0.5W 1 Frequency 1.4×↑ 0 Power = Capacitance × Frequency × Voltage2 1970 1975 1980 1985 1990 1995 2000 2005 2010 2015 Power 0.5×↓ Zambreno, Spring 2017 © ISU CprE 488 (Hardware Acceleration) Lect-08.3 Zambreno, Spring 2017 © ISU CprE 488 (Hardware Acceleration) Lect-08.4 Motivation Dennard Scaling (cont.) Motivation: Dark Silicon • In mid 2000s, Dennard scaling “broke” • Dark silicon – the fraction of transistors that need to be powered off at all times (due to power + thermal constraints) Transistor: 2D Voltage-Controlled Switch Area 0.5×↓ Dimensions Power 0.5×↓ Voltage ×0.7 • Evolution of processors strongly motivated by this ending of Doping Dennard scaling Concentrations – Expected continued evolution towards HW specialization / acceleration Area 0.5×↓ Capacitance 0.7×↓ Frequency 1.4×↑ Power = Capacitance × Frequency × Voltage2 Power 0.5×↓ 2015 Zambreno, Spring 2017 © ISU CprE 488 (Hardware Acceleration) Lect-08.5 Zambreno, Spring 2017 © ISU CprE 488 (Hardware Acceleration) Lect-08.6 This Week’s Topic Straight from the Headlines... • Accelerating a diverse range of applications using • Hardware Acceleration: reconfigurable logic is a trending area: – D. Hoang, D. Lopresti, “FPGA Implementation of – Performance analysis and overhead Systolic Sequence Alignment” – D. Ross, O. Vellacott, M. Turner, “An FPGA-based – Coprocessors vs accelerators Hardware Accelerator for Image Processing” – J. Lockwood, “Design and Implementation of a – Common acceleration techniques Multicast, Input-Buffered ATM Switch for the iPOINT Testbed” – Acceleration examples • What these examples have in common: – Illustrative of the potential for custom computing to enable faster scientific and engineering • Reading: Wolf section 10.4-10.5 discovery – Relatively small impact (outside of academia) – All work done in 1992 Zambreno, Spring 2017 © ISU CprE 488 (Hardware Acceleration) Lect-08.7 Zambreno, Spring 2017 © ISU CprE 488 (Hardware Acceleration) Lect-08.8 And Today? A (Brief) Background • Reconfigurable logic supporting the data center, • Earliest reconfigurable computer proposed at UCLA in the 1960s – G. Estrin et al., “Parallel Processing in a Restructurable Computer specializing clusters , and tightly integrated on-chip System,” IEEE Trans. Electronic Computers, pp. 747-755, Dec. 1963. – Basic concepts well ahead of the enabling technology – could only prototype a crude approximation • Current chips – contain memory cells that hold both configuration and state information – Only a partial architecture exists before programming – After configuration, the device provides an execution environment for a specific application • Goal is to adapt at the logic-level to solve specific problems Zambreno, Spring 2017 © ISU CprE 488 (Hardware Acceleration) Lect-08.9 Zambreno, Spring 2017 © ISU CprE 488 (Hardware Acceleration) Lect-08.10 A (Brief) Background So Who Cares? • Today, well known niches for FPGAs: • Claim – reconfigurable processors offer a definite advantage over – Emulation general-purpose counterparts with regards to functional density – Telecom – A. DeHon. “The Density Advantage of Configurable Computing,” IEEE – Space / defense Computer, pp. 41-49, Apr. 2000 – Computations per chip area per cycle time • $4.5B market, major device manufacturers: – Xilinx (~45% market share) – Altera (~40%) • Considering general computing trends (Moore’s Law, Dennard “Vodafone Sure Signal: Inside a – Microsemi / Actel (10%) Femtocell” – http://zdnet.com (Xilinx Scaling, “dark” silicon) – what can I get for my N billion transistors? – Others (Lattice, Achronix, Tabula) Spartan 3E FPGA used for glue logic) Altera Stratix IV EP4S40G5 Intel Haswell Core i7-5960X “Sneak Peak: Inside NVIDIA’s Emulation Lab” “How Mars Rover Got its ‘Dream Mode’” – – http://blogs.nvidia.com (Cadence Palladium http://eetimes.com (Microsemi FPGAs cluster used for GPU emulation) reprogrammed for surface exploration) Zambreno, Spring 2017 © ISU CprE 488 (Hardware Acceleration) Lect-08.11 Zambreno, Spring 2017 © ISU CprE 488 (Hardware Acceleration) Lect-08.12 Accelerated Systems Accelerator vs. Coprocessor • Use additional computational units dedicated to • A coprocessor executes instructions some functionality – Instructions are dispatched by the CPU • Hardware/software co-design: joint design of • An accelerator appears as a device on the bus hardware and software architectures – Typically controlled by registers (memory-mapped request IO) accelerator result datadata CPU memory I/O Zambreno, Spring 2017 © ISU CprE 488 (Hardware Acceleration) Lect-08.13 Zambreno, Spring 2017 © ISU CprE 488 (Hardware Acceleration) Lect-08.14 EX-08.1: Instruction Augmentation Accelerated System Design • Processor can only describe a small number • First, determine that the system really needs to of basic computations in a cycle be accelerated I – How much faster will the accelerator on the core – I bits -> 2 operations function? • Many operations could be performed on 2 W- – How much data transfer overhead? Compute bound bit words vs memory bound vs I/O bound? • ALU implementations restrict execution of • Design accelerator and system interface some simple operations – e. g. bit reversal • If tighter CPU integration required: a31 a30………. a0 – Create a functional unit for augmented instructions Swap bit – Compiler techniques to identify/use new functional positions unit b31 b0 Zambreno, Spring 2017 © ISU CprE 488 (Hardware Acceleration) Lect-08.15 Zambreno, Spring 2017 © ISU CprE 488 (Hardware Acceleration) Lect-08.16 Amdahl’s Law Heterogeneous Execution Model • If an optimization improves a fraction f of execution time by a factor of a instructions executed over time Told 1 Speedup [(1 f ) f / a]Told (1 f ) f / a 49% of initialization code 0.5% of run time • This formula is known as Amdahl’s Law co-processor “hot” loop • Lessons from 1% of code 99% of run time – If f →100%, then speedup = a – If a →∞, the speedup = 1 / (1 – f ) 49% of clean up • Summary code 0.5% of run time – Make the common case fast – Watch out for the non-optimized component Gene Amdahl Zambreno, Spring 2017 © ISU CprE 488 (Hardware Acceleration) Lect-08.17 Zambreno, Spring 2017 © ISU CprE 488 (Hardware Acceleration) Lect-08.18 Heterogeneous Computing: Performance Hardware/Software Partitioning Move “bottleneck” computations from software to • C Code for FIR Filter Hardware ‘for’ loop hardware Hardware/software* * * * * * * * * partitioning* * * . Designer creates forfor (i=0;(i=0; i i << 16;128; i++) i++) custom accelerator y[i]y[i] +=+= c[i]c[i] ** x[i]x[i] selects+ +performance + + + critical+ . regions. .... using hardware • Example: for hardware implement . .... + + + design methodology .... – Application requires a week of CPU time + + . – One computation consumes 99% of execution time + . Compiler 100 90 Kernel Application Execution 80 speedup speedup time 70 60 50 Hw/Sw 50 34 5.0 hours 50 Sw 40 Sw 100 50 3.3 hours Processor Processor Processor FPGA 30 20 200 67 2.5 hours 10 0 500 83 2.0 hours TimeTime EnergyEnergy 1000 91 1.8 hours • ~1000 cycles ~ 10 cycles Speedup = 1000 cycles/ 10 cycles = 100x Zambreno, Spring 2017 © ISU CprE 488 (Hardware Acceleration) Lect-08.19 Zambreno, Spring 2017 © ISU CprE 488 (Hardware Acceleration) Lect-08.20 High-Level Synthesis Accelerator Design Challenges HighUpdated-level • Debugging – how to properly test the accelerator separately, BinaryCode • Problem: Describing circuit and then in conjunction with the rest of the system (hw/sw using HDL is time co-simulation) HW/SWDecompilation Partitioning consuming/difficult • Coherency – how to safely share results between CPU and Compiler DecompilatioHigh-level • Solution: High-level accelerator Synthesisn synthesis – Impact on cache design, shared memory – Solutions looks similar to those for resource sharing in Libraries/Libraries/ Software Hardware – Create circuit from high-level conventional operating systems, but are typically ad-hoc ObjectObject code CodeCode Linker – Allows developers to use • Analysis – determining the effects of any hardware higher-level specification parallelism on performance Bitstream Bitstream – Potentially, enables synthesis – Must take into account accelerator execution time, data transfer for software developers time, synchronization overhead – Heterogeneous multi-threading helps, but complicates design uP FPGA significantly • More on this in a bit – Overlapping I/O and computation (streaming) Zambreno, Spring 2017 © ISU CprE 488 (Hardware Acceleration)
Details
-
File Typepdf
-
Upload Time-
-
Content LanguagesEnglish
-
Upload UserAnonymous/Not logged-in
-
File Pages13 Page
-
File Size-