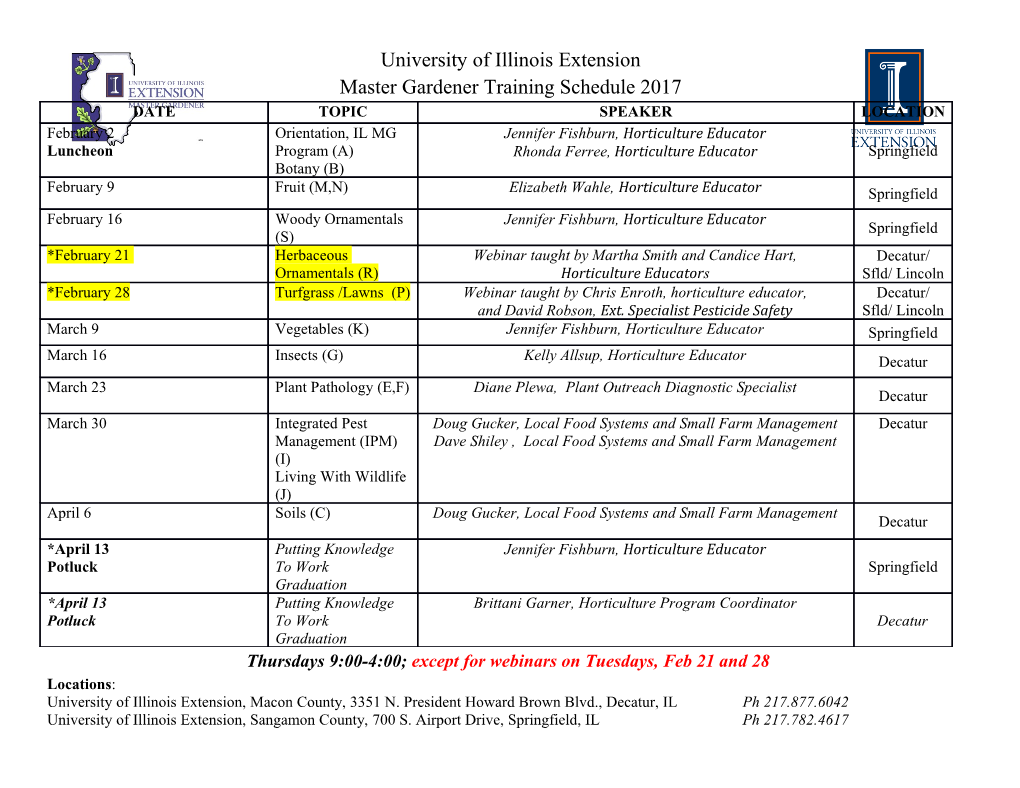
International Journal of Bifurcation and Chaos, Vol. 17, No. 7 (2007) 2491–2500 c World Scientific Publishing Company EPIDEMIC PREDICTABILITY IN META-POPULATION MODELS WITH HETEROGENEOUS COUPLINGS: THE IMPACT OF DISEASE PARAMETER VALUES V. COLIZZA∗,†, A. BARRAT∗,‡,M.BARTHELEMY´ § and A. VESPIGNANI† ∗Complex Networks Lagrange Laboratory (CNLL), Institute for Scientific Interchange (ISI ), 10133 Turin, Italy †School of Informatics and Biocomplexity Institute, Indiana University, Bloomington, IN 47408, USA ‡Laboratoire de Physique Th´eorique, (UMR du CNRS 8627), Universit´edeParis-Sud, Orsay, France §CEA-DIF Centre d’Etudes de Bruy`eres-Le-Chˆatel, BP12, F-91680, France Received June 16, 2006; Revised September 12, 2006 We study the predictability of epidemic forecasts in a data-driven meta-population model con- sidering the complete air transportation system and the associated urban areas. We define the predictability as the robustness of the system evolution with respect to the stochastic fluctu- ations. As a quantitative measure of predictability we consider the information similarity of the time series characterizing different epidemic outbreaks with the same initial conditions. We study the predictability as a function of the parameters describing the basic susceptible-latent- infected and recovered disease dynamics. We find that the overall predictability is determined by the level of sampling of the underlying travel pattern by infected and latent individuals. Keywords: Epidemiology; complex networks; stochastic noise; predictability. 1. Introduction Lloyd & May, 1996; Grenfell & Bolker, 1998; Keel- Large scale epidemic forecast is crucially depen- ing & Rohani, 1995; Ferguson et al., 2003]. The dent upon the accurate and realistic modeling heterogeneities and details of the population struc- where the movement of individuals at various lev- ture have become increasingly important features els is taken into account. In the case of spa- and the introduction of large scale agent based mod- tially extended systems, modeling approaches have els has enabled the simulation of the propagation of evolved into meta-population schemes which explic- an infectious disease at the level of the single indi- itly include spatial structures and consist of mul- vidual [Chowell et al., 2003; Eubank et al., 2004; tiple subpopulations coupled by the movement of Ferguson et al., 2005; Longini et al., 2005]. If one people among those [Anderson & May, 1984; May focuses on the world-wide level, the large scale & Anderson, 1984; Bolker & Grenfell, 1993, 1995; and geographical impact of infectious diseases on 2491 2492 V. Colizza et al. populations in the modern era is mainly due to The paper is organized as follows: we recall the commercial air travel. For this reason, in the early modeling framework in Sec. 2; Sec. 3 presents the 80’s, models aimed at forecasting the geographi- results regarding the impact of disease parameter cal spread of epidemics by using information on values on quantitative forecasting of epidemic out- the airplane passenger fluxes among cities have breaks. Finally, we consider some conclusions and been developed [Rvachev & Longini, 1985], capi- directions for future work in Sec. 4. talizing on previous studies on the Russian airline network [Baroyan et al., 1969]. Similar modeling 2. Meta-Population Model approaches have been used to study specific out- We consider the general modeling framework pre- breaks such as pandemic influenza [Longini, 1988; sented in [Colizza et al., 2006a, 2006b], which Grais et al., 2004], HIV [Flahault & Valleron, 1991], describes the world-wide propagation of a dis- and SARS [Hufnagel et al., 2004]. More recently, ease through a meta-population approach, in the the availability of complete datasets [Guimer`a& same spirit as in the models of [Longini, 1988; Amaral, 2004; Barrat et al., 2004; Guimer`a et al., Grais et al., 2003; Flahault & Valleron, 1991; Huf- 2005] has allowed to build modeling frameworks for nagel et al., 2004]. The spatial structure is explicitly the computational study of epidemics taking into included in the model by considering multiple sub- account the full air transportation systems and the populations coupled by movements of individuals. census data of the corresponding urban areas [Col- More specifically, the sub-populations correspond izza et al., 2006a, 2006b]. In these approaches the to urban areas and individuals in each urban area only parameters in the models are the disease are allowed to travel according to the routes of the transition rates describing the etiology of the dis- airline transportation system. ease. All the couplings due to transportation terms, urban area size and the network connectivity pat- 2.1. Intra-city level: SEIR infection tern are fixed elements of the problem obtained dynamics from real data. While the ability to forecast the spread of The epidemic evolution inside each urban area is an infectious disease is inevitably entangled with described by a fully mixed population with a basic the accuracy and the level of realism introduced standard compartmentalization in which each indi- in the modeling approach, the impact of the vidual can only exist in one of the following discrete parameters and model features on the reliability states such as susceptible (S), latent (E), infected (I) of the predicted scenarios is difficult to assess. or permanently recovered (R). This set of states do Here we investigate how different communicable not correspond strictly to any particular disease but diseases — characterized by different epidemi- encompasses the most relevant features and param- ological parameters regarding infectiousness and eters of a variety of different virus transmission, transition rates — might affect our ability to by including specific stages of the disease dynam- predict their geographical and temporal propaga- ics such as e.g. the latency period. More refined and tion. In order to tackle this issue we consider extended compartmentalizations can be considered. the meta-population model defined in [Colizza In each city j the population is Nj and the num- et al., 2006a, 2006b] for the forecast of the world- bers of individuals in the various classes at time t wide spread of emerging diseases. We perform are denoted as Sj(t), Ej(t), Ij(t)andRj(t) respec- a systematic analysis of the effect of the dis- tively. By definition Nj = Sj(t)+Ej(t)+Ij(t)+ ease parameters on the statistical similarity of Rj(t). Individuals change compartment according the epidemic behavior evolution obtained with to the following rules: during the time interval the same initial conditions and different noise dt, the probability that a susceptible individual realizations. This amounts to the assessment of acquires the infection from any given infected indi- the robustness of the obtained predictions with vidual, and becomes latent, is proportional to βdt, respect to the inherent randomness of the epi- where β is the transmission parameter that cap- demic evolution. The analysis shows that the over- tures the etiology of the infection process. Latents all predictability of the epidemics is determined by become infected with probability dt, and infected competing effects related to the number of infected individuals recover with a probability µdt,where individuals and the heterogeneity of the traveling −1 and µ−1 are the average latency time and aver- pattern. age infection duration. Epidemic Predictability in Meta-Population Models with Heterogeneous Couplings 2493 The populations Nj of the large metropolitan 2.3. Global stochastic epidemic model areas served by the airports are obtained by differ- In each city we work directly with the master equa- ent census sources freely available on the Internet. tions for the dynamical rules given in Sec. 2.1. For large cities, Nj represents the population of the Under the assumption of large populations, we whole metropolitan area. The final data set contains obtain the Langevin equations for the numbers of the population of the 3100 largest airports. susceptible, latent, infected and recovered individu- 2.2. Transport operator als and we associate to each reaction process a noise term with amplitude proportional to the square root In addition to the infection dynamics taking place of the reaction term (for details, see [Colizza et al., inside each urban area, the epidemic evolution at 2006b; Gardiner, 2004; Marro & Dickman, 1998]). the global level is governed by the travel of individ- The noise term accounts for the stochastic nature of uals from one city to another by means of the airline the infection dynamics of directly transmitted dis- transportation network, therefore infecting different eases, with intrinsic fluctuations in the infectious regions of the world. The network is obtained by contact process, the generation of new cases and considering the International Air Transport Associ- 1 the removal of infectious individuals. These stochas- ation (IATA) database, which contains the world tic nonlinear differential equations are then coupled list of airport pairs connected by direct flights and through the transport operator, yielding the follow- the number of available seats on any given connec- ing set of stochastic discretized differential equa- tion. The resulting world-wide air-transportation tions for the worldwide spread of the infection: network is therefore a weighted graph compris- V ing = 3100 vertices denoting airports in 220 Sj(t +∆t) − Sj(t) different countries and E = 17 182 weighted edges whose weights represent the passenger
Details
-
File Typepdf
-
Upload Time-
-
Content LanguagesEnglish
-
Upload UserAnonymous/Not logged-in
-
File Pages10 Page
-
File Size-