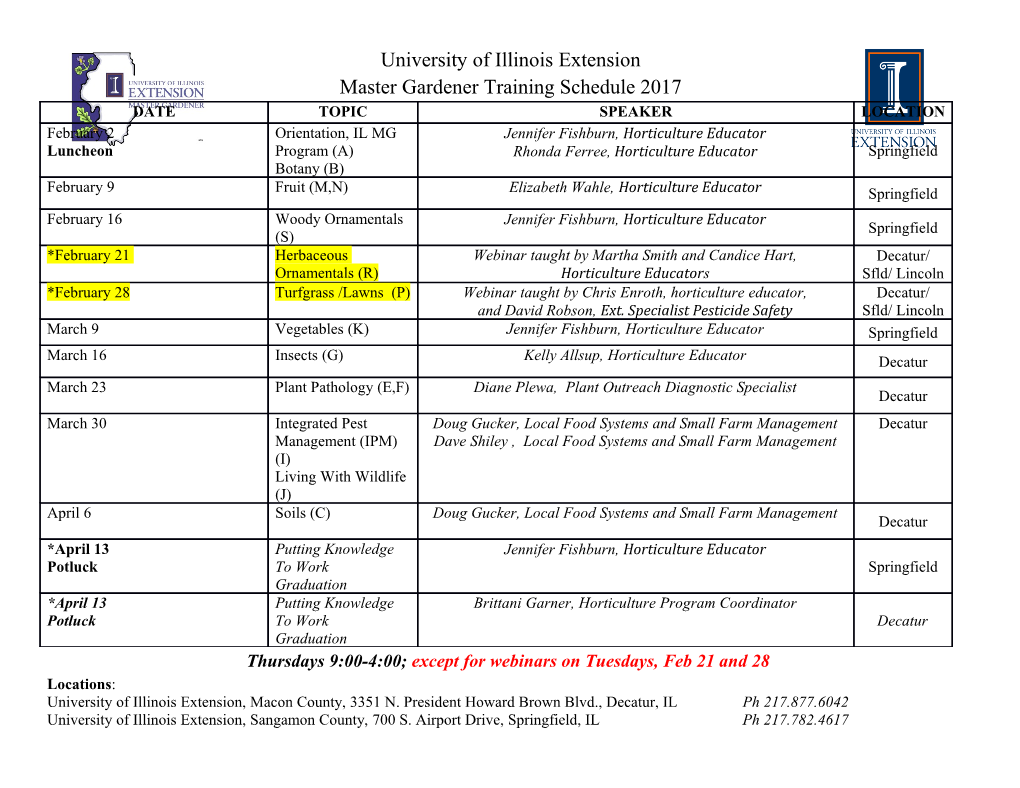
Semantic Web 0 (0) 1 1 IOS Press 1 1 2 2 3 3 4 Systematic Performance Analysis of 4 5 5 6 Distributed SPARQL Query Answering Using 6 7 7 8 8 9 Spark-SQL 9 10 10 * 11 Mohamed Ragab , Sadiq Eyvazov, Riccardo Tommasini and Sherif Sakr 11 12 Data Systems Group, Tartu University, Tartu, Estonia 12 13 E-mail: fi[email protected] 13 14 14 15 15 16 16 17 17 Abstract. Recently, a wide range of Web applications utilize vast RDF knowledge bases (e.g. DBPedia, Uniprot, and Probase), 18 18 and use the SPARQL query language. The continuous growth of these knowledge bases led to the investigation of new paradigms 19 19 and technologies for storing, accessing, and querying RDF data. In practice, modern big data systems like Apache Spark can 20 handle large data repositories. However, their application in the Semantic Web context is still limited. One possible reason is that 20 21 such frameworks are not tailored for dealing with graph data models like RDF. In this paper, we present a systematic evaluation of 21 22 the performance of SparkSQL engine for processing SPARQL queries. We configured the experiments using three relevant RDF 22 23 relational schemas, and two different storage backends, namely, Hive, and HDFS. In addition, we show the impact of using three 23 24 different RDF-based partitioning techniques with our relational scenario. Moreover, we discuss the results of our experiments 24 25 showing interesting insights about the impact of different configuration combinations. 25 26 26 Keywords: Large RDF Graphs, SPARQL, Apache Spark, Spark-SQL, RDF Relational Schema, RDF Partitioning 27 27 28 28 29 29 30 30 1. Introduction data processing and several approaches exist for repre- 31 31 senting the RDF data as relations [6–8]. 32 32 33 The Linked Data initiative is fostering the adop- To the best of our knowledge, a systematic analysis 33 34 tion of semantic technologies like never before [1, 2]. of the performance of Big Data frameworks when an- 34 35 Vast Resource Description Framework (RDF) datasets swering SPARQL Protocol and RDF Query Language 35 36 (e.g. DBPedia, Uniprot, and Probase) are now publicly (SPARQL) queries is still missing. Our research work 36 37 available, and the challenges of storing, managing, and focuses on filling this gap. In particular, we focus on 37 38 querying large RDF datasets are getting popular. Apache Spark that, with the Spark SQL engine, is the 38 39 In this regards, the scalability of native triplestores de-facto standards for processing large datasets. 39 40 like Apache Jena, RDF4J, and RDF-3X is bound In the first phase of our work [9], we presented 40 41 by a centralized architecture. Thus, the Semantic a systematic analysis of the performance of Spark- 41 42 Web community is investigating how to leverage big SQL on a centralized single-machine. In particular, 42 43 data processing frameworks like Apache Spark [3] we measured the execution time required to answer 43 44 to achieve better performance when processing large SPARQL queries. In our evaluation we considered: 44 45 RDF datasets [4, 5]. (i) alternative relational schemas for RDF, i.e., Single 45 46 In fact, despite big data frameworks are not tailored Statement Tables (ST), Vertical Tables (VT), and Prop- 46 47 to perform native RDF processing, they were success- erty Tables (PT); (ii) various storage backends, i.e., 47 48 PostgreSQL Hive HDFS 48 fully used to build engines for large-scale relational , , and , and (iii) and differ- 49 ent data formats (e.g. CSV, Avro, Parquet, ORC). 49 50 In this paper, we present the second phase of our 50 51 *Corresponding author. E-mail: fi[email protected]. investigation. Our experiments include larger dataset 51 1570-0844/0-1900/$35.00 c 0 – IOS Press and the authors. All rights reserved 2 Mohamed Ragab et al. / Systematic Performance Analysis of Distributed SPARQL Query Answering Using Spark-SQL 1 than before, in a distributed environment in presence of bug file format; (ii) Parquet1, which stores the data in 1 2 data partitioning. In particular, we evaluate the impact a nested data structure and a flat columnar format that 2 3 of three different RDF-based partitioning techniques supports compression; (iii) Avro2, which contains data 3 4 (i.e., Subject-based, Predicate-based, and Horizontal serialized in a compact binary format and schema in 4 5 partitioning) on our relational data. An additional con- JSON format. (iv) Optimized Row Columnar (ORC 3), 5 6 tributions of the current paper is a deeper and prescrip- which provides a highly efficient way to store and pro- 6 7 tive analysis of Spark SQL performance. Hence, in- cess Hive data. 7 8 spired by the work in [10], we analyze the experiments Last but not least, DataFrames are a convenient 8 9 results in detail and provide a framework for deciding programming abstraction that adds to the flexibil- 9 10 the best configurations combinations of schema, par- ity of RDDs a specific named schema with typed 10 11 titioning, and storage for seeking better performance. columns like in relational databases. Spark-SQL [12] 11 12 In particular, this paper applies existing techniques for is a high-level library for processing DataFrames in 12 13 ranking experimental results, and discusses their pros a relational manner. In particular, it allows querying 13 14 and cons alongside with their limitations. Last but not DataFrames using an SQL-like language, and it relies 14 15 least, the paper shows how to combine ranking criteria on the Catalyst query optimizer4. 15 16 (i.e. relational schemas, partitioning techniques, and 16 17 storage backends) to better investigate the trade-offs 2.2. Relational RDF Schemas 17 18 that occur across these experimental dimensions. 18 19 The remainder of the paper is organized as follows: Although triplestores can be used to efficiently store 19 20 Section 2 presents an overview of the required knowl- and manage RDF data [13] , some research works sug- 20 21 edge to understand the content of the paper. Section 3 gest how to manage RDF data in relational stores [14]. 21 22 describes the benchmarking scenario of our study. Sec- In the following, we present three relational schemas 22 23 tion 4 describes the experimental setup of our bench- that are suitable for representing RDF data. For each 23 24 mark. While, section 5 presents the analysis methodol- schema we give an example of data using Listing 1, 24 25 ogy followed to analyze the results. Section 6 discusses and we provide the respective SQL translation of the 25 26 these results and various insights regarding them. We SPARQL query in Listing 2. 26 27 discuss the related work in Section 7, before we con- 27 28 :Journal1 rdf:type :Journal ; 28 clude the paper in Section 8. dc:title "Journal 1 (1940)" ; 29 dcterms:issued "1940" . 29 30 2. Background :Article1 rdf:type :Article ; 30 dc:title "richer dwelling scrapped" ; 31 dcterms:issued "2019" ; 31 32 In this section, we present the necessary background :journal :Journal1 . 32 33 to understand the content of the paper. We assume that 33 Listing 1: RDF example in N-Triples. Prefixes are 34 the reader is familiar with RDF data model and the 34 omitted. 35 SPARQL query language. 35 36 SELECT ? yr 36 37 2.1. Spark & Spark-SQL WHERE { ?journal rdf:type bench:Journal. 37 ?journal dc:title "Journal 1 ( 1 9 4 0 ) " 38 ?journal dcterms:issued ?yr. } 38 39 Apache Spark [3] is an in-memory distributed com- 39 40 puting framework for large scale data processing. Listing 2: SPARQL Example against RDF graph in 40 41 At the Spark’s core there are Resilient Distributed Listing 1.1. Prefixes are omitted. 41 42 Datasets (RDDs, i.e., immutable distributed collection 42 43 of data elements. Single Statement Table Schema requires to store 43 44 Spark supports different storage backends for read- RDF triples in a single table with three columns that 44 45 ing and writing data. Those that are relevant for our represent the three components of the RDF triple, i.e., 45 46 performance evaluation are Apache Hive, i.e., a data subject, predicate, and object. ST schema is widely 46 47 warehouse built on top of Apache Hadoop for pro- 47 48 viding data query and analysis [11]; the Hadoop Dis- 48 1https://parquet.apache.org/ 49 49 tributed File System (HDFS). In particular, HDFS 2https://avro.apache.org/ 50 supports the following file formats: (i) Comma Sepa- 3https://orc.apache.org/ 50 51 rated Values (CSV), which is a readable and easy to de- 4https://databricks.com/glossary/catalyst-optimizer 51 Mohamed Ragab et al. / Systematic Performance Analysis of Distributed SPARQL Query Answering Using Spark-SQL 3 1 adopted [15, 16]. For instance, the major open-source Subject-Based Partitioning (SBP) requires to dis- 1 2 triplestores, i.e., Apache Jena, RDF4J and Virtuoso, tribute triples to the various partitions according to the 2 3 use the ST schema for storing RDF data. Figure 1 hash value computed for the subjects. As a result, all 3 4 shows the ST schema representation of the sample in the triples that have the same subject are assumed to 4 5 Listing 1, and the associated SQL translation for the reside on the same partition. In our scenario, we ap- 5 6 SPARQL query in Listing 2. plied spark partitioning using the subject as a key with 6 7 Vertically Partitioned Tables Schema requires to our different relational schema tables/Dataframes.
Details
-
File Typepdf
-
Upload Time-
-
Content LanguagesEnglish
-
Upload UserAnonymous/Not logged-in
-
File Pages21 Page
-
File Size-