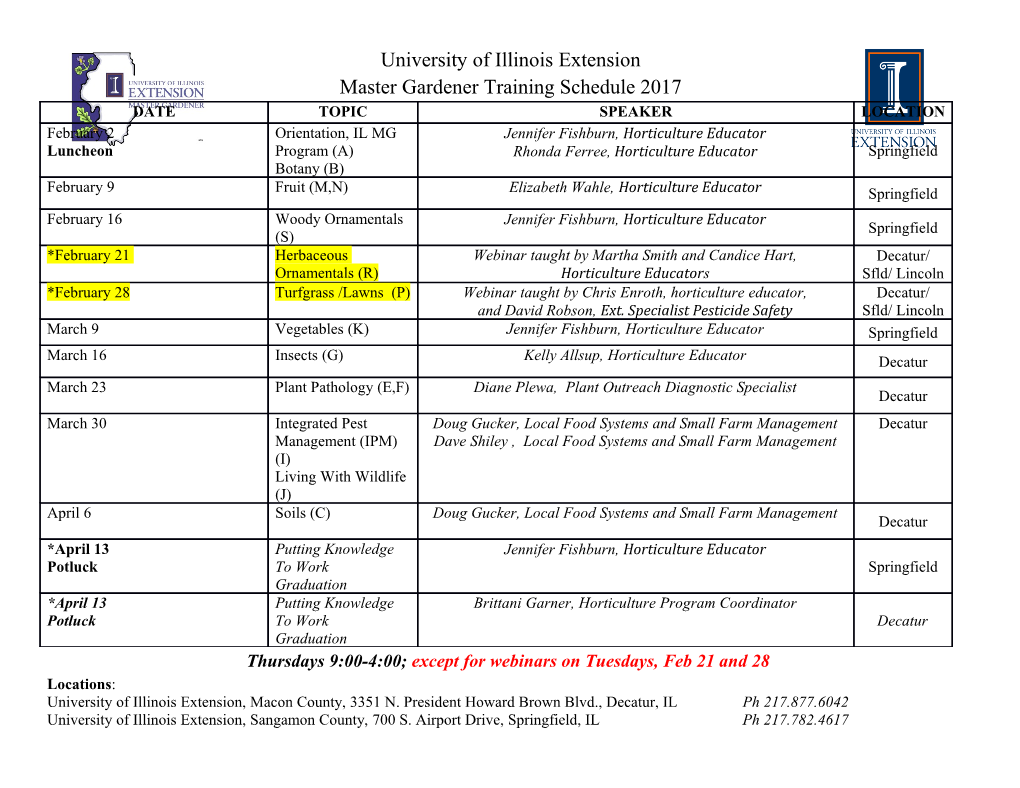
9/8/2016 CHE384, From Data to Decisions: Measurement, Kurtosis Uncertainty, Analysis, and Modeling • For any distribution, the kurtosis (sometimes Lecture 14 called the excess kurtosis) is defined as Testing for Kurtosis 3 (old notation = ) • For a unimodal, symmetric distribution, Chris A. Mack – a positive kurtosis means “heavy tails” and a more Adjunct Associate Professor peaked center compared to a normal distribution – a negative kurtosis means “light tails” and a more spread center compared to a normal distribution http://www.lithoguru.com/scientist/statistics/ © Chris Mack, 2016Data to Decisions 1 © Chris Mack, 2016Data to Decisions 2 Kurtosis Examples One Impact of Excess Kurtosis • For the Student’s t • For a normal distribution, the sample distribution, the variance will have an expected value of s2, excess kurtosis is and a variance of 6 2 4 1 for DF > 4 ( for DF ≤ 4 the kurtosis is infinite) • For a distribution with excess kurtosis • For a uniform 2 1 1 distribution, 1 2 © Chris Mack, 2016Data to Decisions 3 © Chris Mack, 2016Data to Decisions 4 Sample Kurtosis Sample Kurtosis • For a sample of size n, the sample kurtosis is • An unbiased estimator of the sample excess 1 kurtosis is ∑ ̅ 1 3 3 1 6 1 2 3 ∑ ̅ Standard Error: • For large n, the sampling distribution of 1 24 2 1 approaches Normal with mean 0 and variance 2 1 of 24/n 3 5 • For small samples, this estimator is biased D. N. Joanes and C. A. Gill, “Comparing Measures of Sample Skewness and Kurtosis”, The Statistician, 47(1),183–189 (1998). © Chris Mack, 2016Data to Decisions 5 © Chris Mack, 2016Data to Decisions 6 1 9/8/2016 Sample Kurtosis Test Jarque-Berra Test • Only perform the kurtosis test if the skewness • The tests for skewness and kurtosis can test fails to reject the null hypothesis be combined into one • Null hypothesis: g2 = 0 (Normal distribution) • Test statistic: is approximately / ~ 2 standard Normal for n > 20 – We generally perform a two-tailed test • If the test statistic / is beyond the • For example, the 95% (a = 0.05) critical critical z-value for our significance level we value for 2 is 5.99 and the 99% reject the null hypothesis that the distribution is critical value is 9.21 Normal © Chris Mack, 2016Data to Decisions 7 © Chris Mack, 2016Data to Decisions 8 Impact of Outliers Lecture 14: What have we learned? • Both the Skewness test and the Kurtosis • How is kurtosis defined? test are very sensitive outlier detectors • For positive excess Kurtosis, what is the – One outlier will make the distribution appear shape of the pdf? skewed • Be able to test a sample data set for – Two symmetric outliers will make the tails excess kurtosis. What test statistic is appear heavy used? What is its sampling distribution? • More on outlier detection in the next lectures © Chris Mack, 2016Data to Decisions 9 © Chris Mack, 2016Data to Decisions 10 2.
Details
-
File Typepdf
-
Upload Time-
-
Content LanguagesEnglish
-
Upload UserAnonymous/Not logged-in
-
File Pages2 Page
-
File Size-