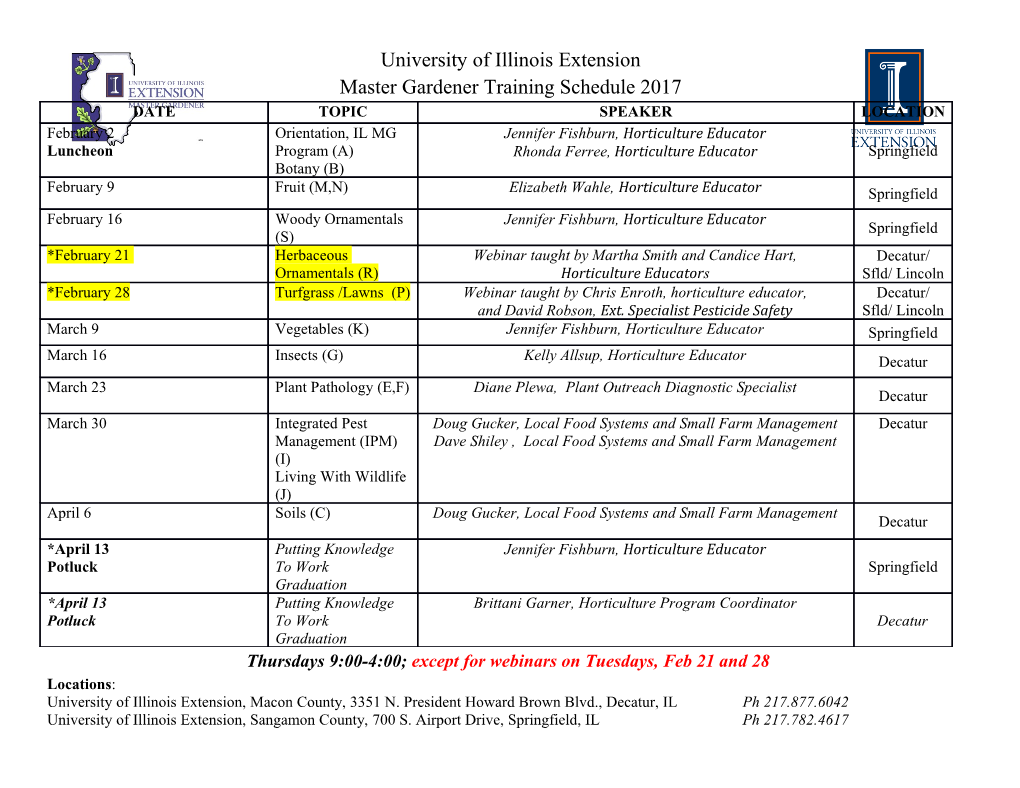
9/16/2016 CHE384, From Data to Decisions: Measurement, Leverage During Regression Uncertainty, Analysis, and Modeling • During a regression, some data points have Lecture 21 more leverage than others – Leverage points = data with an extreme value Leverage in Regression of the predictor variable (x) Chris A. Mack • Like outliers, high leverage data can have Adjunct Associate Professor outsized influence on the regression results – We’ll define “influence” more exactly later http://www.lithoguru.com/scientist/statistics/ © Chris Mack, 2016Data to Decisions 1 © Chris Mack, 2016Data to Decisions 2 Defining Leverage Defining Leverage • Leverage can be quantified by looking at the distances • For the case of only one predictor variable, between x’s (accounting for correlation) – In the matrix formulation of linear regression, this is given by the diagonal elements of the “hat” matrix H (also called the 1 ̅ 1 1 ̅ projection matrix): , (from Lecture 6) ∑ ̅ 1 , ≡ 0 1 • For two or more predictor variables (multiple regression), we use matrix math to calculate the hat matrix and its Number of diagonal elements parameters Note that so that in the model © Chris Mack, 2016Data to Decisions 3 © Chris Mack, 2016Data to Decisions 4 From the Anscombe Problems Looking at Residuals (recall Lecture 8) • Our model is , , ~ 0, iid • But when we estimate the bk’s with bk’s, the hii = 1 resulting residuals ei do not have a constant variance hii = 0.1 – High leverage points have lower variance © Chris Mack, 2016Data to Decisions 5 © Chris Mack, 2016Data to Decisions 6 1 9/16/2016 Residual Variance From the Anscombe Problems • For ~ 0, , our true residuals have a This residual constant variance , with unbiased has zero variance! estimator 1 The fit always hii = 1 passes exactly through this • But the variance of each fit residual ei is point. h = 0.1 1 ii 1 © Chris Mack, 2016Data to Decisions 7 © Chris Mack, 2016Data to Decisions 8 Studentized Residuals Studentized Residuals • When doing statistical tests on residuals • Internally Studentized Residual: all data are (Grubbs’ test, skewness, etc.) one must included in the calculation studentize the residuals first This distribution is complicated 1 1 • Externally Studentized Residual: the ith data point Called “internally studentized residual” or “standardized residual” is excluded from the calculation of se Also called • Since the SE of the residual varies, tests t-distributed with 1 “studentized DF = n – p – 1 should always be done on the studentized deleted residual” [for e ~ N(0,s )] residuals, not the raw residuals e © Chris Mack, 2016Data to Decisions 9 © Chris Mack, 2016Data to Decisions 10 Testing the Residuals Williams Graph • To see the possibility for both outliers and high-leverage • Perform statistical tests on the esr data, plot the externally studentized residual (esr) versus (externally studentized residuals) only the normalized leverage (/) for each data point – QQ Plots Potential Outliers – Moment testing Grubbs Critical T – Outlier detection/rejection (Grubbs, etc.) High |esr| – More to come … leverage • This is because the esr has a simple sampling distribution: Student’s t Twice the average leverage © Chris Mack, 2016Data to Decisions 11 © Chris Mack, 2016Data to Decisions 12 2 9/16/2016 Lecture 21: What have we learned? • Define leverage • What is the role of the hat matrix in determining leverage? • What is the difference between internally and externally studentized residuals? • How should residuals be studentized for statistical testing? • Know how to create and interpret the Williams Graph © Chris Mack, 2016Data to Decisions 13 3.
Details
-
File Typepdf
-
Upload Time-
-
Content LanguagesEnglish
-
Upload UserAnonymous/Not logged-in
-
File Pages3 Page
-
File Size-