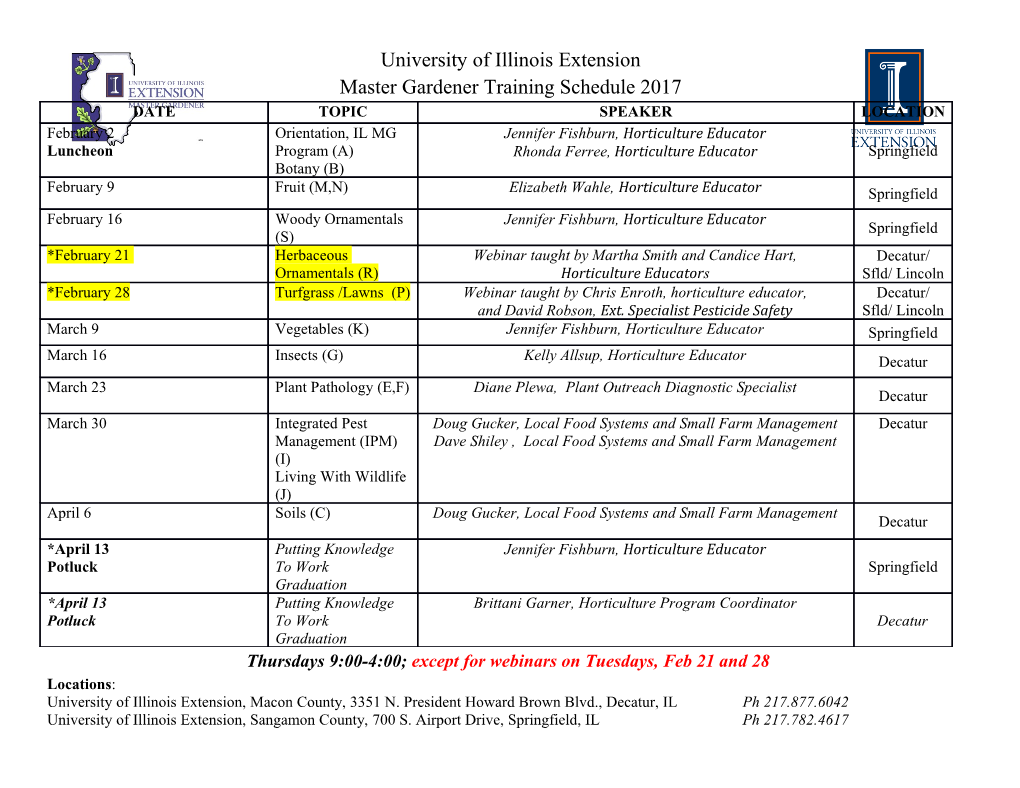
An Index of Oscar-Worthiness: Predicting the Academy Award for Best Picture∗ Andrew B. Bernard Tuck School of Business February 21, 2005 ∗Profuse thanks are due to Conor Dougherty and the staff at the Weekend Journal of the Wall Street Journal for asking whether this might work and providing all the data. As of the writing of this paper I had not seen any of the nominated films for 2004. Unfortunately, all errors remain mine alone. 1 1 Introduction The awarding of the Oscar for Best Picture probably has more to do with art than with science. However, once the five nominees have been selected, history and statistics provide a strong guide as to which film will take home the small golden statue. I use data from the last twenty years to identify whichmovieshadthegreatestchanceatwinningtheBestPictureaward and to make a prediction about the probability of winning for this year’s nominees. According to my model, The Aviator is a heavy favorite to take home the 2004 Best Picture award. In addition, I create a complete historical ranking for all the nominated films over the last twenty years, an index of so-called ‘Oscar-Worthiness’. This index helps us identify several types of movies: a) ‘Sure Things’ or films that would have won in almost any year, b) ‘Lucky Dogs’, movies that won by virtue of having weak competition, and c) ‘Doomed Gems’, movies thatwouldhavewoninalmostanyotheryearbuthadthemisfortuneto compete against a ‘Sure Thing’. Even by historical standards, The Aviator is a strong Oscar contender, with an Oscar-Worthiness Index among the top 15 nominated movies since 1984. The paper is laid out as follows: Section 2 presents a list of movie charac- teristics and their association with winning the Best Picture award; Section 3 presents the empirical model used to predict the Best Picture Award and gives the results of the prediction exercise for 2004; Section 4 introduces the Index of Oscar-Worthiness and presents ‘Sure Things’, ‘Lucky Dogs’, and ‘Doomed Gems’ over the last twenty years. Section 5 provides the requisite happy ending. 2 Potential Predictors To begin, I identify a list of characteristics of each film nominated for an Oscar overthelasttwentyyears.1 These potential explanatory variables include both performance measures, e.g. the number of Golden Globe awards, and attributes of the film, e.g. whether the lead rode a horse, etc. The complete list of variables is given below: Performance Measures • 1 Ideas for the variables were contributed by the WSJ staff. 2 — Number of Oscar Nominations — Number of Golden Globe Wins — Golden Globe for Best Picture Movie Characteristics • — Based on Novel or Play — Comedy2 — Set at Least 20 Years before Release Date — Deals with Real Incident or Person — Lead Character Meets Untimely Death — Five Hanky Tearjerker — Unlikely Love Story — Lead Actor/Actress Comes From a Commonwealth Country — Has a Sports Theme — Lead Character gets on a Horse — Happy but Poor — Lead is Disabled — Lead Character is a Genius — Film Includes War Plot Line — Includes Action Outside of North America or Europe The list of potential explanatory variables is not intended to be exhaustive and surely misses important elements in the Oscar selection process. Table 1 reports the differences for these characteristics across winners and losers for the last twenty years. Quite a few of the variables show statistical differences across the two groups. Perhaps not surprisingly, winners have more total Oscar nominations, 10.5, than losers, 6.7. In addition, Best Picture winners aremorelikelytohavewonGoldenGlobeawardsinthatsameyear:on average, winners take home 2.9 Golden Globes while losers end up with just 2 The designation of a movie as a comedy was based on its primary category on R Netflix°. For example, Shakespeare in Love is classified as a romance. 3 Table 1: Differences Between Best Picture Winners and Losers, 1983-2003 Losers Winners Significant Number of Oscar Nominations 6.7 10.5 9 Number of Golden Globe Wins 0.9 2.9 9 Golden Globe for Best Picture 20% 85% 9 Based on Novel or Play 41% 45% Set at Least 20 Years 55% 70% Deals with Real Incident or Person 30% 30% Lead Meets Untimely Death 33% 30% Five Hanky Tearjerker 20% 10% Unlikely Love Story 28% 35% Lead Comes From A Commonwealth Country 35% 60% 9 Has a Sports Theme 4% 5% Lead Gets on a Horse 10% 30% 9 Happy but Poor 11% 15% Lead is Disabled 10% 10% Lead is a Genius 5% 20% 9 Includes War Plot Line 18% 20% Includes Action Outside of North America or Europe 19% 35% Comedy 15% 0% 9 The first two columns give the mean (or percentage) of the variable for winners and losers. The third column indicates that the differences in the variable between winners and losers were statistically significant at the 5% level. under one Golden Globe apiece. Similarly, movies that go on to win the Academy Award for Best Picture are substantially more likely to have won aGoldenGlobeforBestPicture.3 Turning to the characteristics of the movies themselves, I find fewer sta- tistically significant variables. However, there are some surprises. Movies whose lead comes from a Commonwealth country, i.e. an English-speaking country such as England, Australia or New Zealand, are almost twice as likely to end up winning. Having the lead character get on a horse is also associated with Oscar success; 30 percent of the Best Picture winners saw the lead get on a horse while only 10 percent of the losers had mounted leads. It also appears to help if the leading character has above average intelligence; 20 percent of winners had ‘geniuses’ for a lead character while only 5 percent 3 There are two Golden Globe awards for Best Picture, one for Drama and one for Musical or Comedy. 4 of the losers did.4 Finally, the ultimate predictor of failure among nomi- nated pictures is the designation as a comedy. None of the ten nominated comedies has won the Best Picture Oscar over the last twenty years.5 3 A Model for Best Picture Based on the results in Table 1, I assemble a short list of likely candidates for Best Picture predictors. I then proceed to assess the predictive power of combinations of the variables. The basic empirical technique employed in the paper is commonly referred to as a probit model. The simplest probit model attempts to estimate an unobserved variable, in this case the probability of winning the Best Picture award, by relating two observed phenomena: whether the picture won or lost and a characteristic of the movie.6 Pr(Winning)=f (Variable) . Results from these simple probits (the log-likelihoods) can be examined to determine which variables are most strongly correlated with winning over the last twenty years. Using these results, I then run a probit on larger groups of variables, i.e. a probit with several explanatory factors. 3.1 The Simplest Model That Works Well From the earlier results, I narrow down the list of potential predictors to the group of seven significant characteristics, i.e. those marked with a X in Table 1. As expected, the number of overall Oscar nominations does a reasonable job in predicting Oscar winners. By itself, total nominations correctly identifies a clear winner in 14 of the last twenty years.7 Similarly, the number of Golden Globes correctly predicts the winner in 13 of 20 years.8 4 However, these results do not suggest that any film featuring an English genius who rides a horse is a shoe-in for Best Picture. 5 Annie Hall won the Best Picture in 1977, however, that film is not designated as a R comedy by Netflix°. 6 Formally probits are a form of regression that are described in every introductory econometrics text. 7 In four more years, the eventual winner is tied for the most nominations. 8 The number of Golden Globes incorrectly identifies the winner 6 times and in one year there is a tie. 5 Table 2: The Effects of Nominations and Golden Globes on Winning, 1984- 2003 Marginal Effect Standard Error Z-Stat Number of Oscar Nominations 0.045 0.019 3.18 Number of Golden Globe Wins 0.102 0.040 3.73 Number of Correct Best Picture Predictions 18 out of 20 90% The first column gives the marginal increase in the probability of winning the Best Picture Oscar for an extra Oscar nomination or Golden Globe for the average movie. The second column reports the standard error and the third column gives the z-score. The probit was run on the sample of non-comedy nominated movies as Comedy perfectly predicts losing in sample. Both variables are significant at the 1% level. However, using groups of variables I am able to substantially improve the predictive power of the simple model. Among these seven variables, one group of three variables far outper- forms any other combination in predicting the Best Picture winner over the last twenty years. These three predictors are: Total Oscar Nominations, Golden Globes Won, and Comedy. No other variable on the list improves the explanatory power of the model once these three predictors are included. The resulting estimating equation is Pr(Winning)=f (α Nominations + β Golden Globes + θ Comedy + εit) . · · · To estimate the marginal effects of each variable on the probability of winning, I run a probit on Nominations and Golden Globes excluding the sample of comedies. Since no Comedy has won Best Picture in the last twenty years, the Comedy variable is perfectly correlated with losing. The results of the probit are summarized in Table 2. Foreachmovie,themodelgivesanoverallprobabilityofbeingawinner across the twenty years. To turn this into a prediction for each year, I examine the probability of winning for each of the five nominees in every year.
Details
-
File Typepdf
-
Upload Time-
-
Content LanguagesEnglish
-
Upload UserAnonymous/Not logged-in
-
File Pages12 Page
-
File Size-