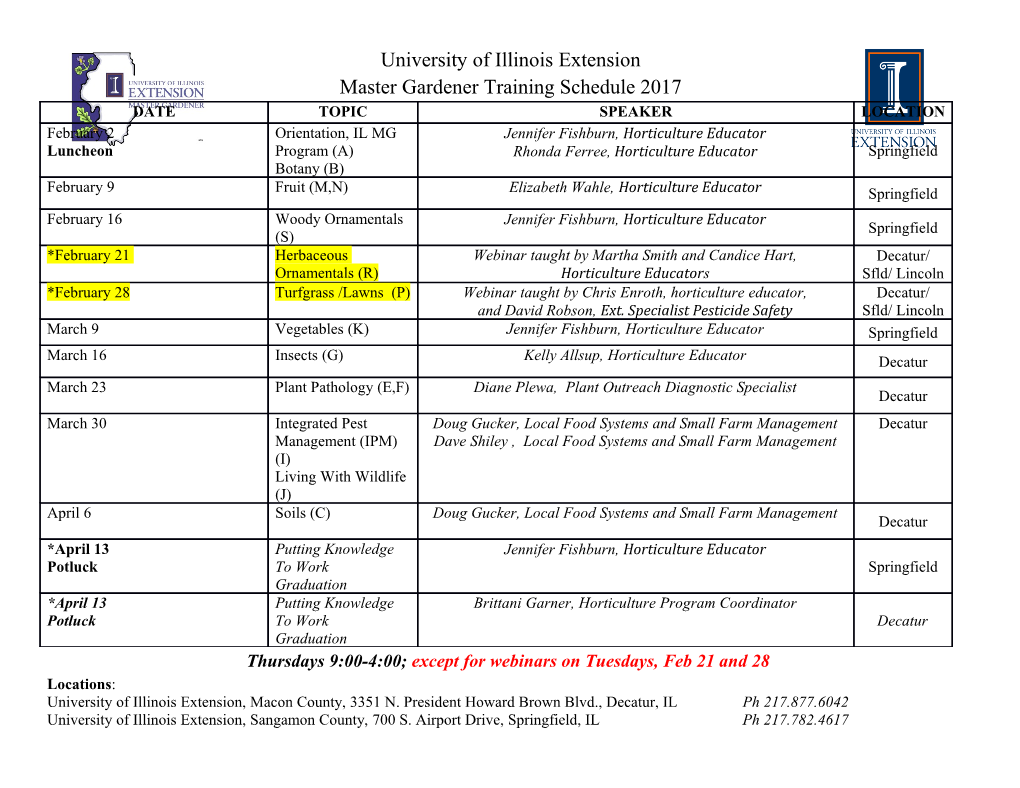
Journal of Econometrics 154 (2010) 139–153 Contents lists available at ScienceDirect Journal of Econometrics journal homepage: www.elsevier.com/locate/jeconom A comparison of two model averaging techniques with an application to growth empirics Jan R. Magnus a,∗, Owen Powell b, Patricia Prüfer c a Department of Econometrics & Operations Research, Tilburg University, The Netherlands b CentER, Tilburg University, The Netherlands c Netherlands Bureau for Economic Policy Analysis (CPB) and CentER, Tilburg University, The Netherlands article info a b s t r a c t Article history: Parameter estimation under model uncertainty is a difficult and fundamental issue in econometrics. Received 5 April 2008 This paper compares the performance of various model averaging techniques. In particular, it contrasts Received in revised form Bayesian model averaging (BMA) — currently one of the standard methods used in growth empirics — with 18 May 2009 a new method called weighted-average least squares (WALS). The new method has two major advantages Accepted 25 July 2009 over BMA: its computational burden is trivial and it is based on a transparent definition of prior ignorance. Available online 6 August 2009 The theory is applied to and sheds new light on growth empirics where a high degree of model uncertainty is typically present. JEL classification: C51 ' 2009 Elsevier B.V. All rights reserved. C52 C13 C11 Keywords: Model averaging Bayesian analysis Growth determinants 1. Introduction The second purpose is to contribute to the debate on growth empirics. Since the seminal studies of Kormendi and Meguire This paper has two purposes. First, it introduces a new model (1985) and Barro (1991), empirical research on the determinants averaging technique, called weighted-average least squares (here- of economic growth has identified numerous variables as being after WALS), which we claim to be theoretically and practically robustly (partially) correlated with productivity growth in an superior to standard Bayesian model averaging (BMA). It is theo- economy. Durlauf et al. (2005) list 145 potential right-hand side retically superior because it treats our ignorance about the priors variables for growth regressions and cluster them into more in a different manner, thereby obtaining a better risk profile and, than forty areas (or theories), such as human capital, finance, in particular, avoiding unbounded risk. It is practically superior be- government, and trade. Taking into account the limited number cause the space over which we need to perform model selection in- of observations available at a national level, growth empirics has been heavily criticized because of the inherent model uncertainty; creases linearly rather than exponentially in size. Thus, if we have see Durlauf et al. (2005) for a recent in-depth survey. sixty regressors to search over (which is not unusual in the growth Sometimes growth theory can support choices of specific literature), then computing time of standard BMA is of the order variables, but the inclusion or exclusion of most variables is 260, while computing time of WALS is of the order 60. This means typically arbitrary, a phenomenon labeled the `open-endedness' that what WALS can do in one second, BMA can only do in six hun- of growth theory (Brock and Durlauf, 2001). In addition, while dred million years. Exact computation of a complete BMA is there- theory may provide general qualitative variables (such as human fore rarely done; instead some Markov chain Monte Carlo (MCMC) capital), it does not tell us how these variables are to be specified method is typically applied. or measured. We are thus faced with (at least) two types of uncertainty, each of which brings about model uncertainty. Since there exist a wide set of possible model specifications, we ∗ Corresponding address: Department of Econometrics & OR, Tilburg University, often obtain contradictory conclusions. To make matters worse, PO Box 90153, 5000 LE Tilburg, The Netherlands. Tel.: +31 13 466 3092. estimation results are often not robust to small changes in E-mail address: [email protected] (J.R. Magnus). model specification, making credible interpretations of the results 0304-4076/$ – see front matter ' 2009 Elsevier B.V. All rights reserved. doi:10.1016/j.jeconom.2009.07.004 140 J.R. Magnus et al. / Journal of Econometrics 154 (2010) 139–153 hazardous. A proper treatment of model uncertainty is clearly the model selection procedure is completely separated from the important. estimation procedure. What is reported are therefore conditional One such treatment is model averaging, where the aim of the in- estimates, but the researcher acts as if they are unconditional vestigator is not to find the best possible model, but rather to find estimates. This problem is known as pretesting. the best possible estimates. Each model contributes information In order to combine model selection and estimation, the about the parameters of interest, and all these pieces of informa- Bayesian method offers a natural framework. The basic equations tion are combined taking into account the trust we have in each of BMA were first presented by Leamer (1978, Sections 4.4–4.6), model, based on our prior beliefs and on the data. who proposed Bayesian averaging of Bayesian estimates. In the In a sense, all estimation procedures are model averaging context of growth econometrics, BMA was first applied by algorithms, although possibly extreme or limiting cases. Our Fernández et al. (2001a) and Brock and Durlauf (2001). BMA framework is the linear regression model is flexible with respect to the size and exact specification of a model and it does not require the a priori selection of any model. y D X β C X β C " D Xβ C "; " ∼ N.0; σ 2/; (1) 1 1 2 2 Inference is based on a weighted average over all models. The idea where y (n × 1) is the vector of observations, X1 (n × k1) and of Bayesian averaging of classical estimates was first proposed in X2 (n × k2) are matrices of nonrandom regressors, " is a random Raftery (1995) and later by Sala-i-Martin et al. (2004). In growth vector of unobservable disturbances, and β1 and β2 are unknown econometrics, BMA has proved useful, and recent applications parameter vectors. We assume that k1 ≥ 1, k2 ≥ 0, k VD k1 C k2 ≤ include León-González and Montolio (2004), Sala-i-Martin et al. n − 1, that X VD .X1 V X2/ has full column-rank, and that the (2004) and Masanjala and Papageorgiou (2007). Recently, interest 2 disturbances ."1;:::;"n/ are i.i.d. N.0; σ /. is growing in different aspects of growth empirics, such as The reason for distinguishing between X1 and X2 is that X1 nonlinearities, parameter heterogeneity, and endogeneity. BMA contains explanatory variables which we want in the model on is also applied in other areas of economics; see for example theoretical or other grounds (irrespective of the found t-ratios Tsangarides et al. (2004), Crespo-Cuaresma and Doppelhofer of the β1-parameters), while X2 contains additional explanatory (2007), Eicher et al. (2007a,c), Masanjala and Papageorgiou (2008) variables of which we are less certain. The columns of X1 are called and Prüfer and Tondl (2008). In short, BMA has become an 1 `focus' regressors, and the columns of X2 `auxiliary' regressors. important technique. There are k components of β , and a different model arises 2 2 There are, however, two major problems with BMA. First, the whenever a different subset of the β 's is set equal to zero. If k D 0, 2 2 computational burden is very substantial. In fact, it is usually then no model selection takes place. If k D 1, then there are 2 impossible to get exact BMA estimates, in which case some two models to consider: the unrestricted and the restricted model. MCMC method must be applied, of which the Metropolis–Hastings If k D 2, there are four models: the unrestricted, two partially 2 algorithm is the most common. Second, Bayesian techniques work restricted (where one of the two β 's is zero), and the restricted 2 well when prior information is available, in which case they model. In general, there are 2k2 models to consider. We denote the guide us as to how this information should be combined with ith model by Mi, which we write as information from the data. But when no prior information is y D X1β1 C X2iβ2i C "; available and nevertheless informative priors need to be specified (as is the case with BMA), then we need to reflect on the meaning where X2i denotes an n × k2i matrix containing a subset of k2i and impact of these priors. columns of X2, and β2i denotes the corresponding k2i ×1 subvector In addition to these two problems, there are some further of β2. We have of course 0 ≤ k2i ≤ k2. Model averaging estimation proceeds in two steps. In the first uncomfortable aspects to BMA. One is that BMA takes different step we ask how to estimate the parameters, conditional upon a priors for the same parameter depending on which submodel is selected model. In the second step we compute the estimator as considered. This was also noted by Hjort and Claeskens (2003), and a weighted average of these conditional estimators. There exist it is a little difficult to interpret. Another uncomfortable aspect is both Bayesian and non-Bayesian ideas about how to estimate and that — since exact BMA is computationally so demanding — it is how to find the weights. Our emphasis will be on the Bayesian very difficult to consider extensions, for example to nonspherical framework; for the non-Bayesian approach, see Claeskens and disturbances.2 Hjort (2003), Hjort and Claeskens (2003), Hansen (2007), and Liang Our proposed WALS method deals with all these problems. The et al.
Details
-
File Typepdf
-
Upload Time-
-
Content LanguagesEnglish
-
Upload UserAnonymous/Not logged-in
-
File Pages15 Page
-
File Size-