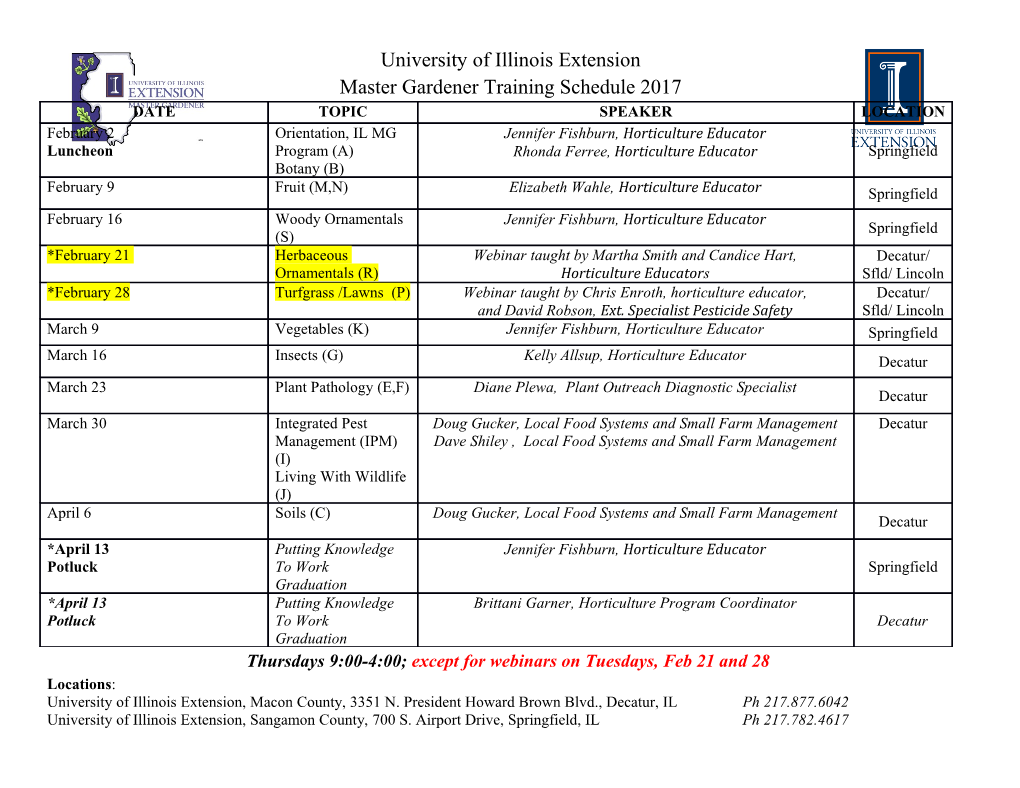
Received: 31 March 2020 Accepted: 23 June 2020 Published online: 17 September 2020 DOI: 10.1002/csc2.20255 Crop Science ORIGINAL RESEARCH ARTICLE Crop Breeding & Genetics Single nucleotide polymorphism calling and imputation strategies for cost-effective genotyping in a tropical maize breeding program Amanda Avelar de Oliveira1 Lauro José Moreira Guimarães2 Claudia Teixeira Guimarães2 Paulo Evaristo de Oliveira Guimarães2 Marcos de Oliveira Pinto2 Maria Marta Pastina2 Gabriel Rodrigues Alves Margarido1 1 “Luiz de Queiroz” College of Agriculture, Department of Genetics, Abstract University of São Paulo, Piracicaba, SP Genotyping-by-sequencing (GBS) datasets typically feature high rates of miss- 13418–900, Brazil ingness and heterozygote undercalling, prompting the use of data imputation. 2 Embrapa Milho e Sorgo, Sete Lagoas, We compared the accuracy of four imputation methods—NPUTE, Beagle, k- MG 35701–970, Brazil nearest neighbors imputation (KNNI), and fast inbreed line library imputation Correspondence (FILLIN)—using GBS data of maize (Zea mays L.) inbred lines, genotyped using Gabriel Rodrigues Alves Margarido, Uni- versity of São Paulo, “Luiz de Queiroz” different multiplexing levels. Two strategies for SNP-calling and genotype impu- College of Agriculture, Department of tation were evaluated. First, only lines genotyped through 96-plex were used for Genetics, Piracicaba, SP 13418–900, Brazil. single nucleotide polymorphism (SNP) discovery, whereas both 96- and 384-plex Email: [email protected] Maria Marta Pastina, Embrapa Milho e were simultaneously used in the second strategy. In the first genotype impu- Sorgo, Sete Lagoas, MG 35701–970, Brazil. tation strategy, only the 96-plex lines were imputed, then the remaining lines Email: [email protected] were appended (96-plex-imputed plus 384-plex) and then imputed. In the sec- Assigned to Associate Editor Timothy ond imputation strategy, we jointly imputed both datasets. We also investigated Beissinger. the impacts of including heterozygous genotypes and distinct rates of missing genotypes per locus. The different SNP-calling strategies and percentage of miss- Funding information Embrapa (Brazilian Agricultural Research ing data did not substantially affect the imputation accuracy. However, the dif- Corporation); CAPES (Coordenação de ferent imputation strategies showed a substantial effect. Generally, imputations Aperfeiçoamento de Pessoal de Nível Supe- rior); CNPq (Conselho Nacional de Desen- were less accurate for heterozygotes. The scenario 96-plex-imputed plus 384- volvimento Científico e Tecnológico); plex showed accuracies similar to the 96-plex scenario. Beagle and NPUTE pro- FAPEMIG (Fundação de Amparo à duced the highest accuracies. Our results indicate that combining SNP-calling Pesquisa de Minas Gerais) and imputation strategies can enhance genotyping in a cost-effective manner, resulting in higher imputation accuracies. 1 INTRODUCTION Abbreviations: FILLIN, fast inbreed line library imputation; GBS, genotyping-by-sequencing; InDel, insertion–deletion; KNNI, k-nearest The emergence of next-generation sequencing technol- neighbors imputation; MAF, minor allele frequency; SNP, single ogy presented the possibility of obtaining molecular nucleotide polymorphism. © 2020 The Authors. Crop Science © 2020 Crop Science Society of America 3066 wileyonlinelibrary.com/journal/csc2 Crop Science. 2020;60:3066–3082. DE OLIVEIRA et al. Crop Science 3067 markers densely distributed across the genome using high- throughput techniques such as GBS (Elshire et al., 2011). Core ideas Making use of these genome-wide genotyping platforms, ∙ genomic selection and genome-wide association studies Combining SNP-calling and imputation strate- offer great potential to accelerate and enhance selec- gies can enhance cost-effective genotyping ∙ tion efficiency of plant breeding programs (Chang, Toghi- SNP-calling strategies and percentage of miss- ani, Ling, Aggrey, & Rekaya, 2018; Desta & Ortiz, 2014; ing data did not affect the imputation accuracy ∙ Dias et al., 2018; Faville et al., 2018; Gerard, Kobiljski, Beagle and NPUTE produced the highest accu- Lohwasser, Börner, & Simón, 2018; Haile et al., 2018; racies Kayondo et al., 2018). However, the costs of high-density genotyping for large numbers of individuals are still infea- sible, representing a barrier to a more widespread adop- tion of these tools. Because the accuracy of genomic selec- However, to the best of our knowledge, no studies have tion and power of association studies usually increase with yet empirically investigated the combined use of SNP- increasing numbers of individuals and density of mark- calling and imputation strategies to improve GBS data ers, low-cost genotyping strategies have to be adopted to quality. address resource limitations (Cericola et al., 2018;Han There are several imputation methods available but et al., 2018; Jacobson, Lian, Zhong, & Bernardo, 2015). most of them were developed for humans (Browning & The adoption of genomic selection in a maize breeding Yu, 2009; Howie et al., 2009;Liu,Li,Wang,&Li,2013). program allows breeders to genotype elite lines and to pre- However, humans are highly heterozygous, obligate out- dict the performance of all possible hybrids even if they crossers, show little inbreeding, and much less structural are not phenotypically evaluated. This strategy reduces the variation than that observed in crop plants. These factors costs and labor involved in field trials and can increase make the imputation methods designed for humans not genetic gains. In any case, cost-effective genotyping is necessarily optimized for use in crop systems. For this crucial. Key features of GBS data are the high rates of reason, it is worthwhile to compare different imputation missingness, heterozygote undercalling, and nonuniform methods, which may or may not allow for heterozygous distribution of sequence reads, which vary according to genotypes. Situations in breeding programs where there the kind of population and multiplexing level (Beissinger are genotypic datasets with varying levels of multiplex- et al., 2013). Several studies have reported the efficiency ing and heterozygosity are increasingly common. There is of imputing missing data using different methods and therefore scientific and practical interest in gaining knowl- strategies (Bouwman, Hickey, Calus, & Veerkamp, 2014; edge about how to better explore such datasets in order to Cleveland, Hickey, & Kinghorn, 2011; Gonen et al., 2018; achieve high imputation accuracies. Hickey, Crossa, Babu, & De Los Campos, 2012; Howie, In this paper, we compared the imputation accuracy Donnelly, & Marchini, 2009; Nazzicari, Biscarini, Cozzi, of four imputation methods: NPUTE (Roberts et al., Brummer, & Annicchiarico, 2016; Swarts et al., 2014). An 2007), Beagle (Browning & Browning, 2007), KNNI effective strategy involves genotyping some of the individu- (Troyanskaya et al., 2001), and FILLIN (Swarts et al., als at higher marker density, then using these high-density 2014), which are well known algorithms implemented data to impute larger numbers of individuals genotyped in freely available software libraries. We imputed miss- at lower marker density. Genomic selection studies adopt- ing genotypes from GBS data of maize inbred lines ing this approach reported increases in the predictive accu- genotyped using different levels of multiplexing per racy (Gorjanc et al., 2017a; 2017b; Jacobson et al., 2015). For sequencing lane. We evaluated different SNP-calling species for which genotyping chips are available, as is the strategies in order to better explore the low and high case of economically important animals and some crops, multiplexing levels of our dataset. Because this dataset rep- combining data from high- and low-density SNP arrays is resents a panel of maize inbred lines mostly in final stages a cost-effective strategy (Gorjanc et al., 2017b; Hickey, Gor- of the breeding program, we expected that these lines were janc, Varshney, & Nettelblad, 2015; Jacobson et al., 2015). homozygous for the majority of loci. However, a few of When genotyping chips are not available or their use is those lines were in initial stages of the breeding program prohibitive, the GBS technology allows breeders to adjust and could thus have higher heterozygosity rates. Hence, we the amount of retrieved information and its cost in dif- also evaluated the impact of including heterozygous geno- ferent ways (Gorjanc et al., 2017b). For instance, choos- types on imputation accuracy. The main objective of this ing different restriction enzymes, regulating sequencing study was to evaluate different SNP-calling and imputa- depth, and the level of multiplexing (Deschamps, Llaca, tion strategies in a real maize breeding program scenario. &May,2012; Elshire et al., 2011; Poland & Rife, 2012). By doing so, we aimed to better explore the possibility of 3068 Crop Science DE OLIVEIRA et al. using both low and high multiplexing levels to deliver cost- plugin with all 1,060 lines. By doing so, all lines were effec- effective genotyping without compromising the imputa- tively genotyped but only for the loci detected in the first tion accuracy. set (Figure 1a, blue color). For SNP-calling strategy II, we ran the discovery and production SNP caller plugins com- bining both the high- and low-multiplexing sets of lines. 2 MATERIALS AND METHODS This strategy was likely to affect the number and qual- ity of discovered SNPs because we included lines geno- 2.1 Experimental data typed
Details
-
File Typepdf
-
Upload Time-
-
Content LanguagesEnglish
-
Upload UserAnonymous/Not logged-in
-
File Pages17 Page
-
File Size-