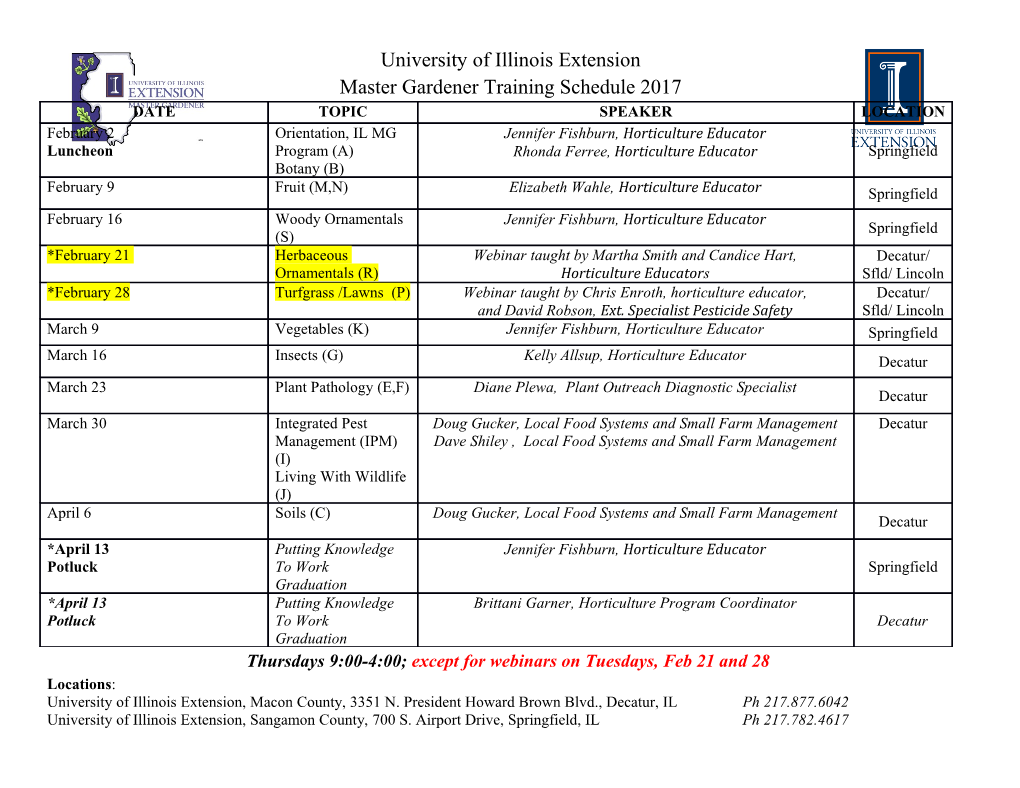
Institute of Mathematical Statistics LECTURE NOTES–MONOGRAPH SERIES Recent Developments in Nonparametric Inference and Probability Festschrift for Michael Woodroofe Jiayang Sun, Anirban DasGupta, Vince Melfi, Connie Page, Editors Volume 50 ISBN 0-940600-66-9 Institute of Mathematical Statistics LECTURE NOTES–MONOGRAPH SERIES Volume 50 Recent Developments in Nonparametric Inference and Probability Festschrift for Michael Woodroofe Jiayang Sun, Anirban DasGupta, Vince Melfi, Connie Page, Editors Institute of Mathematical Statistics Beachwood, Ohio, USA Institute of Mathematical Statistics Lecture Notes–Monograph Series Series Editor: R. A. Vitale The production of the Institute of Mathematical Statistics Lecture Notes–Monograph Series is managed by the IMS Office: Jiayang Sun, Treasurer and Elyse Gustafson, Executive Director. Library of Congress Control Number: 2006934183 International Standard Book Number 0-940600-66-9 International Standard Serial Number 0749-2170 Copyright c 2006 Institute of Mathematical Statistics All rights reserved Printed in the United States of America Contents Preface Jiayang Sun, Anirban DasGupta, Vince Melfi and Connie Page ............. v Contributors to this volume ................................................. vii PROBABILITY, BAYESIAN INFERENCE AND STOCHASTIC PROCESS Group invariant inferred distributions via noncommutative probability B. Heller and M. Wang ................................... 1 Invariance principles for fractionally integrated nonlinear processes Wei Biao Wu and Xiaofeng Shao .............................. 20 Random walk on a polygon Jyotirmoy Sarkar ....................................... 31 CONTEMPORARY SEQUENTIAL ANALYSIS Bias correction and confidence intervals following sequential tests Tze Leung Lai, Zheng Su and Chin Shan Chuang ..................... 44 Multivariate sequential analysis with linear boundaries Robert Keener ......................................... 58 Corrected confidence intervals for secondary parameters following sequential tests R. C. Weng and D. S. Coad ................................. 80 Efficient three-stage t-tests Jay Bartroff ..........................................105 BIASED SAMPLING, MEASUREMENT ERROR MODELS AND RESTRICTED INFERENCE On the behavior of Bayesian credible intervals for some restricted parameter space problems Eric´ Marchand and William E. Strawderman ....................... 112 Sieve estimates for biased survival data Jiayang Sun and Bin Wang .................................127 Existence of the signal in the signal plus background model Tonglin Zhang .........................................144 A test for equality of multinomial distributions vs increasing convex order Arthur Cohen, John Kolassa and Harold Sackrowitz ................... 156 NONLINEAR RENEWAL THEORY Nonlinear renewal theorems for random walks with perturbations of intermediate order Keiji Nagai and Cun-Hui Zhang ..............................164 A non-linear Renewal Theorem with stationary and slowly changing perturbations Dong-Yun Kim and Michael Woodroofe ..........................176 MULTIPLE TESTING, FDR, STATISTICS IN IMAGING AND DATA MINING On the false discovery rates of a frequentist: Asymptotic expansions Anirban DasGupta and Tonglin Zhang ...........................190 Spatial-temporal data mining procedure: LASR Xiaofeng Wang, Jiayang Sun and Kath Bogie ....................... 213 iii Preface There have been extensive developments recently in modern nonparametric infer- ence and modeling. Nonparametric and semi-parametric methods are especially useful with large amounts of data that are now routinely collected in many areas of science. Probability and stochastic modeling are also playing major new roles in scientific applications. This refereed special volume highlights challenges and developments at this in- terface of statistics, probability and the sciences, and honors Michael B. Woodroofe for his pioneering contributions to nonparametric inference and probability. Topics covered include biased sampling and missing data, shape-restricted infer- ence, contemporary sequential analysis, modern nonparametric inference, probabil- ity, and statistics applications. Some of the papers in this volume were presented at “A Conference on Nonparametric Inference and Probability with Applications to Science”, September 24–25, 2005, at Ann Arbor, Michigan: http:// www.stat.lsa.umich.edu/conference/mw2005/ for which there were 97 pre-registered participants. The conference was organized by Robert Keener and Jiayang Sun. Invited Speakers and participants included: Persi Diaconis, Robert Keener, Steve Lalley, T.L. Lai, Mario Mateo, Mary Meyer, Vijay Nair, Gordie Simons, Jiayang Sun, Michael Woodroofe, Wei Biao Wu, Cun-Hui Zhang, Charles Hagwood, Steve Coad, Hira Koul, Anand Vidyashenkar, Zhiliang Ying, Arniban DasGupta, Moulinath Banerjee, Anna Amirdjanova, Bill Strawder- man, Connie Page, Tom Sellke, Byron Roe, Vince Melfi, and Herman Chernoff. Michael Woodroofe has had a distinguished career and is widely recognized as a pre-eminent scientist in statistics and probability. He has broad interests and has made deep and significant contributions in many areas, many represented by sessions at this conference. His work in probability, sequential analysis and inference is regarded as seminal and pioneering and has influenced an entire generation of researchers. He has published more than 100 research articles, written a SIAM monograph, and authored a book. He is a former Editor of the Annals of Statistics, a member of Phi Beta Kappa, and a fellow of the Institute of Mathematical Statistics and an elected member of the International Statistical Institute. We thank the National Science Foundation, the National Security Agency, and the University of Michigan, (the Department of Statistics; the College of Litera- ture, Science, and the Arts; the Office of the Vice President for Research; and the Rackham Graduate School) for their financial support to the conference, and the Institute of Mathematical Statistics, and the American Statistical Association for the co-sponsorship. We also thank Amy Rundquist for conference administration. Jiayang Sun, Anirban DasGupta, Case Western Reserve University Purdue University Vince Melfi, Connie Page, Michigan State University Michigan State University v Special Thanks to Editorial Assistants Steve Ganocy Xiaofeng Wang vi Contributors to this volume Bartroff, J., Stanford University Bogie, K., Cleveland FES center Chuang, C. S., Millennium Partners Coad, D. S., University of London Cohen, A., Rutgers University DasGupta, A., Purdue University Heller, B., Illinois Institute of Technology Keener, R., University of Michigan Kim, D.-Y., Michigan State University Kolassa, J., Rutgers University Lai, T. L., Stanford University Marchand, E., Universit´ede Sherbrooke Nagai, K., Yokohama National University Sackrowitz, H., Rutgers University Sarkar, J., Indiana University Purdue University Indianapolis Shao, X., University of Chicago Strawderman, W. E., Rutgers University Su, Z., Stanford University Sun, J., Case Western Reserve University Wang, B., University of South Alabama Wang, M., University of Chicago Wang, X., The Cleveland Clinic Foundation Weng, R. C., National Chengchi University Woodroofe, M., the University of Michigan Wu, W. B., University of Illinois, at Urbana-Champaign Zhang, C.-H., Rutgers University Zhang, T., Purdue University vii IMS Lecture Notes–Monograph Series Recent Developments in Nonparametric Inference and Probability Vol. 50 (2006) 1–19 c Institute of Mathematical Statistics, 2006 DOI: 10.1214/074921706000000563 Group invariant inferred distributions via noncommutative probability 1 2, B. Heller and M. Wang ∗ Illinois Institute of Technology and University of Chicago Abstract: One may consider three types of statistical inference: Bayesian, fre- quentist, and group invariance-based. The focus here is on the last method. We consider the Poisson and binomial distributions in detail to illustrate a group invariance method for constructing inferred distributions on parameter spaces given observed results. These inferred distributions are obtained without using Bayes’ method and in particular without using a joint distribution of random variable and parameter. In the Poisson and binomial cases, the final formulas for inferred distributions coincide with the formulas for Bayes posteriors with uniform priors. 1. Introduction The purpose of this paper is to construct a probability distribution on the parame- ter space given an observed result (inferred distribution) in the case of a discrete random variable with a continuous parameter space using group theoretic meth- ods. We present two examples, the Poisson and binomial distributions. From the point of view of posterior Bayes distributions, these group theoretic methods lead to uniform non-informative prior distributions on canonical parameters for both the Poisson and binomial cases. Alternatively, posterior distributions are obtained here from group theory alone without explicitly using Bayes method. The construction of inferred probability distributions by non-Bayesian methods has a long history beginning with Fisher’s fiducial method of inference. The use of group theoretic methods to construct pivotal functions also has a long history as introduced in Fraser (1961) and amplified by many others since then. Briefly, the group theoretic or “invariance” method of inference has operated from a context in which a group G acts upon both the parameter space and the sample space. Consider
Details
-
File Typepdf
-
Upload Time-
-
Content LanguagesEnglish
-
Upload UserAnonymous/Not logged-in
-
File Pages239 Page
-
File Size-