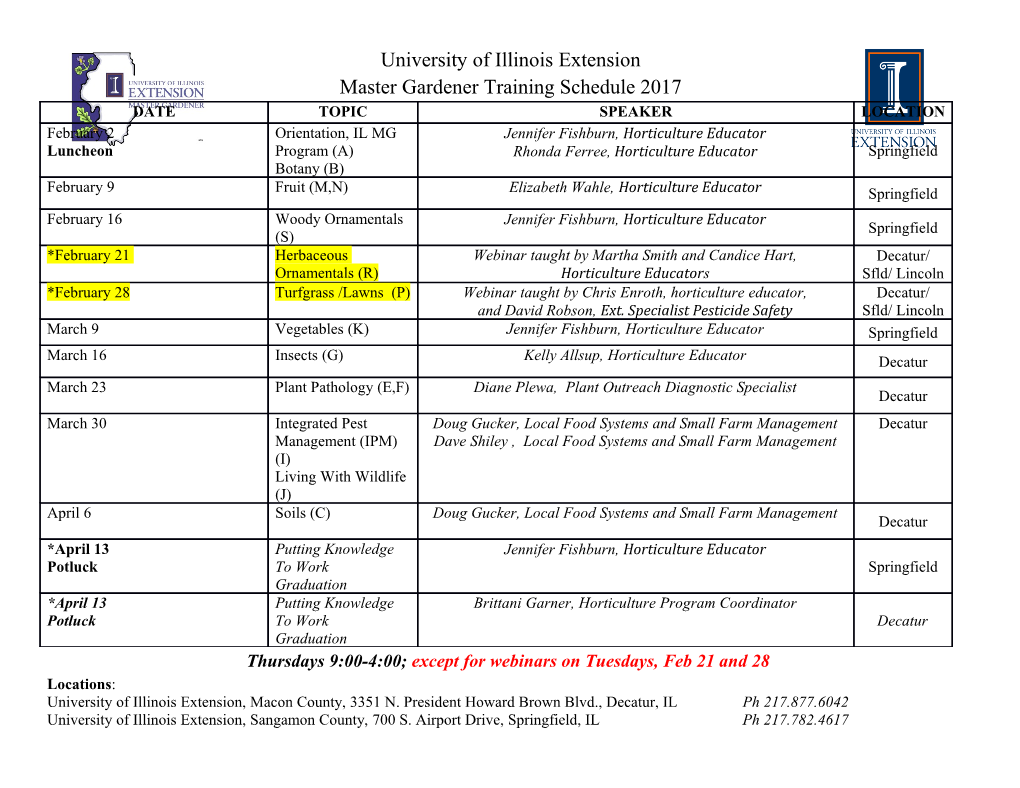
ArrayFire: Open Source An Introduction to the ArrayFire Library ● We make code run faster ○ Started in 2007 by Georgia Tech researchers ArrayFire—The People ● Diverse research background ○ HPC ○ Computer vision ○ Machine learning ○ Image processing ○ Social network analysis ○ Optical interferometry ○ Computer graphics ● From all over: Georgia Tech, U. Penn., Clemson, GSU, Texas A&M, UCSD, and more ● Passionate about making things faster ArrayFire—R&D ● Case studies on image analysis for massive datasets ● Large scale triangle counting on multiple GPUs ● Virtual fitting of glasses ● Computer aided logo detection ● Sleep disorder diagnosis ArrayFire—The Library ● The Library ○ GPU accelerated high performance library ○ Extensive set of built in functions ○ Applicable for numerous domains ■ Science ■ Engineering ■ Finance ■ Biology ○ Great for mobile and embedded computing Libraries are Great Eliminate Hidden Costs Library Types ● Specialized GPU library ○ Targeted at a specific set of operators (functionality) ■ Precision tools ○ Optimized for specific systems ○ C-like interface ○ Raw pointer interface Images taken from: http://classroom.synonym.com/learn-surgical-instruments-2429.html Library Types (cont.) ● General GPU library ○ Manage GPU resources using containers ○ Applicable to a large set of applications and domains ○ Portable across multiple architectures ○ Higher level functions ○ Can make use of specialized GPU libraries Images taken from: http://wordlesstech.com/2010/12/09/swiss-army-knife-giant-elite/ ArrayFire Capabilities ● Hundreds of parallel functions for multi-disciplinary work ○ Image processing ○ Machine learning ○ Graphics ○ Sets ● Support for multiple languages ○ C/C++, Fortran, Java and R ● Multiple backends - great for portability ○ CUDA ○ OpenCL ○ CPU (requires gcc) ArrayFire Capabilities (cont.) ● Linux, Windows, Mac OS X ● OpenGL based graphics ● JIT ○ Combine multiple operations into one kernel ArrayFire Functions ● Hundreds of highly-optimized parallel functions ○ Signal/image processing ■ Convolution ■ FFT ■ Histograms ■ Interpolation ■ Connected components ○ Linear Algebra ■ Matrix multiply ■ Linear system solving ■ Factorization ArrayFire Functions ● Supports hundreds of parallel functions ○ Building blocks ■ Reductions ■ Scan ■ Set operations ■ Sorting ■ Statistics ■ Basic matrix manipulation Images taken from: http://technogems.blogspot.com/2011/06/sorting-included-files-by-importance.html http://www.cmsoft.com.br/tutorialOpenCL/CLMatrixMultExplanationSubMatrixes.png ArrayFire—Data Structures ● Built around a flexible data structure named "array" ○ Lightweight wrapper around the data on the compute device ○ Manages the data and basic metadata such as size, type and dimensions ● You can transfer data into an array using constructors ● Column major float hA[6] = {0, 1, 2, 3, 4, 5}; array A(2, 3, hA); Introducing... Open source! https://www.github.com/arrayfire/arrayfire ArrayFire—Indexing #include <arrayfire.h> #include <af/utils.h> void af_example() { float f[8] = {1, 2, 4, 8, 16, 32, 64, 128}; array a(2, 4, f); // 2 rows x 4 col array initialized with f values array sumSecondCol = sum(a(span, 1)); // reduce-sum over the second column print(sumSecondCol); // 12 } ArrayFire Example—Swap R and B array tmp = img(span,span,0); // save the R channel img(span,span,0) = img(span,span,2); // R channel gets values of B img(span,span,2) = tmp; // B channel gets value of R array swapped = join(2, img(span,span,2), // blue img(span,span,1), // green img(span,span,0)); // red array swapped = img(span,span,seq(2,-1,0)); ArrayFire Functions Original Grayscale Box Filter Blur Gaussian Blur Image Negative Erosion ArrayFire // erode an image, 8-neighbor connectivity array mask8 = constant(1,3, 3); array img_out = erode(img_in, mask8); // erode an image, 4-neighbor connectivity const float h_mask4[] = { 0.0, 1.0, 0.0, 1.0, 1.0, 1.0, 0.0, 1.0, 0.0 }; array mask4 = array(3, 3, h_mask4); array img_out = erode(img_in, mask4); Erosion Filtering ArrayFire array R = convolve(img, ker); // 1, 2 and 3d convolution filter array R = convolve(fcol, frow, img); // Separable convolution array R = filter(img, ker); // 2d correlation filter Histograms ArrayFire int nbins = 256; array hist = histogram(img,nbins); Transforms ArrayFire array half = resize(0.5, img); array rot90 = rotate(img, af::Pi/2); array warped = approx2(img, xLocations, yLocations); Image smoothing ArrayFire array S = bilateral(I, sigma_r, sigma_c); array M = meanshift(I, sigma_r, sigma_c, iter); array R = medfilt(img, 3, 3); // Gaussian blur array gker = gaussiankernel(ncols, ncols); array res = convolve(img, gker); FFT FFT (API) array R1 = fft2(I); // 2d fft. check fft, fft3 array R2 = fft2(I, M, N); // fft2 with padding array R3 = ifft2(fft2(I, M, N) * fft2(K, M, N)); // convolve using fft2 JIT Code Generation ● Run time kernel generation ● Combines multiple element wise operations into one kernel ● Reduces number of kernel launches ● Improves cache performance ○ Intermediate data not allocated JIT—Monte Carlo Pi Simulation array temp = (sqrt(x*x+y*y)<1); return sum<float>(temp); No JIT: ● 5 function calls (2”*”, 1 “+”, “ ”,1 “<”, 1 “√”) With JIT: ● One function call ● Fewer cache misses ○ “Capacity” cache misses avoided JIT Performance Results are from a K20 GPU Lower is better Additional Performance Results OpenCV ● Open source computer vision library ● C++ interface ● Some CUDA supported functionality OpenCV—ArrayFire Interoperability ● Helper Functions ○ https://github.com/arrayfire-community/arrayfire_opencv.git Mat R; // OpenCV API Rodrigues(poses(Rect(0, 0, 1, 3)), R); // OpenCV function call af::array af_R = mat_to_array(R); // Results transferred to array data-structure Feature Tracking—FAST Corner Detection ● In comparison with OpenCV CPU implementation ● Larger data-sets can be analyzed ● Single-threaded CPU implementation Feature Tracking—Harris Speedup ● Feature Detection algorithm ● Better performance ● Larger data-sets can be analyzed ● Single-threaded CPU implementation Feature Tracking—ORB Speedup ● Better performance ● Uses the FAST algorithm ○ At multiple scales ● Single-threaded CPU implementation Feature Tracking—Example (ORB) Speedup for sample: 21.6x Performance Results—Bilateral Filtering Performance Results—Convolution Performance Results—Image Rotate Performance Results—Bilinear Image Resize Performance insights ● Large images - GPUS are ideal. ● Smaller images - GPUs are great, but better performance can be achieved through batching and data parallelism. ○ With batching or data parallelism, GPU are also ideal. Conway’s Game of Life // Convolve gets neighbors af::array nHood = convolve(state, kernel, false); // Generate conditions for life af::array C0 = (nHood == 2); af::array C1 = (nHood == 3); // Update state state = state * C0 + C1; Open Source Roadmap ● Create capabilities for ○ Streaming video ○ Large number of images ○ Machine learning ○ Data analysis ○ Dynamic data ● Faster visualization/rendering utilities for large scale data sets Open Source Roadmap ● Support for sparse linear algebra ● Additional language wrappers ● More machine learning and computer vision functions ● Additional “big-data” infrastructure Look Us Up Company website: www.arrayfire.com Open source library: https://github. com/arrayfire/arrayfire Language wrapper {Fortran, R, Java}: https://github.com/arrayfire/arrayfire_$lang Q & A Speaker: Oded Green ([email protected]) Engineer: Shehzan Mohammed ([email protected]) Sales: Scott Blakeslee ([email protected]) TEST DRIVE GPU ACCELERATORS TODAY Accelerate your scientific discoveries: ✓ Reducing simulation time from hours to minutes ✓ Using the latest Tesla K80 GPUs FREE GPU Trial at: www.nvidia.com/GPUTestDrive UPCOMING LIVE GTC EXPRESS WEBINAR Thursday, December 18 Photorealistic Visualization with Speed and Ease Using Iray+ for Autodesk 3ds Max David Coldron, Lightwork Design and Peter de Lappe, NVIDIA ON-DEMAND GTC EXPRESS WEBINARS More than 100 GTC Express Webinar recordings. www.gputechconf.com/gtcexpress REGISTRATION IS OPEN! 20% OFF March 17-20, 2015 | San Jose, CA GM15WEB www.gputechconf.com #GTC15 CONNECT LEARN DISCOVER INNOVATE Connect with experts Get key learnings and Discover the latest Hear about disruptive from NVIDIA and other hands-on training in the technologies shaping innovations as early-stage organizations across a 400+ sessions and 150+ the GPU ecosystem start-ups present their work wide range of fields research posters.
Details
-
File Typepdf
-
Upload Time-
-
Content LanguagesEnglish
-
Upload UserAnonymous/Not logged-in
-
File Pages53 Page
-
File Size-