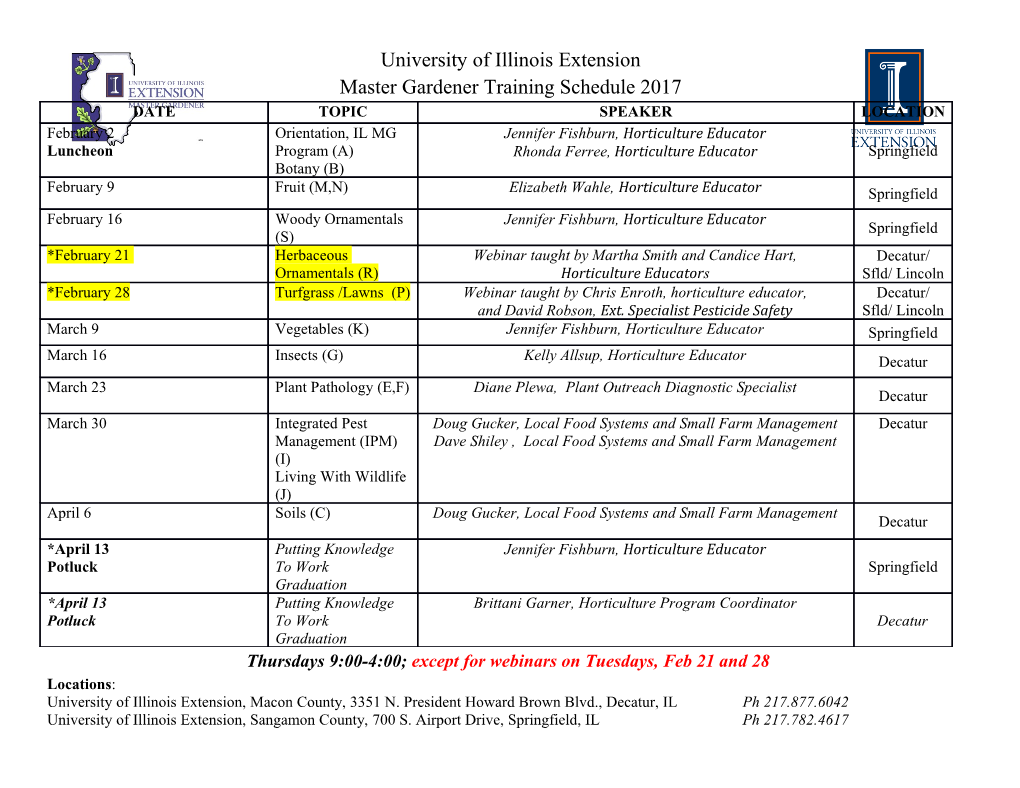
Applications of the Shannon-Hartley Theorem to Data Streams and Sparse Recovery Eric Price David P. Woodruff MIT IBM Almaden Abstract—The Shannon-Hartley theorem bounds the is also needed for network aggregation, which usually maximum rate at which information can be transmitted follows a bottom-up approach [18]: given a routing tree over a Gaussian channel in terms of the ratio of the signal where the nodes represent sensors, starting from the to noise power. We show two unexpected applications of this theorem in computer science: (1) we give a much simpler leaves the aggregation propagates upwards to the root. proof of an Ω(n1−2=p) bound on the number of linear We refer to the rows of A as measurements. measurements required to approximate the p-th frequency Alon, Matias, and Szegedy [2] initiated the line of moment in a data stream, and show a new distribution work on frequency moments. There is a long line of which is hard for this problem, (2) we show that the number of measurements needed to solve the k-sparse upper bounds on the number of linear measurements; we recovery problem on an n-dimensional vector x with the refer the reader to the most recent works [3], [11] and the C-approximate `2=`2 guarantee is Ω(k log(n=k)= log C). references therein. Similarly, we refer the reader to the We complement this result with an almost matching ∗ most recent lower bounds [16], [22] and the references O(k log k log(n=k)= log C) upper bound. therein. The best upper and lower bounds for obtaining I. INTRODUCTION a (1 + )-approximation with probability at least 1 − δ have the form n1−2=p · poly(−1 log(nδ−1). Let S be a real-valued random variable with E[S2] = τ 2. Consider the random variable S + T , where T ∼ The existing lower bounds are rather involved, using N(0; σ2) is additive white Gaussian noise of variance the direct sum paradigm for information complexity σ2. The Shannon-Hartley theorem states that [5]. Moreover, they apply to the number of bits rather than the number of linear measurements, and typically 2 1 τ do not provide an explicit distribution which is hard. I(S; S + T ) ≤ log 1 + ; 2 σ2 These issues can be resolved using techniques from [4], [20] and [15]. The resulting hard distribution is: choose where I(X; Y ) = h(X) − h(XjY ) is the mu- x 2 {−1; 0; 1gn uniformly at random, and then with tual information between X and Y , and h(X) = probability 1=2, replace a random coordinate x of x − R f(x) log f(x)dx is the differential entropy of a i X with a value in Θ(n1=p). F (x) changes by a constant random variable X with probability density function f. p factor in the two cases, and so the approximation algo- We show two unexpected applications of the Shannon- rithm must determine which case we are in. Hartley theorem in computer science, the first to estimat- ing frequency moments in a data stream, and the second We instead consider the following continuous dis- to approximating a vector by a sparse vector. tribution: choose x to be a random N(0;In) vector, i.e., a vector whose coordinates are independent stan- A. Sketching Frequency Moments dard normal random variables. With probability 1=2, In the data stream literature, a line of work has consid- replace a random coordinate xi of x with a value 1=p ered the problem of estimating the frequency moments in Θ(n ). The use of Gaussians instead of signs p Pn p n allows us to derive our lower bound almost immedi- Fp(x) = kxkp = i=1 jxij , where x 2 R and p ≥ 2. One usually wants a linear sketch, that is, we choose a ately from the Shannon-Hartley theorem. We obtain an m×n Ω(n1−2=p) bound on the number of linear measurements random matrix A 2 R from a certain distribution, for m n, and compute Ax, from which one can required for estimating Fp, matching known bounds up to poly(−1 log(Mnδ−1) factors. Our proof is much output a constant-factor approximation to Fp(x) with high probability. Linearity is crucial for distributed com- simpler than previous proofs. putation, formalized in the MUD (Massive Unordered Our new hard distribution may also more accurately Distributed) model [9]. In this model the vector x is model those signals x arising in practice, since it corre- split into pieces x1; : : : ; xr, each of which is handled by sponds to a signal with support 1 which is corrupted by a different machine. The machines individually compute independent Gaussian noise in each coordinate. Identify- Ax1; : : : ; Axr, and an aggregation function combines ing natural hard distributions has been studied for other these to compute Ax and estimate Fp(x). Linearity data stream problems, see, e.g., [19] and [17]. 0 0 B. Sparse Recovery r ≥ 2Gpn, then Bob sets Z = 1, else Bob sets Z = 0. 0 In the field of compressed sensing, a standard problem We thus have a Markov chain `; Z ! x ! y ! Z . is that of stable sparse recovery: we want a distribution If A works for any x with probability 1 − δ, as a m×n n distribution over A, then there is a specific A and random A of matrices A 2 R such that, for any x 2 R and with probability 1 − δ > 2=3 over A 2 A, there is an seed such that A, together with the associated estimation algorithm to recover x^ from Ax with procedure, succeeds with probability 1−δ over x drawn from the distribution described above. Let us fix this 0 kx^ − xkp ≤ (1 + ) min kx − x kp choice of A and associated random seed, so that Alice k−sparse x0 and Bob run deterministic algorithms. Let m be the for some > 0 and norm p. We call this a (1 + )- number of rows of A. We can assume the rows of A are approximate `p=`p recovery scheme with failure proba- orthonormal since this can be done in post-processing. bility δ. We will focus on the popular case of p = 2. Lemma 2: I(Z; Z0) = O(m=n1−2=p). For any constant δ > 0 and any satisfying = O(1) Proof: Let the rows of A be denoted v1; : : : ; vm. −1=2 and = Ω(n ), the optimal number of measurements Then we have that is Θ(k log(n=k)/). The upper bound is in [12], and the i 1=p 1=p i 0 lower bound is given by [1], [6], [14], [20]; see [20] for yi = hv ; xi = (4Gp) n · hv ; e`iZ + wi; a comparison of these works. 0 1=p 1=p One question is if the number of measurements can where wi ∼ N(0; 1). Define zi = (4Gp) n · i 0 be improved when the approximation factor C = 1 + hv ; e`iZ so yi = zi + wi. Then is very large (i.e. !(1)). In the limiting case of C = 1, 2 1 2=p i 2 corresponding to sparse recovery in the absence of noise, EZ;`[z ] = · (4Gpn) E`[(v ) ] i 2 ` it is known that O(k) measurements are sufficient [7]. 1 (4G n)2=p 1 (4G )2=p However, the intermediate regime has not been well = p = p = Θ(1=n1−2=p): 2 n 2 1−2=p studied. n 0 Using the Shannon-Hartley theorem, we prove an Hence, yi = zi + wi is a Gaussian channel with power 2 1−2=p Ω(k log(n=k)= log C) lower bound on the number of constraint E[zi ] = Θ(1=n ) and noise variance 0 2 measurements. We complement this with a novel sparse E[(wi) ] = 1. By the Shannon-Hartley theorem, recovery algorithm, which builds upon [12] and [13], 2 but is the first to obtain an improved bound for C > 1. 1 E[zi ] max I(zi; yi) ≤ log 1 + ∗ i 0 2 Our bound is O(k + k log(n=k) log k= log C), which v 2 E[(wi) ] ∗ matches our lower bound up to a log k factor. Because 1 1−2=p 1−2=p 1 = log 1 + Θ(1=n ) = Θ(1=n ): log(1 + ) ≈ , these results match the Θ( k log(n=k)) 2 results for 1. By the data processing inequality for Markov chains and Related work. Related lower bounds have appeared the chain rule for entropy, in a number of recent works, including [6], [14], [1], [21], and [10]. See [20] for a comparison. I(Z; Z0) ≤ I(z; y) = h(y) − h(yjz) II. LOWER BOUND FOR FREQUENCY MOMENTS = h(y) − h(y − zjz) X 0 0 0 This section is devoted to proving the following the- = h(y) − h(wijz; w1; : : : ; wi−1) orem: i X 0 X 0 Theorem 1: Any sketching algorithm for Fp up to a = h(y) − h(wi) ≤ h(yi) − h(wi) factor of (1 ± ) for < 1=2, which succeeds with i i X X probability 1 − δ for a sufficiently small constant δ > 0, = h(yi) − h(yijzi) = I(yi; zi) 1−2=p requires m = Ω(n ). i i p 1−2=p Let Gp = E[jXj ] where X ∼ N(0; 1). For constant ≤ O(m=n ): p, Gp is Θ(1). Consider the following communication game between p two players, Alice and Bob. Alice chooses a random Proof of Theorem 1: If Z = 1, then kxkp ≥ 4Gp·n, ` 2 [n] and associated standard unit vector e` = and so any (1 ± )-approximation is at least 2Gpn for n p (0;:::; 0; 1; 0;:::; 0) 2 R .
Details
-
File Typepdf
-
Upload Time-
-
Content LanguagesEnglish
-
Upload UserAnonymous/Not logged-in
-
File Pages5 Page
-
File Size-