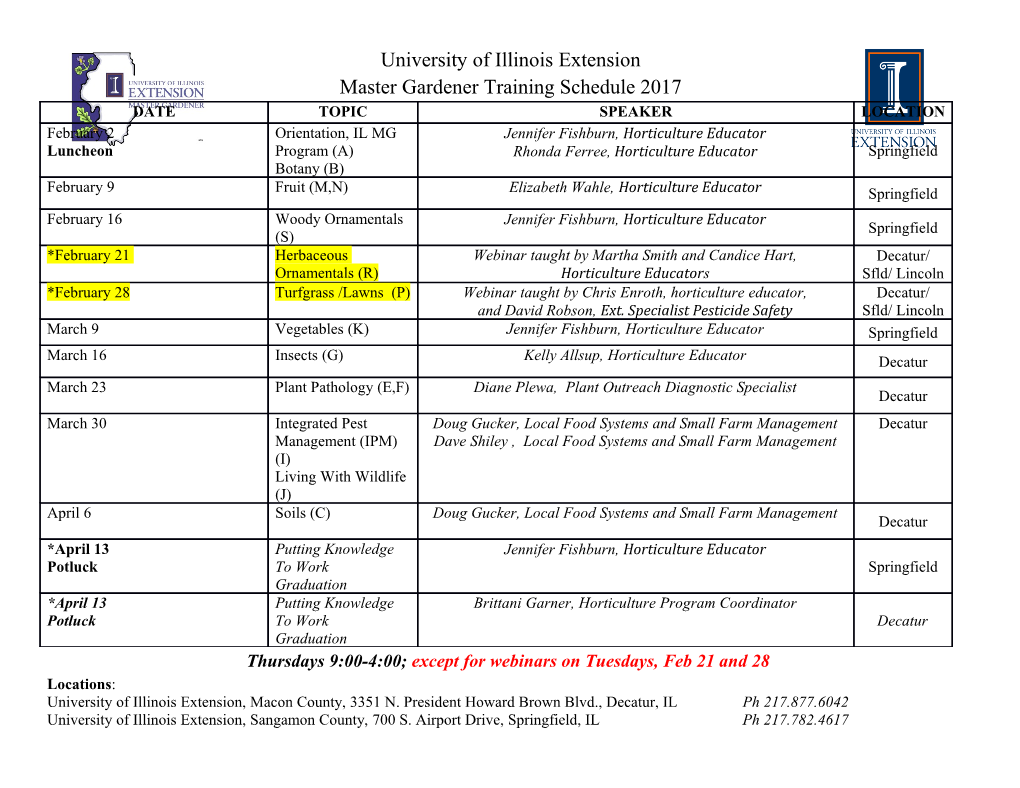
Automatically Tuning Collective Communication for One-Sided Programming Models by Rajesh Nishtala A dissertation submitted in partial satisfaction of the requirements for the degree of Doctor of Philosophy in Computer Science in the Graduate Division of the University of California, Berkeley Committee in charge: Professor Katherine A. Yelick, Chair Professor James W. Demmel Professor Panayiotis Papadopoulos Fall 2009 Automatically Tuning Collective Communication for One-Sided Programming Models Copyright 2009 by Rajesh Nishtala 1 Abstract Automatically Tuning Collective Communication for One-Sided Programming Models by Rajesh Nishtala Doctor of Philosophy in Computer Science University of California, Berkeley Professor Katherine A. Yelick, Chair Technology trends suggest that future machines will rely on parallelism to meet increasing performance requirements. To aid in programmer productivity and application performance, many parallel programming models provide communication building blocks called collective communication. These operations, such as Broadcast, Scatter, Gather, and Reduce, ab- stract common global data movement patterns behind a simple library interface allowing the hardware and runtime system to optimize them for performance and scalability. We consider the problem of optimizing collective communication in Partitioned Global Address Space (PGAS) languages. Rooted in traditional shared memory programming models, they deliver the benefits of sophisticated distributed data structures using language extensions and one-sided communication. One-sided communication allows one processor to directly read and write memory associated with another. Many popular PGAS language implementations share a common runtime system called GASNet for implementing such communication. To provide a highly scalable platform for our work, we present a new implementation of GASNet for the IBM BlueGene/P, allowing GASNet to scale to tens of thousands of processors. We demonstrate that PGAS languages are highly scalable and that the one-sided com- munication within them is an efficient and convenient platform for collective communication. We show how to use one-sided communication to achieve 3× improvements in the latency and throughput of the collectives over standard message passing implementations. Using a 3D FFT as a representative communication bound benchmark, for example, we see a 17% increase in performance on 32,768 cores of the BlueGene/P and a 1.5× improvement on 1024 cores of the CrayXT4. We also show how the automatically tuned collectives can deliver more than an order of magnitude in performance over existing implementations on shared memory platforms. There is no obvious best algorithm that serves all machines and usage patterns demon- strating the need for tuning and we thus build an automatic tuning system in GASNet that optimizes the collectives for a variety of large scale supercomputers and novel multicore architectures. To understand the large search space, we construct analytic performance 2 models use them to minimize the overhead of autotuning. We demonstrate that autotun- ing is an effective approach to addressing performance optimizations on complex parallel systems. i Dedicated to Rakhee, Amma, and Nanna for all their love and encouragement ii Contents List of Figures v 1 Introduction 1 1.1 Related Work . 3 1.1.1 Automatically Tuning MPI Collective Communication . 3 1.2 Contributions . 4 1.3 Outline . 5 2 Experimental Platforms 7 2.1 Processor Cores . 8 2.2 Nodes . 8 2.2.1 Node Architectures . 8 2.2.2 Remote Direct Memory Access . 11 2.3 Interconnection Networks . 12 2.3.1 CLOS Networks . 12 2.3.2 Torus Networks . 17 2.4 Summary . 17 3 One-Sided Communication Models 19 3.1 Partitioned Global Address Space Languages . 19 3.1.1 UPC . 20 3.1.2 One-sided Communication . 22 3.2 GASNet . 23 3.2.1 GASNet on top of the BlueGene/P . 23 3.2.2 Active Messages . 28 4 Collective Communication 29 4.1 The Operations . 29 4.1.1 Why Are They Important? . 30 4.2 Implications of One-Sided communication for Collectives . 31 4.2.1 Global Address Space and Synchronization . 31 4.2.2 Current Set of Synchronization Flags . 32 4.2.3 Synchronization Flags: Arguments for and Against . 33 4.2.4 Optimizing the Synchronization and Collective Together . 34 iii 4.3 Collectives Used in Applications . 35 5 Rooted Collectives for Distributed Memory 38 5.1 Broadcast . 39 5.1.1 Leveraging Shared Memory . 39 5.1.2 Trees . 40 5.1.3 Address Modes . 49 5.1.4 Data Transfer . 51 5.1.5 Nonblocking Collectives . 52 5.1.6 Hardware Collectives . 55 5.1.7 Comparison with MPI . 56 5.2 Other Rooted Collectives . 58 5.2.1 Scratch Space . 58 5.2.2 Scatter . 59 5.2.3 Gather . 60 5.2.4 Reduce . 62 5.3 Performance Models . 64 5.3.1 Scatter . 66 5.3.2 Gather . 69 5.3.3 Broadcast . 69 5.3.4 Reduce . 72 5.4 Application Examples . 72 5.4.1 Dense Matrix Multiplication . 74 5.4.2 Dense Cholesky Factorization . 76 5.5 Summary . 78 6 Non-Rooted Collectives for Distributed Memory 80 6.1 Exchange . 80 6.1.1 Performance Model . 82 6.1.2 Nonblocking Collective Performance . 86 6.2 Gather-to-All . 86 6.2.1 Performance Model . 89 6.2.2 Nonblocking Collective Performance . 89 6.3 Application Example: 3D FFT . 90 6.3.1 Packed Slabs . 92 6.3.2 Slabs . 94 6.3.3 Summary . 94 6.3.4 Performance Results . 95 6.4 Summary . 99 7 Collectives for Shared Memory Systems 101 7.1 Non-rooted Collective: Barrier . 102 7.2 Rooted Collectives . 106 7.2.1 Reduce . 106 iv 7.2.2 Other Rooted Collectives . 110 7.3 Application Example: Sparse Conjugate Gradient . 113 7.4 Summary . 115 8 Software Architecture of the Automatic Tuner 117 8.1 Related Work . 118 8.2 Software Architecture . 119 8.2.1 Algorithm Index . 119 8.2.2 Phases of the Automatic Tuner . 120 8.3 Collective Tuning . 122 8.3.1 Factors that Influence Performance . 122 8.3.2 Offline Tuning . 123 8.3.3 Online Tuning . 124 8.3.4 Performance Models . 125 8.4 Summary . 129 9 Teams 132 9.1 Thread-Centric Collectives . 132 9.1.1 Similarities and Differences with MPI . 133 9.2 Data-Centric Collectives . 134 9.2.1 Proposed Collective Model . 135 9.2.2 An Example Interface . 135 9.2.3 Application Examples . 138 9.3 Automatic Tuning with Teams . 142 9.3.1 Current Status . 144 10 Conclusion 146 Bibliography 150 v List of Figures 2.1 Sun Constellation Node Architecture . 9 2.2 Cray XT4 Node Architecture . 10 2.3 Cray XT5 Node Architecture . 10 2.4 IBM BlueGene/P Node Architecture . 11 2.5 Example Hierarchies with 4 port switches . 13 2.6 Example 16-node 5-stage CLOS Network . 13 2.7 Sun Constellation System Architecture . 15 2.8 Example 8x8 2D torus Network . 16 3.1 UPC Pointer Example . 21 3.2 Roundtrip Latency Comparison on the IBM BlueGene/P . 26 3.3 Flood Bandwidth Comparison on the IBM BlueGene/P . 26 4.1 Comparison of Loose and Strict.
Details
-
File Typepdf
-
Upload Time-
-
Content LanguagesEnglish
-
Upload UserAnonymous/Not logged-in
-
File Pages176 Page
-
File Size-