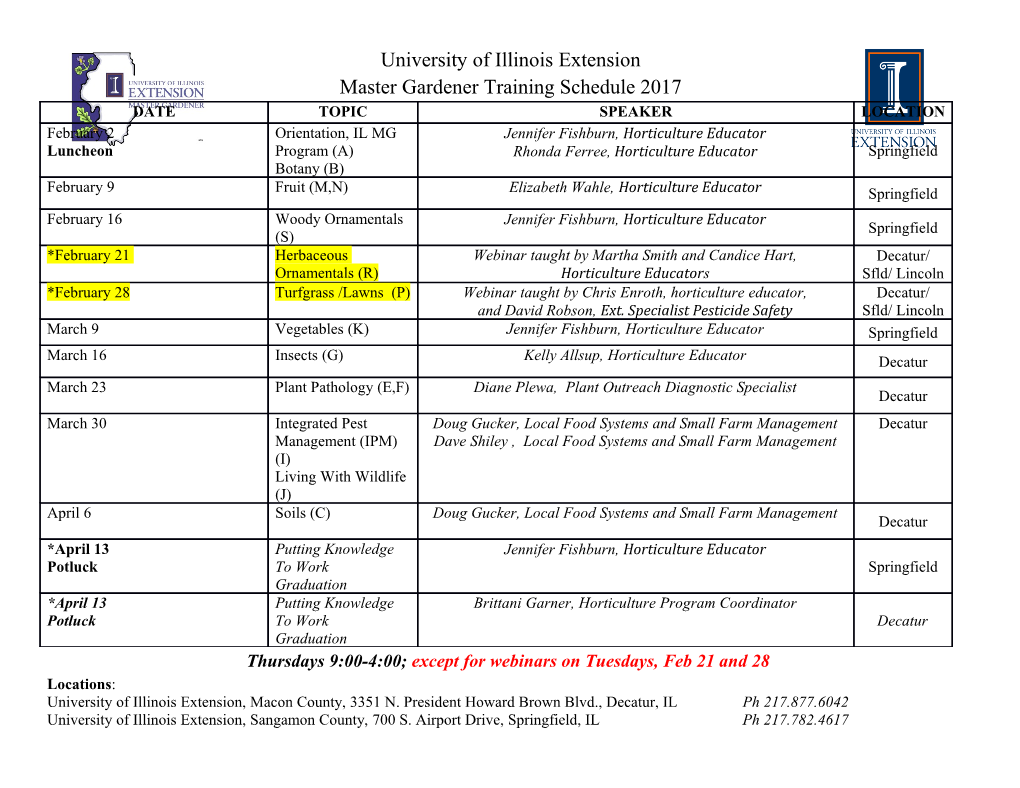
Memory-guided Exploration in Reinforcement Learning James L. Carroll, Todd S. Peterson & Nancy E. Owens Machine Intelligence, Learning, and Decisions Laboratory Brigham Young University Provo Ut. 84601 USA [email protected], [email protected], [email protected] Abstract 1.1 Proposed solution One approach to overcoming these problems is with the \life-long learning" paradigm for machine learn- The life-long learning architecture attempts to create ing [12][2][11][5]. In the life-long learning approach an an adaptive agent through the incorporation of prior agent encounters many di®erent tasks over its lifetime. knowledge over the lifetime of a learning agent. Our These tasks are often related to each other. Through paper focuses on task transfer in reinforcement learn- the appropriate transfer of knowledge it is possible for ing and speci¯cally in Q-learning. There are three main an agent to learn each new task with greater e±ciency. model free methods for performing task transfer in Q- Less training examples would be required because the learning: direct transfer, soft transfer and memory- agent could use past experience to ¯ll in the holes in its guided exploration. In direct transfer Q-values from a training set. Accurate models would be learned, and previous task are used to initialize the Q-values of the transferred. Such knowledge would be computer acces- next task. Soft transfer initializes the Q-values of the sible because it would be learned, and the need to hard new task with a weighted average of the standard ini- code such information would be reduced. By transfer- tialization value and the Q-values of the previous task. ring information from simpler problems to more com- In memory-guided exploration the Q-values of previous plex problems the tractability issues would be signi¯- tasks are used as a guide in the initial exploration of cantly reduced. Learning tends to adapt automatically the agent. The weight that the agent gives to its past to precision problems in the sensors and actuators. In experience decreases over time. We explore stability is- short many of the problems in machine learning could sues related to the o®-policy nature of memory-guided be solved or simpli¯ed through the life-long learning exploration and compare memory-guided exploration to paradigm. soft transfer and direct transfer in three di®erent envi- ronments. For any life-long learning system to succeed it will be important for an agent to be able to transfer informa- tion successfully between tasks. Task transfer requires the agent to automatically determine which features from previous tasks are signi¯cant to the new task to 1 Introduction be learned, and which are not. Appropriate task trans- fer is a complex problem and it lies at the very heart of life-long learning. This paper focuses on task transfer There are several major problems that have been en- in reinforcement learning, speci¯cally in Q-learning. countered by researchers as they have attempted to cre- ate intelligent agents. These problems mostly center on the availability of good data, training time, and over- ¯tting. 2 Current Transfer Methods [12] has identi¯ed the following four major machine If the agent's actuators and sensors remain constant, learning bottlenecks: knowledge (a need for accu- there are two situations that could be encountered rate information and models), engineering (informa- when attempting task transfer. Either the environ- tion must be made computer accessible), tractability ment will have changed, or the reward structure will (complexity of robot domains), and precision (impre- have changed [12]. If the environment has changed, cise sensors and actuators cause di®erences between the the state transition probabilities will no longer be the real world and models, simulators, or plans). same. Alternately if the reward structure has changed, the state transition probabilities will be the same but methods for task transfer in Q-learning that we devel- the reward received for taking those transitions will oped include memory-guided exploration [6] and what have changed. Combinations of these two situations we call \soft transfer." We discuss these three methods can also be encountered. If the agent itself changes, and their applications in three di®erent environments. then the actual state space can grow or contract (if preceptors are added or removed), or the agent's ac- 2.1.1 Direct Transfer. One simple way to transfer tion space can grow or contract (if actuators are added information from one Q-learning task to another is by or removed). using the Q-values from the ¯rst task to initialize the Q- values of the second task, then allowing normal updates Task transfer mechanisms can be thought of as algo- to adjust to any di®erences between the two tasks. rithms that 1) attempt to determine invariants among all tasks, 2) generalize invariant information among If the problems are too dissimilar the agent will spend various groups of tasks, 3) determine group member- too much time unlearning the incorrect portion of the ship of new tasks, 4) determine task speci¯c informa- policy causing the agent to learn the new task slower tion, and 5) allow specialization in those areas where than learning it from scratch [6][1]. Also as the agent the tasks di®er. In general such algorithms should allow unlearns this incorrect information it often unlearns the agent to learn a new task with greater speed than correct information as well. This usually happens as γ learning that task from scratch. When encountering discounts Q-values along the path to the goal and be- a task unlike any the agent has encountered before we fore the reward from the new goal has back-propagated should allow the agent to take slightly longer than when su±ciently to overcome this situation. We call this the learning from scratch. This is understandable because unlearning problem. For direct transfer to function well we would expect the agent to explore areas of similarity the two tasks must be su±ciently similar, which limits between the current and past tasks ¯rst. However we the applicability of the method. believe that any appropriate task transfer mechanism should be guaranteed to eventually learn any learnable 2.1.2 Soft Transfer. One way to make direct trans- task, regardless of the amount of incorrect or irrelevant fer more robust to di®erences in the past task is through prior information given to the agent. soft transfer. Soft transfer preserves the current pol- icy while softening the Q-values making them easier 2.1 Q-learning to change. This is a similar idea to that of avoiding Q-learning is a reinforcement learning method where over¯tting in many other machine learning problems. the agent stores the expected discounted reward for This is accomplished by initializing the Q-values using performing action a in state s Q(st; at) updated by a weighted average between the old Q-values and the standard tabula rasa I thus: ¢Q(st; at) = ®(R(st; at) + γmaxaQ(st+1; a)); new ¡ old where R is the reward and t is the time step, ® is the Q0 (s; a) = (1 W )I + W (QF (s; a)); learning rate and γ is the discount factor. Q-values are typically initialized to some constant value Q(s; a) = I. where Q0 is the initial Q-value, and QF is the ¯nal Q- value. If W = 1 this is the same as direct transfer and Some methods of incorporating prior knowledge in Q- if W = 0 then agent learns from scratch. By picking learning have involved building a model of the envi- 0 < W < 1 the agent can unlearn the incorrect por- ronment [7], biasing the inputs [9] or changing other tions of its policy easier. Care needs to be taken to aspects of a function approximator [4]. [8] suggested set this parameter appropriately. If W is too high the that transfer of information from one task to another in agent will spend too much time unlearning the incor- Q-learning can be accomplished by stringing together rect information in the past task, if it is too low the solutions to elemental sequential sub tasks. Unfortu- agent will loose useful information. nately this requires design intervention.1 to determine each elemental task. 2.1.3 Memory-guided Exploration. Memory- A common model free task transfer method in Q- guided exploration stores two or more sets of Q-values old new learning is to use the Q-values from the ¯rst task to Q (s; a) and Q (s; a). One set of Q-values rep- initialize the Q-values of the second task. This method resents the agent's current experience in the new is called direct transfer of Q-values. Other model free task. The other set of Q-values represents the stored memory of a past task. The old Q-values are never 1manual intervention by the designer of the system changed, preventing the accidental loss of pertinent information. The new Q-values are updated normally leaf nodes, and the agent's task is to ¯nd it. The agent as the agent explores its environment, reducing the receives a reward of +1 if it ¯nds the goal and a reward time necessary to \unlearn" the incorrect portions of of 0 if the agent terminates in a non-goal state. Once the policy [6]. the agent reaches a leaf node the task begins over, and the agent is placed at the top of the tree. The transi- A process similar to memory-guided exploration was tions are deterministic. The tasks varied in depth d and independently developed by [3].
Details
-
File Typepdf
-
Upload Time-
-
Content LanguagesEnglish
-
Upload UserAnonymous/Not logged-in
-
File Pages6 Page
-
File Size-