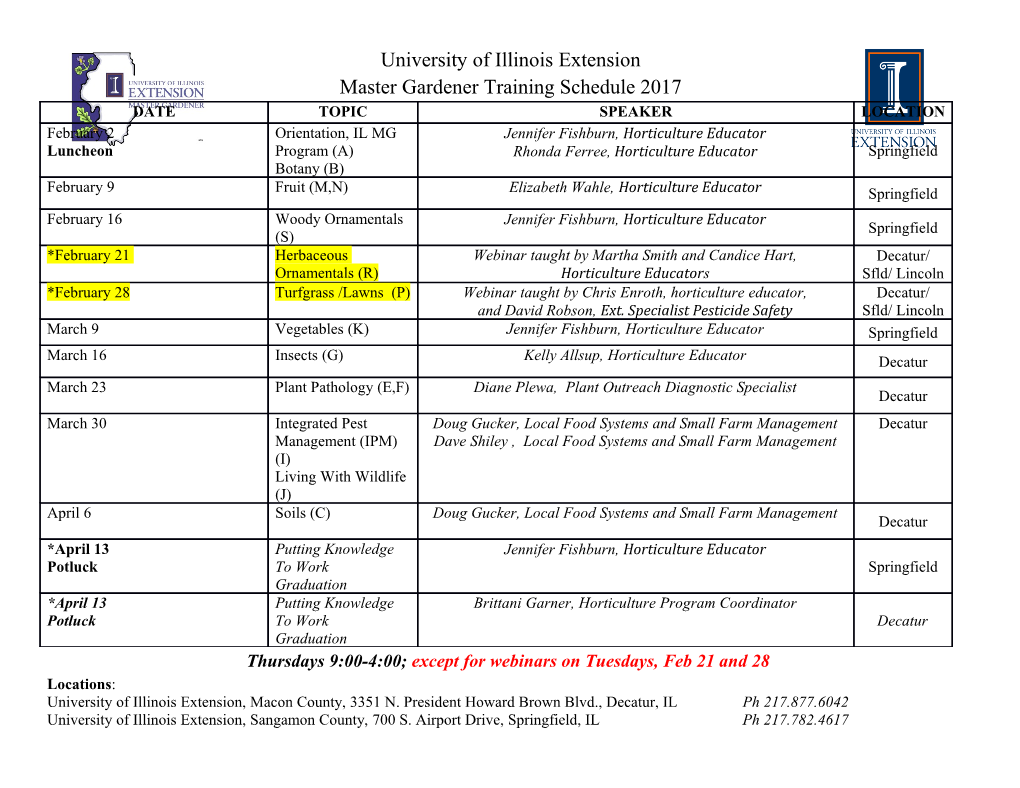
Global Ecology and Conservation 24 (2020) e01341 Contents lists available at ScienceDirect Global Ecology and Conservation journal homepage: http://www.elsevier.com/locate/gecco Modelling the habitat of the endangered Carpentarian Grasswren (Amytornis dorotheae): The importance of spatio- temporal habitat availability in a fire prone landscape * Henry J. Stoetzel a, , Nicholas P. Leseberg a, Stephen A. Murphy a, Margaret E. Andrew b, Kayler J. Plant c, Graham N. Harrington d, James E.M. Watson a, e a School of Earth and Environmental Sciences, University of Queensland, St Lucia, QLD, 4072, Australia b Environmental and Conservation Sciences, Murdoch University, Murdoch, WA, 6150, Australia c Southern Gulf NRM, 43 Barkly Hwy, Mount Isa, QLD, 4825, Australia d Birdlife Northern Queensland, PO Box 680, Malanda, QLD, 4885, Australia e Wildlife Conservation Society, Global Conservation Program, 2300 Southern Boulevard Bronx, New York, 10460, NY, USA article info abstract Article history: Species distribution modelling (SDM), a tool increasingly adopted to quantify geographic Received 22 October 2020 range size, often predicts species’ distributions as static. However, habitat availability may Accepted 23 October 2020 exhibit spatial and temporal variation when dynamic processes, such as fire, determine suitability. Static SDM approaches may not satisfactorily represent this dynamic process. Keywords: We investigated the potential use of SDM to quantify dynamic habitat availability by Habitat dynamics applying the MaxEnt SDM technique to model the habitat of the Carpentarian Grasswren Species distribution modelling (Amytornis dorotheae), an endangered Australian passerine dependent on long unburnt Threatened species fi Extinction risk vegetation in a re prone system. By adjusting a typical SDM approach to incorporate the fi Fire frequency dynamic nature of re, we modelled the spatio-temporal variation of suitable habitat over Time since fire 12 years and compared it to a static modelling approach. Incorporating fire as a dynamic process increased the importance of the fire variable to models (from <18% to >35% per- mutation importance) and improved model performance, as evaluated by the AUC using cross-validation. Our dynamic model revealed sizeable temporal variation in the area and spatial arrangement of suitable habitat that was not apparent in the static model. This result may partly solve the mystery of why the species occurs as widely separated pop- ulations despite the presence of seemingly suitable intervening habitat. In areas where the species is no longer found, habitat availability was less consistent due to frequent fire, and fire refugia was more limited and isolated, when compared to sites with recent records. These results demonstrate that, when compared to a static approach, a dynamic SDM approach can lead to improved understanding of dynamic ecological processes, and their impact on a species. © 2020 The Authors. Published by Elsevier B.V. This is an open access article under the CC BY-NC-ND license (http://creativecommons.org/licenses/by-nc-nd/4.0/). * Corresponding author. Level 2, Steele Building (3), Room 210, University of Queensland, St Lucia, QLD, 4072, Australia. E-mail address: [email protected] (H.J. Stoetzel). https://doi.org/10.1016/j.gecco.2020.e01341 2351-9894/© 2020 The Authors. Published by Elsevier B.V. This is an open access article under the CC BY-NC-ND license (http://creativecommons.org/ licenses/by-nc-nd/4.0/). H.J. Stoetzel, N.P. Leseberg, S.A. Murphy et al. Global Ecology and Conservation 24 (2020) e01341 1. Introduction Understanding the distribution of a species, and the environmental variables that constrain it, is essential when evaluating that species’ threat status and conservation requirements (Kukkala and Moilanen 2013; Kujala et al., 2018). Species distri- bution modelling (SDM) is an established approach that uses environmental characteristics and known occurrence locations to predict the spatial extent of suitable habitat, and therefore a species’ potential distribution (Pearson 2007). The outputs from SDMs can provide information useful for conservation management (Pearson 2007; Villero et al., 2017), identifying previously unknown sites of occurrence and mechanisms of population separation (Lu et al., 2012; Fois et al., 2018; Bertola et al., 2019). SDMs typically combine static environmental predictors, or predictors that average environmental variability over time, with a set of species occurrence records (Thuiller and Münkemüller 2010). However, it has been established that these static models are unlikely to capture complex patterns of species occurrence when dynamic ecological processes are present (Franklin 2010; Runge et al., 2015; Zurell et al., 2016; Williams et al., 2017a; Andrew and Fox 2020). Therefore, a static SDM approach may not accurately estimate a species’ status if population size responds to significant changes in suitable habitat through time (Purvis et al., 2000; Runge et al., 2015; Webb et al., 2017). One approach to understanding dynamic distributions is to project an SDM model onto temporally explicit environmental conditions. Numerous studies have used this approach to predict potential distribution shifts for species under future climate scenarios (Kearney et al., 2010; Thuiller and Münkemüller 2010; Austin and Van Niel 2011; Reside et al., 2012b). More recently, distribution modellers have revealed temporal variation in suitable habitat at shorter time scales in response to dynamic resource availability (Osborne and Suarez-Seoane 2007; Runge et al., 2015; Webb et al., 2017; Andrew and Fox 2020). For example, Runge et al. (2015) created a series of time sliced layers depicting temporally explicit weather conditions that drive resource availability for many of Australia’s nomadic, arid zone avifauna. Dynamic habitat availability is relevant to many species worldwide, and we propose dynamic modelling, using time-sliced predictions, is potentially applicable to understanding species responses to ecological disturbance. The tropical savannas of Australia’s north are an environment that experiences conditions that are highly variable at the local scale but broadly predictable at the landscape scale (Williams et al., 2017b). In the open grassy woodlands that dominate the region, wet-season rainfall is followed by a hot dry-season, allowing for a cycle of prolific growth and curing and creating ideal conditions for fire (Russell-Smith and Edwards 2006). Large proportions of this biome burn each year (Russell-Smith et al., 2003). While some species use recently burnt habitat, others are dependent on vegetation structure only provided by late-successional habitat (Clarke 2008; Connell et al., 2017). For both cases, the occurrence of fire will result in spatio- temporal shifts in habitat suitability. For some systems, such as those dominated by the genus of highly flammable grass Triodia, the return to their pre-fire state may take several years. Species that depend on late successional habitat within these systems are therefore vulnerable to the impacts of frequent fire (Horton 2011). Changes in the nature and frequency of fire across northern Australia is a known threatening process that has been implicated in changes to species assemblages (Woinarski and Legge 2013) and declines of many species (Garnett et al., 2011). One such species is the Carpentarian Grasswren (Amytornis dorotheae), a small passerine (16e17.5 cm; 21e25 g), endemic to northern Australia (Higgins 2001). It occurs in four recognised sub-populations that each occupy distinct localities stretching from the McArthur River in the Northern Territory, southeast to Mount Isa in north-western Queensland (Harrington and Murphy 2016). Long unburnt and therefore structurally complex Triodia hummocks provide the Carpentarian Grasswren with foraging substrates, nest sites and refuge from predators and bad weather (Higgins 2001; Perry et al., 2011; Harrington and Murphy 2016). Altered fire regimes that reduce the availability of long unburnt habitat are assumed to be the species’ main threat, and as a result, it has been listed federally as endangered (Australian Government 2019). However, the link between fire and population decline is largely circumstantial (Harrington and Murphy 2016) and a more thorough exploration of this relationship is needed to better define the species’ population trajectory and true conservation status. Fire is known to influence the distributions of many species, and the most appropriate measures of fire to consider when modelling a species’ distribution will depend on the species of interest (Reside et al., 2012a; Tucker et al., 2012; Watson et al., 2012; Connell et al., 2017; Kelly et al., 2017). Temporal summaries like fire frequency and inter-fire interval have been used to account for fire regimes in SDM (Kelly et al., 2017). Single predictions derived from these measures of fire may only identify areas that have fire regimes broadly suitable for a species persistence, but may fail to identify suitable habitat at any given point in time. Alternatively, a time since fire layer can account for a species preference for particular post-fire successional habitat in SDM (Connell et al., 2017; Lee et al., 2018). However, a single SDM prediction using time since fire depicts habitat at an exact point in time and does not reflect the variation of this habitat through time. To our knowledge, using SDM to investigate the dynamic nature of suitable habitat over successive years in response to annual fire
Details
-
File Typepdf
-
Upload Time-
-
Content LanguagesEnglish
-
Upload UserAnonymous/Not logged-in
-
File Pages13 Page
-
File Size-