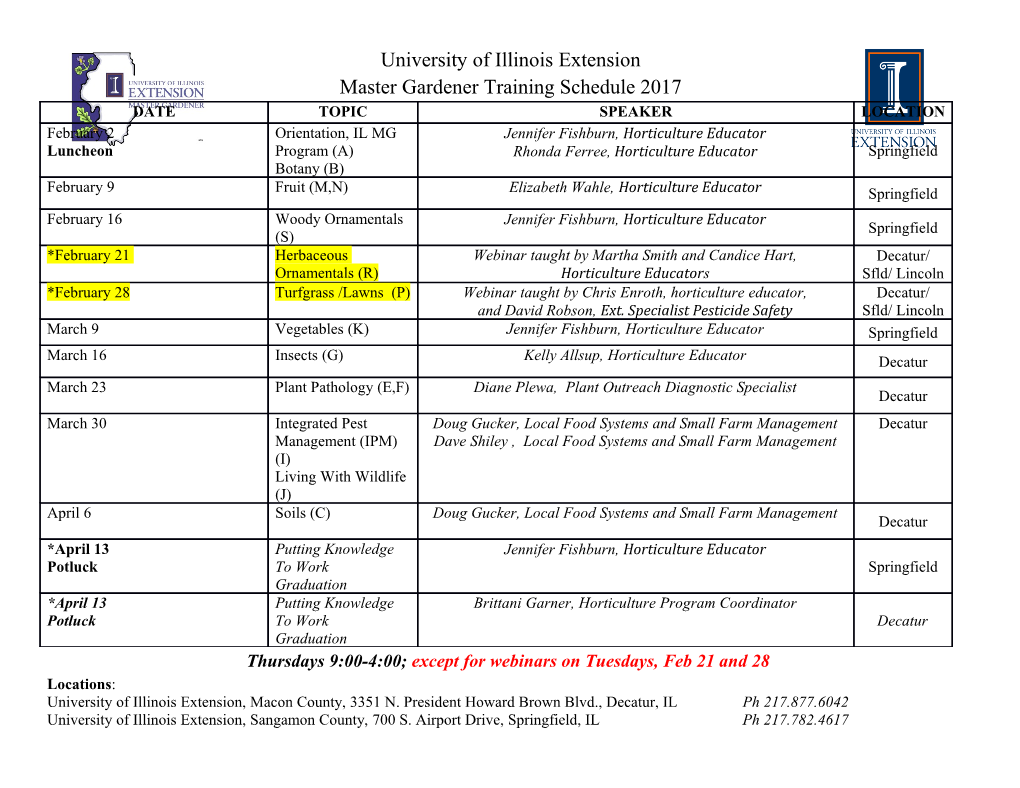
Towards edge-caching for image recognition Utsav Drolia*, Katherine Guoy, Jiaqi Tan*, Rajeev Gandhi*, Priya Narasimhan* *Carnegie Mellon University, yBell Labs, Nokia [email protected], [email protected], [email protected], [email protected], [email protected] Abstract—With the available sensors on mobile devices and Currently, such services and applications rely on cloud their improved CPU and storage capability, users expect their computing. These services are compute- and data- intensive devices to recognize the surrounding environment and to provide since image data needs to be processed to make sense of it, relevant information and/or content automatically and immedi- ately. For such classes of real-time applications, user perception e.g. a captured image has to be recognized as a specific person. of performance is key. To enable a truly seamless experience for Cloud computing enables running these intensive services the user, responses to requests need to be provided with minimal on powerful servers and allows mobile devices to use them user-perceived latency. remotely. Devices capture the data and upload it to the remote Current state-of-the-art systems for these applications require servers, where the data is processed, and then the results are offloading requests and data to the cloud. This paper proposes an approach to allow users’ devices and their onboard applications returned to the devices. This network-based interaction already to leverage resources closer to home, i.e., resources at the edge of induces latency and the ever-increasing number of mobile the network. We propose to use edge-servers as specialized caches devices and other Internet-capable things will further aggravate for image-recognition applications. We develop a detailed formula this model of computing. Such large numbers of devices, for the expected latency for such a cache that incorporates the especially in high densities, can cause network congestion in effects of recognition algorithms’ computation time and accuracy. We show that, counter-intuitively, large cache sizes can lead to the Internet backbone, and will inundate remote servers with higher latencies. To the best of our knowledge, this is the first a deluge of data. This will increase user-perceived latency as work that models edge-servers as caches for compute-intensive user-requests fight for network and compute resources. The recognition applications. centralized model of cloud computing cannot satisfy the low- latency requirements of such applications for the growing I. INTRODUCTION number of users. With the large number of sensors available on mobile One proposed approach towards meeting this challenge has devices and their improved CPU and storage capability, users been to place more compute resources at the edge of the expect their devices and applications to be smarter, i.e., recog- network, e.g. fog computing [9] and cloudlets [23], both sug- nize the surrounding environment and to provide relevant in- gest placing and utilizing compute resources at the edge, near formation and/or content automatically and immediately [17], the user, alongside last-mile network elements. It is proposed [7]. Users need a more seamless way of fetching information, that computation can be offloaded from users’ devices to and are moving away from typing text into devices. Vision- these edge-resources instead of offloading to the cloud. This based applications help users augment their understanding of would possibly reduce the expected end-to-end latency for the physical world by querying it through the camera(s) on applications. their mobile devices. Applications such as face recognition, Our work proposes an alternative in which we use an edge- augmented reality, place recognition [6], object recognition, server as a “cache with compute resources”. Using a caching vision-based indoor localization, all fall under this category. model gives us an established framework and parameters to There are a number of mobile applications available which reason about the performance of the system. This model makes do one or more of these [3], [1], [5]. Specialized augmented the edge-server transparent to the client, and self-managed - reality hardware is also available to the public now, such as administrators do not need to place content on it manually. The Google’s Glass [2] and Microsoft’s Hololens [4], to augment key insight lies in the fact that an edge-server serves a limited their vision of their surroundings. physical area, dictated by the network element it is attached to, This class of applications has a real-time nature - user and thus experiences spatiotemporal locality in requests and perception of performance is of primary importance; users data from users. It has already been established that document will find delays unacceptable and will cease using the ap- retrieval systems over the Internet, i.e. the World Wide Web plication when that happens. We want to enable a truly (WWW), can reap extensive benefits with caches at the edge seamless experience for the users by providing instantaneous [10] by leveraging locality in requests. Recognition applica- responses to user’s requests, with minimal user-perceived tions are similar to such retrieval systems and our proposed latency. Moreover, with devices like Glass and Hololens, users cache for recognition can accelerate applications transparently will not make explicit requests - objects will have to be by leveraging locality as well. In fact, we believe, recognition recognized in the stream of images captured while the user applications will benefit even more from caching, since many is looking at something. This further makes the case for real- users will be making requests related to the same physical time performance of vision-based recognition. objects in the area. The challenge is that, unlike requests in the WWW that use identifiers that deterministically map It can contain features from more than one image of each to specific content being requested, e.g. Uniform Resource object. The more objects one wants to recognize, the bigger Identifier (URI), requests in recognition applications are not this database will be. The matching procedure boils down deterministic. The object in the image needs to be recognized to finding the nearest-neighbor feature in the database for before the related content can be fetched. This is a compute- each feature of the input image. To accelerate this process, intensive task. This property has direct implications on how approximate nearest-neighbor searches have been proposed, an edge-cache for recognition is designed. such as Locality Sensitive Hashing [13] and kd-trees [24]. In this work we develop a detailed model for expected Best match selection. Once the closest features are found, they latency for a cloud-backed recognition cache. We incorporate are analyzed to predict the object contained in the request the effects of computation time and recognition accuracy into image. The object that has the most feature-matches with the the model. The intention is that a detailed model can help in input image, is chosen. This can be followed by geometric understanding tradeoffs and be used for prediction purposes verification for confirmation. in the future, to dynamically adjust the cache. As a first step This high level procedure, of extraction, matching with an towards that goal, we show the impact of changing the cache object-features set and verification, is common across vision- size on image-recognition applications. To the best of our based recognition algorithms [25]. knowledge, this is the first work that models edge-servers These stages for mobile image-recognition applications are as caches for compute-intensive recognition applications and typically carried out in the cloud. As shown in Figure 1, shows how offline analysis can help decide important model the image is captured by the mobile device, uploaded over a parameters. We do not propose any new computer vision or wireless network and sent across the Internet to the cloud. The image-recognition algorithms themselves. Instead we show image-recognition procedures are then carried out in the cloud, how we can take existing algorithms and applications, and and the response is returned to the device. These responses are use edge-resources effectively to reduce their user-perceived typically of some form of information or content. latency. Also, our approach does not involve the users’ mobile In this architecture, overall latency consists of two main devices. Instead we address the interaction between edge- factors: (a) Network latency, and (b) Compute time. servers and backend servers (a.k.a the cloud). (a) Network Latency: Applications incur this latency since In the next section we provide background on mobile image- they need to upload significant amounts of data to the recognition and edge-computing. In Section III we model the cloud, over the Internet, for each recognition request. edge-server as an image-recognition cache. We then discuss This latency can be reduced if the distance between the future work in Section IV. Section V presents related work, computing entity, currently the cloud, and the mobile and we conclude in Section VI. device is reduced. II. BACKGROUND (b) Compute Time: Image recognition algorithms are com- pute intensive. The main contributor to latency is the image-recognition.pdf matching of request-image features against the object- features set. Moreover, the size of this set has a direct impact on the latency. For a large number of objects, the computation time can lead to high latency and hence a poor user experience. B. Web Caching Fig. 1: Example architecture for mobile image-recognition Caching at the edge is a widely used technique for reduc- applications ing user-perceived latency when retrieving information from across the World Wide Web. This is not compute-intensive - users’ requests contain the specific identifier for the content A. Mobile Image Recognition they want to view, e.g.
Details
-
File Typepdf
-
Upload Time-
-
Content LanguagesEnglish
-
Upload UserAnonymous/Not logged-in
-
File Pages6 Page
-
File Size-