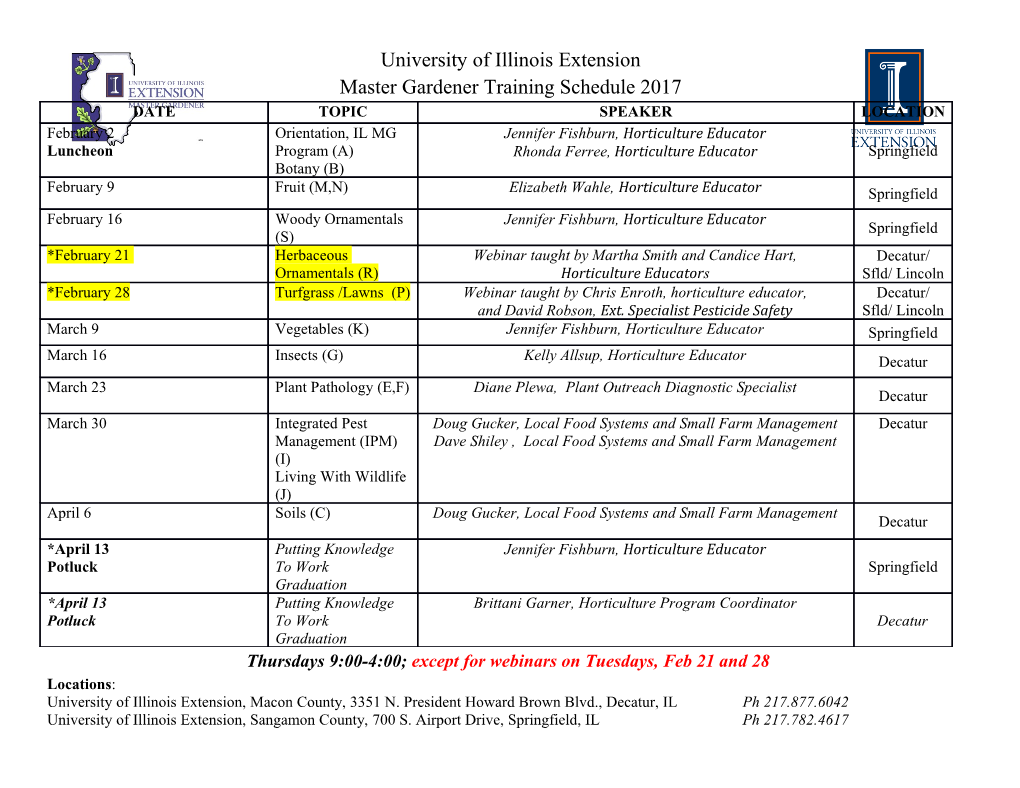
Research Article Derivation of Stable Microarray Cancer-Differentiating Signatures Using Consensus Scoring of Multiple Random Sampling and Gene-Ranking Consistency Evaluation Zhi Qun Tang,1,2 Lian Yi Han,1,2 Hong Huang Lin,1,2 Juan Cui,1,2 Jia Jia,1,2 Boon Chuan Low,2,3 Bao Wen Li,2,4 and Yu Zong Chen1,2 1Bioinformatics and Drug Design Group, Department of Pharmacy; 2Center for Computational Science and Engineering; and Departments of 3Biological Sciences and 4Physics, National University of Singapore, Singapore, Singapore Abstract sampling methods. Only 1 to 5 of the 4 to 60 selected predictor Microarrays have been explored for deriving molecular genes in each of these sets are present in more than half of the signatures to determine disease outcomes, mechanisms, other nine sets (Table 1), and 2 to 20 of the predictor genes in each targets, and treatment strategies. Although exhibiting good set are cancer related (Table 2). Despite the use of sophisticated predictive performance, some derived signatures are unstable class differentiation and signature selection methods, the selected due to noises arising from measurement variability and signatures show few overlapping predictor genes, as in the case of biological differences. Improvements in measurement, anno- other microarray data sets including non–Hodgkin lymphoma, tation, and signature selection methods have been proposed. acute lymphocytic leukemia, breast cancer, lung adenocarcinoma, We explored a new signature selection method that incorpo- medulloblastoma, hepatocellular carcinoma, and acute myeloid rates consensus scoring of multiple random sampling and leukemia (9, 15). multistep evaluation of gene-ranking consistency for maxi- Although these signatures display high cancer differentiation mally avoiding erroneous elimination of predictor genes. This accuracies at levels of 85.9% to 100%, the highly unstable and method was tested by using a well-studied 62-sample colon patient-dependent nature of these signatures diminishes their cancer data set and two other cancer data sets (86-sample lung application potential for diagnosis and prognosis (9). Moreover, the adenocarcinoma and 60-sample hepatocellular carcinoma). complex and heterogenic nature of cancer may not be adequately For the colon cancer data set, the derived signatures of described by the few cancer-related genes in some of these 20 sampling sets, composed of 10,000 training test sets, are signatures. The unstable nature of these signatures and their lack of fairly stable with 80% of top 50 and 69% to 93% of all predictor disease-relevant genes also limit their potential for target discovery. genes shared by all 20 signatures. These shared predictor The instability of derived signatures is likely caused by the noises in genes include 48 cancer-related and 16cancer-implicated the microarray data arising from measurement variability and genes, as well as 50% of the previously derived predictor genes. biological differences (9, 14). These noises arise from such factors The derived signatures outperform all previously derived as the precision of measured absolute expression levels, capability signatures in predicting colon cancer outcomes from an for detecting low abundance genes, quality of design and probes, independent data set collected from the Stanford Microarray annotation accuracy and coverage, and biological differences of Database. Our method showed similar performance for the expression profiles (14, 17). Apart from enhancing the quality of other two data sets, suggesting its usefulness in deriving stable measurement and annotation (17), strategies for improving signatures for biomarker and target discovery. [Cancer Res signature selection have also been proposed. These include the 2007;67(20):9996–10003] use of multiple random validation (9), larger sample size (18), known mechanisms (19), and signature selection methods less Introduction sensitive to noises (1, 14, 20). We explored a new signature selection method aimed at Microarrays have been explored for deriving molecular signa- reducing the chances of erroneous elimination of predictor genes tures, which are subsets of genes differentially expressed in patients due to the noises contained in microarray data set. In our of different disease outcomes, for disease diagnosis and prognosis approach, non–predictor genes were eliminated by consensus (1–6), and for determining disease mechanisms (1, 7), targets (8), scoring of a large number of training and test sets generated from and treatment strategies (9, 10). Although showing good predictive repeated random sampling (9), and by incorporating a multistep performance (4, 5, 9, 11–13), the derived signatures have been gene-ranking consistency evaluation procedure into a well- found to be highly unstable and to include fewer disease-related established signature selection method. Our method was tested genes (9, 14, 15). For instance, 10 different sets of signatures for by using a well-studied colon cancer data set (16) and two other separating colon cancer tissues and normal colon tissues have been data sets—86 samples of lung adenocarcinoma (21) and 60 samples derived from the same 62-sample data set (16) by using different of hepatocellular carcinoma (22) so that our derived signatures can be adequately evaluated and compared with those of other studies using different sampling sets, class differentiation methods, Note: Supplementary data for this article are available at Cancer Research Online (http://cancerres.aacrjournals.org/). and feature selection methods. The performance of selected Requests for reprints: Yu Zong Chen, Department of Pharmacy, National signatures for the colon cancer data set was further evaluated by University of Singapore, S16, Level 8, 6 Science Drive 2, Singapore 117546, Singapore. applying the selected signatures in predicting colon cancer Phone: 65-6516-6877; Fax: 65-6774-6756; E-mail: [email protected]. I2007 American Association for Cancer Research. outcomes from an independent colon cancer data set separately doi:10.1158/0008-5472.CAN-07-1601 generated from Stanford Microarray Database (23), and the Cancer Res 2007; 67: (20). October 15, 2007 9996 www.aacrjournals.org Downloaded from cancerres.aacrjournals.org on September 30, 2021. © 2007 American Association for Cancer Research. Stable Cancer Signature Derivation from Microarray Data performance of these signatures was compared with those of The performance of SVM classification can be measured by true positive previously derived colon cancer signatures applied to the same TP (number of cancer patients correctly predicted as cancer patient), false FN data set. negative (number of cancer patients incorrectly predicted as normal), true negative TN (number of normal correctly predicted normal), and false positive FP (number of normal incorrectly predicted as cancer Materials and Methods patients). Three indicators, sensitivity Qp = TP /(TP + FN), specificity Classification method. Support vector machines (SVM), a supervised Qn = TN /(TN + FP), and overall accuracy Q =(TP + TN)/(TP + FN + TN + FP), machine learning method, was used for training a class differentiation were used to measure the predictive performance. system (14, 24, 25). In classification of microarray data sets, it has been Feature selection method. Predictor genes of each training test set were found that supervised machine learning methods generally yield better selected by using SVM recursive feature elimination (RFE-SVM), which is a results (26), particularly for smaller sample sizes (14). In particular, SVM wrapper method that selects predictor genes by eliminating non–predictor consistently shows outstanding performance, is less penalized by sample genes according to a gene-ranking function generated from a class redundancy, and has lower risk for overfitting (25, 27). differentiation system (28). Wrapper methods generally perform better SVMs (24) project feature vectors into a high-dimensional feature space than other feature selection methods (28). RFE-SVM is the best performing jjX ÀX ¶jj2 by using a kernel function kðX ; X ¶Þ¼exp À 2r2 . The linear SVM wrapper method and has thus been more widely used in cancer microarray procedure can then be applied to the feature vectors in this feature space: analysis (24, 27). It constructs a hyperplane that separates two different classes of feature The ranking criterion of RFE-SVM is based on the change in the vectors with a maximum margin. This hyperplane is constructed by objective function upon removing each feature. To improve the efficiency finding a vector w and a variable b that minimizes kwk2, which satisfies of training, this objective function is represented by a cost function J for the following conditions: wÁxi + b z +1, for yi = +1 (cancer patients) the kth feature computed by using training set only. When a given feature and wÁxi + b V À1, yi = À1 (normal people). Here, xi is a feature vector, is removed or its weight wk is reduced to zero, the change in the cost y b k k J k DJðkÞ¼1 B2J ðDw Þ2 Dw w À 0 i is the group index, w is a vector normal to the hyperplane, | |/ w is function ( ) is given by 2 Bw2 k . The case of k = k k the perpendicular distance from the hyperplane to the origin, and kwk is corresponds to the removal of feature k. In our case, the change in the b DJðkÞ¼1 aT Ha À 1 aT HðÀkÞa H the Euclidean norm of w. After the determination of w and , a given cost function can be estimated as 2 2 2 , where jjxiÀxjjj vector x can be classified by using sign [(wÁx)+b]; a
Details
-
File Typepdf
-
Upload Time-
-
Content LanguagesEnglish
-
Upload UserAnonymous/Not logged-in
-
File Pages9 Page
-
File Size-