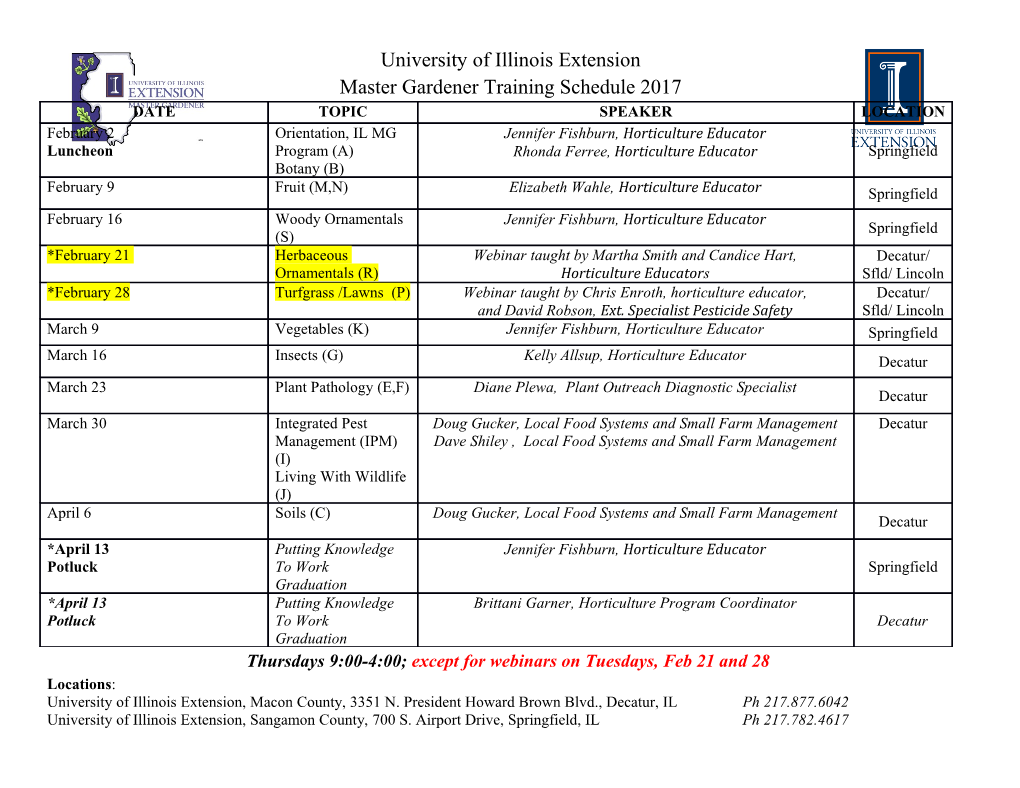
Guidelines on Homogenization 2020 edition WEATHER CLIMATE WATER CLIMATE WEATHER WMO-No. 1245 Guidelines on Homogenization 2020 edition WMO-No. 1245 EDITORIAL NOTE METEOTERM, the WMO terminology database, may be consulted at https://public.wmo.int/en/ meteoterm. Readers who copy hyperlinks by selecting them in the text should be aware that additional spaces may appear immediately following http://, https://, ftp://, mailto:, and after slashes (/), dashes (-), periods (.) and unbroken sequences of characters (letters and numbers). These spaces should be removed from the pasted URL. The correct URL is displayed when hovering over the link or when clicking on the link and then copying it from the browser. WMO-No. 1245 © World Meteorological Organization, 2020 The right of publication in print, electronic and any other form and in any language is reserved by WMO. Short extracts from WMO publications may be reproduced without authorization, provided that the complete source is clearly indicated. Editorial correspondence and requests to publish, reproduce or translate this publication in part or in whole should be addressed to: Chair, Publications Board World Meteorological Organization (WMO) 7 bis, avenue de la Paix Tel.: +41 (0) 22 730 84 03 P.O. Box 2300 Fax: +41 (0) 22 730 81 17 CH-1211 Geneva 2, Switzerland Email: [email protected] ISBN 978-92-63-11245-3 NOTE The designations employed in WMO publications and the presentation of material in this publication do not imply the expression of any opinion whatsoever on the part of WMO concerning the legal status of any country, territory, city or area, or of its authorities, or concerning the delimitation of its frontiers or boundaries. The mention of specific companies or products does not imply that they are endorsed or recommended by WMO in preference to others of a similar nature which are not mentioned or advertised. CONTENTS Page ACKNOWLEDGEMENTS . v INTRODUCTION . 1 CHAPTER 1 . PREREQUISITES . 3 1.1 Engagement with observing station network managers ................... 4 1.2 Parallel measurements. 4 1.3 Data rescue ......................................................... 5 1.4 Quality control ...................................................... 5 1.5 Station history and metadata .......................................... 6 1.5.1 What are metadata?. 6 1.5.2 The value of metadata and their limitations ..................... 6 1.5.3 Station identifiers. 7 1.5.4 Formats and accessibility of metadata .......................... 8 1.6 Training ............................................................ 8 CHAPTER 2 . HOMOGENIZATION PRACTICE . 9 2.1 Physical and statistical homogenization ................................. 10 2.2 Selecting the data to be homogenized .................................. 11 2.2.1 Which stations should be selected? ............................ 11 2.2.2 How to prepare data for homogenization ...................... 12 2.3 Statistical detection or determination of change points .................... 13 2.3.1 Incorporating metadata in statistical tests ...................... 13 2.4 Reference series ..................................................... 15 2.4.1 Overlap ................................................... 17 2.4.2 Noise reduction ............................................ 17 2.4.3 Inhomogeneities in the reference ............................. 17 2.4.4 References with similar climate signals ......................... 18 2.4.5 Using references ............................................ 19 2.5 Options if no useful reference station exists .............................. 19 2.6 Statistical adjustments ................................................ 21 2.7 Data review and multiple rounds of homogenization ...................... 22 2.8 Documentation ...................................................... 24 2.9 Operational maintenance of a homogenized dataset ...................... 24 2.10 Network-wide issues and options for dealing with them ................... 25 2.11 Specific challenges for multinational datasets ............................ 26 2.12 Conclusion: Good practices for homogenization. 27 CHAPTER 3 . SELECTING STATISTICAL HOMOGENIZATION SOFTWARE . 29 3.1 Statistical homogenization packages .................................... 29 3.2 Performance of statistical homogenization methods ...................... 33 3.2.1 Theoretical principles ....................................... 33 3.2.2 Numerical studies .......................................... 34 3.3 Automated and manual methods. 36 3.4 Use cases ........................................................... 36 CHAPTER 4 . HISTORY OF HOMOGENIZATION . 38 4.1 Detection and adjustment ............................................ 38 4.2 Reference series ..................................................... 38 4.3 Modern developments ............................................... 38 CHAPTER 5 . THEORETICAL BACKGROUND OF HOMOGENIZATION . 40 5.1 General structure of the additive spatio-temporal models .................. 40 5.2 Methodology for comparison of series in the case of an additive model ...... 42 5.3 Methodology for breakpoint (changepoint) detection ..................... 43 5.3.1 Breakpoint detection based on maximum likelihood estimation .... 43 5.3.2 Breakpoint detection based on hypothesis testing ............... 43 5.3.3 Attribution of the detected breakpoints for the candidate series .... 44 iv GUIDELINES ON HOMOGENIZATION Page 5.4 Methodology for adjustment of series ................................... 44 5.5 Possibilities for evaluation and validation of methods ...................... 44 GLOSSARY . 46 REFERENCES . 48 SUGGESTED READING . 53 ACKNOWLEDGEMENTS This is an updated version of Guidelines on Climate Metadata and Homogenization (WMO/TD No. 1186). It was prepared by the Task Team on Homogenization of the Commission for Climatology. We thank the following people for their outstanding contributions to this publication: Victor Venema (lead) Meteorological Institute, University of Bonn, Germany Blair Trewin Bureau of Meteorology, Melbourne, Australia Xiaolan L. Wang Climate Research Division, Science and Technology Branch, Environment and Climate Change Canada, Toronto, Ontario, Canada Tamás Szentimrey Varimax Limited Partnership, Budapest, Hungary Monika Lakatos Hungarian Meteorological Service, Climate Division, Hungary Enric Aguilar Rovira i Virgili University, Tarragona, Spain Ingeborg Auer Zentralanstalt für Meteorologie und Geodynamik (ZAMG), Vienna, Austria Jose A. Guijarro State Meteorological Agency (AEMET), Spain Matthew Menne National Oceanic and Atmospheric Administration (NOAA), National Centers for Environmental Information, Dataset Section, Asheville, North Carolina, United States Clara Oria Servicio Nacional de Meteorología e Hidrología (SENAMHI) del Perú, Peru Wilfrid Direction de la météorologie, Brazzaville, Congo S. R. L. Louamba Ghulam Rasul Pakistan Meteorological Department, Islamabad, Pakistan Further contributions have been made by: Athanassios Argiriou Greece Peer Hechler WMO Gregor Vertačnik Slovenia Yizhak Yosef Israel INTRODUCTION High-quality, homogeneous time series data are essential to analyse climate variability and change. Homogenization aims to make data “homogeneous”. This word derives from the ancient Greek and means “of the same nature”. Unfortunately, most long-term raw climate time series do not fulfil this principle and are internally inhomogeneous, that is not of the same nature, and are therefore unsuitable for statistical climate change analysis. Non-climatic factors such as change in the circumstances of the measurements can substantially affect measured values and cause distortions in the statistical behaviour of the time series. The impact of these distortions can be comparable to that of climate change and may lead to erroneous conclusions. Each data value arriving at the computer of a user is the result of a series of consecutive procedures: a set of instruments is deployed in a location, the value is measured, recorded, undergoes transformations and is included in a database, then it is transmitted to the intermediate and final users. With regard to long-term time series data, some of these procedures may have been altered over the years. In a temperature time series starting in the late nineteenth century, the thermometer has most likely been replaced several times or even substituted with an electronic sensor in recent decades. The shelter has probably changed from an open stand to a Stevenson screen and then possibly to a multi-plate screen if the station has been automated. Around the middle of the twentieth century, many observation stations were moved to airports to service the growing demand for civil aviation. If the station remained at the same location, the surroundings have probably been altered. For example, if the station was deployed in the outskirts of a village 100 years ago, today, it might be surrounded by buildings. Perhaps due to land use changes or other practical reasons, the station has been relocated to a more convenient place in the suburban area. If we combined the observations made in such different periods, they would obviously not be comparable. It is rare, although not unknown, for a climate data record of 100 years or more to be truly homogeneous. For example, in the Australian ACORN-SAT temperature dataset, only 2 out of 112 stations (both starting in the 1940s) were found to be homogeneous for both maximum and minimum temperatures, and in Europe, the period between two detected breaks
Details
-
File Typepdf
-
Upload Time-
-
Content LanguagesEnglish
-
Upload UserAnonymous/Not logged-in
-
File Pages63 Page
-
File Size-