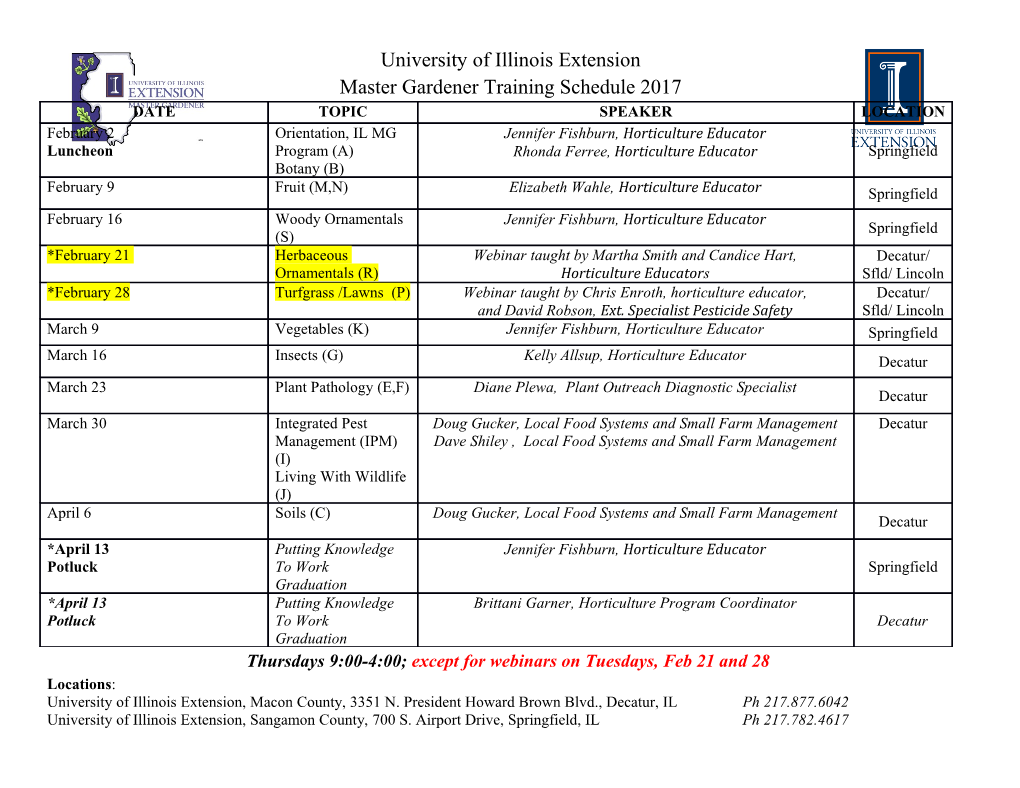
Large-Scale Statistics with MonetDB and R Hannes Mühleisen DAMDID 2015, 2015-10-13 About Me • Postdoc at CWI Database architectures since 2012 • Amsterdam is nice. We have open positions. • Special interest in data management for statistical analysis • Various research & software projects in this space 2 Outline • Column Store / MonetDB Introduction • Connecting R and MonetDB • Advanced Topics • “R as a Query Language” • “Capturing The Laws of Data Nature” 3 Column Stores / MonetDB Introduction 4 Postgres, Oracle, DB2, etc.: Conceptional class speed flux NX 1 3 Constitution 1 8 Galaxy 1 3 Defiant 1 6 Intrepid 1 1 Physical (on Disk) NX 1 3 Constitution 1 8 Galaxy 1 3 Defiant 1 6 Intrepid 1 1 5 Column Store: class speed flux Compression! NX 1 3 Constitution 1 8 Galaxy 1 3 Defiant 1 6 Intrepid 1 1 NX Constitution Galaxy Defiant Intrepid 1 1 1 1 1 3 8 3 6 1 6 What is MonetDB? • Strict columnar architecture OLAP RDBMS (SQL) • Started by Martin Kersten and Peter Boncz ~1994 • Free & Open Open source, active development ongoing • www.monetdb.org Peter A. Boncz, Martin L. Kersten, and Stefan Manegold. 2008. Breaking the memory wall in MonetDB. Communications of the ACM 51, 12 (December 2008), 77-85. DOI=10.1145/1409360.1409380 7 MonetDB today • Expanded C code • MAL “DB assembly” & optimisers • SQL to MAL compiler • Memory-Mapped files • Automatic indexing 8 Some MAL • Optimisers run on MAL code • Efficient Column-at-a-time implementations EXPLAIN SELECT * FROM mtcars; | X_2 := sql.mvc(); | | X_3:bat[:oid,:oid] := sql.tid(X_2,"sys","mtcars"); | | X_6:bat[:oid,:dbl] := sql.bind(X_2,"sys","mtcars","mpg",0); | | (X_9,r1_9) := sql.bind(X_2,"sys","mtcars","mpg",2); | | X_12:bat[:oid,:dbl] := sql.bind(X_2,"sys","mtcars","mpg",1); | | X_14 := sql.delta(X_6,X_9,r1_9,X_12); | | X_15 := algebra.leftfetchjoin(X_3,X_14); | | X_16:bat[:oid,:dbl] := sql.bind(X_2,"sys","mtcars","cyl",0); | | (X_18,r1_18) := sql.bind(X_2,"sys","mtcars","cyl",2); | | X_20:bat[:oid,:dbl] := sql.bind(X_2,"sys","mtcars","cyl",1); | | X_21 := sql.delta(X_16,X_18,r1_18,X_20); | | X_22 := algebra.leftfetchjoin(X_3,X_21); | 9 “Invisible JOIN” Performance... TPC−H SF−100 Hot runs ● ● ● ● ● ● ● ● ● ● ● ● ● ● ● ● ● ● ● ● ● ● ● ● ● 100.00 ● ● ● ● ● ● ● ● ● ● ● 1.00 ● ● Average time (s) Average 0.01 ● monetdb ● postgres Query log! 10 But statistics with SQL? 11 Integrate not Reinvent Statistical Toolkits ? Flexibility Data Management 2 Systems Efficiency 12 Collect data Filter, Load data transform & Analyze & Plot aggregate data Publish paper 13 Collect data Growing Filter, Load data transform & Analyze & Plot aggregate data Not really Analysis features Publish paper 14 Collect data Statistical Toolkit Filter, Load data transform & Analyze & Plot aggregate data Data Management 2 System Publish paper 15 Statistical Toolkit Filter, transform & Analyze & Plot aggregate data Data Management 2 System 16 Bridge the Gap • JDB + Native operators, lazy evaluation • JDB + Cheap data transfer 17 18 Previous Work MonetDB.R connector on CRAN since 2013 Embedded R in MonetDB Part of MonetDB since 2014 MonetDBLite for R Preview release available 19 Also… Embedded Python/NumPy Next MonetDB release 20 MonetDB.R connector Hannes Mühleisen and Thomas Lumley: Best of Both Worlds – Relational Databases and Statistics 25th International Conference on Scientific and Statistical Database Management (SSDBM2013) 21 DBI • DBI is for R what JDBC is for Java • Low-level interface to talk to SQL databases • Drivers available for most relational databases • Typically socket connection between R and DB df <- dbGetQuery(con, "SELECT * FROM table") 22 DBI • Works, but (generally) • Serialising/Unserialising large datasets is slow • Data ingest is slow • SQL knowledge required 23 dplyr • Data reorganisation package in “Hadleyverse” • Works with data.frame, data.table, SQL DBs • Maps relational operations (selection, projection, join, grouping etc.) to native R operators • Lazy evaluation, call chaining • MonetDB.R includes a dplyr compatibility layer 24 dplyr In R: ni <- select(n, first_name, last_name, race_desc, sex, birth_age) ow <- filter(ni, as.integer(birth_age) > 66, sex=="MALE", race_desc == “WHITE") print(ow) Generated: SELECT "first_name" AS "first_name", "last_name" AS "last_name", "race_desc" AS "race_desc", "sex" AS "sex", "birth_age" AS "birth_age" FROM "ncvoter" WHERE CAST("birth_age" AS INTEGER) > 66.0 AND "sex" = 'MALE' AND "race_desc" = 'WHITE' LIMIT 10 25 dplyr • Better, but • Most (All) R packages cannot work with dplyr tables, so at some point data needs to be transferred. • What if this dataset is large? 26 Embedded R in MonetDB 27 Relationally Integrated π Statistical analysis + as operators in relational queries ⨝ σ σ 28 Table-producing CREATE FUNCTION rapi01(i INTEGER) RETURNS TABLE (i INTEGER, d DOUBLE) LANGUAGE R { data.frame(i=seq(1,i),d=42.0) }; SELECT i,d FROM rapi01(42) AS r WHERE i>40; 29 π Transformations CREATE FUNCTION rapi02 (i INTEGER, j INTEGER, z INTEGER) RETURNS INTEGER LANGUAGE R { i*sum(j)*z }; SELECT rapi02(i,j,2) AS r02 FROM rval; 30 σ Filtering CREATE FUNCTION rapi03(i INTEGER, z INTEGER) RETURNS BOOLEAN LANGUAGE R { i>z }; SELECT * FROM rval WHERE rapi03(i,2); 31 Aggregation CREATE AGGREGATE kmeans(data FLOAT, ncluster INTEGER) RETURNS INTEGER LANGUAGE R { kmeans(data,ncluster)$cluster }; SELECT cluster FROM (SELECT MIN(x) AS minx, MAX(x) AS maxx, kmeans(x,5) AS cluster FROM xdata GROUP BY cluster) as cdata ORDER BY cluster; 32 Performance… R−col ● MonetDB ● 40 R−full ● 30 PL/R−tuned ● 20 Time (s) RInt PL/R−naive ● 10 ● ● ● ● ● ● 0 ● ● ● ● 1 K 10 K 100 K 1 M 10 M 100 M Rows (log) 33 Code Shipping > rf.fit <- randomForest(income~., data=training, mtry=2, ntree=10) > predictions <- mdbapply(con, “t1", function(d) { p <- predict(rf.fit, type=“prob", newdata=d)[,2] p[p > .9] }) MonetDB.R 1.0.0, soon 34 MonetDBLite 35 MonetDBLite • Socket serialization/deserialization for client/server protocol is slow for large result sets. • Too slow for many machine learning problems! • Running a database server is cumbersome and overkill for a single R client • Solution: Run entire database inside the R process • Only copy ingest data / query results around in memory, fast • Same interface as MonetDB.R, DBI/dplyr https://goo.gl/jelaOy 36 Quick Benchmark lineitem table with 10M rows, SELECT * FROM lineitem 18 17.2 s 14 s 9 5 0.4 s 0 Old (MAPI Socket) MonetDBLite 37 Zero-Copy Jonathan Lajus and Hannes Mühleisen: Efficient Data Management and Statistics with Zero-Copy Integration 26th International Conference on Scientific and Statistical Database Management (SSDBM2014) 38 R SEXP Array SEXP Header 42 43 44 ... Reference MonetDB BAT head Column 0 1 2 ... Descriptor BAT Arrays Reference Descriptor Column ... tail Descriptor 42 43 44 39 Dress-up BAT MonetDB Descriptor Reference Column tail Descriptor R SEXP Header 42 43 44 ... Reference 40 + Garbage Collection Fun Advanced Topics 41 R as a Query Language Hannes Mühleisen, Alex Bertram and Maarten-Jan Kallen: Relational Optimizations for Statistical Analysis, Journal of Statistical Software (under review) 42 What is Renjin? • R on the JVM • Compatibility is paramount, not just academic exercise (e.g. automatic Fortran/C translations) • R anywhere on any data format (e.g. Cloud environments) • Increased performance through lazy evaluation, parallel execution, … • Easy to plug any Java code into R analysis, easy to plug Renjin into java projects 43 Abstraction in Renjin > a <- 1:10^9 > a[1000000] <- NA #harr harr > system.time(print(anyNA(a)))[[3]] [1] TRUE [1] 0.001 > system.time(print(any(is.na(a))))[[3]] [1] TRUE [1] 2.23 GNU R > system.time(print(any(is.na(a))))[[3]] [1] TRUE [1] 0.05 Renjin 44 “R as a query language” dplyr::, data.table:: subset() [ • Observation 1: Lots of data wrangling happening in R scripts merge() $ aggregate() 45 “R as a query language” • Observation 2: Things get slow quickly as vectors get longer • Lots of optimisation opportunities, but how? • State of the art: Tactical optimisation/Band aids 46 “R as a query language” • Proposal: Treat R scripts as declaration of intent (not as a procedural contract written in blood) • Then we can optimise strategically! 47 Rule-based query optimisation 48 Optimisations • Selection Pushdown • Data-parallel scheduling • Function specialisation/vectorisation • Common expression elimination/caching • Redundant computation elimination 49 Static analysis? 50 Deferred Evaluation / a <- 1:1000 min max b <- a + 42 c <- b[1:10] [ d <- min(c) / max(c) print(d) + a 42 51 Pushdown b <- factorial(a) c <- b[1:10] print(c) n=10 n=10 [ (subset) factorial n=1000 n=10 factorial [ (subset) n=1000 n=1000 a a 52 Pushdown 6 ●● GNU R 4 2 Execution Time (s) Time Execution ●● Renjin ●● 0 ●● ●● ●● 106 107 108 Dataset Size (elements, log scale) 53 Recycling for (i in 1:100) print((a[i] - min(a))/(max(a)-min(a))) / / - - - (cached) a[i] min max a[i] (cached) a 54 Recycling Renjin ●● 60 40 ●● Execution Time (s) Time Execution 20 GNU R ●● ●● ●● ●● 0 ●● Renjin + R. 106 107 108 Dataset Size (elements, log scale) 55 svymean agep <- svymean(~agep, svydsgn, se=TRUE) for(i in 1:ncol(wts)) { repmeans[i,]<-t(colSums(wts[,i]*x*pw)/ sum(pw*wts[,i])) } […] v<-crossprod(sweep(thetas,2, meantheta,"-")*sqrt(rscales))*scale 56 * svymean crossprod 0.2 * - [5] t rep repmeans 5 / / / / / / colSums sum sum colSums sum colSums sum colSums colSums sum colSums sum * * * * * * * * * * * rep * * * p * * 47512 wts[,2] wts[,3] wts[,4] x wts[,5] wts[,1] svymean * crossprod 0.2 * - [5] t repmeans rep / / / / / 5 / colSums (cached) colSums (cached) colSums (cached) colSums (cached) colSums (cached) colSums (cached) * * * * * * wts[,2] wts[,3] wts[,4] x wts[,5] wts[,1] (cached) 58 svymean ●● 100 Renjin −opt 75 50 GNU R ●● Execution Time (s) Time Execution 25 Renjin 1t ●● ●● ●● ●● ● ●● 0 ●● ● Renjin 47512 1060060 9093077 Dataset Size (elements, log scale) 59 Capturing the Laws of Data Nature Hannes Mühleisen, Martin Kersten and Stefan Manegold: Capturing the Laws of (Data) Nature, 7th Biennial Conference on Innovative Data Systems Research (CIDR), Jan.
Details
-
File Typepdf
-
Upload Time-
-
Content LanguagesEnglish
-
Upload UserAnonymous/Not logged-in
-
File Pages74 Page
-
File Size-