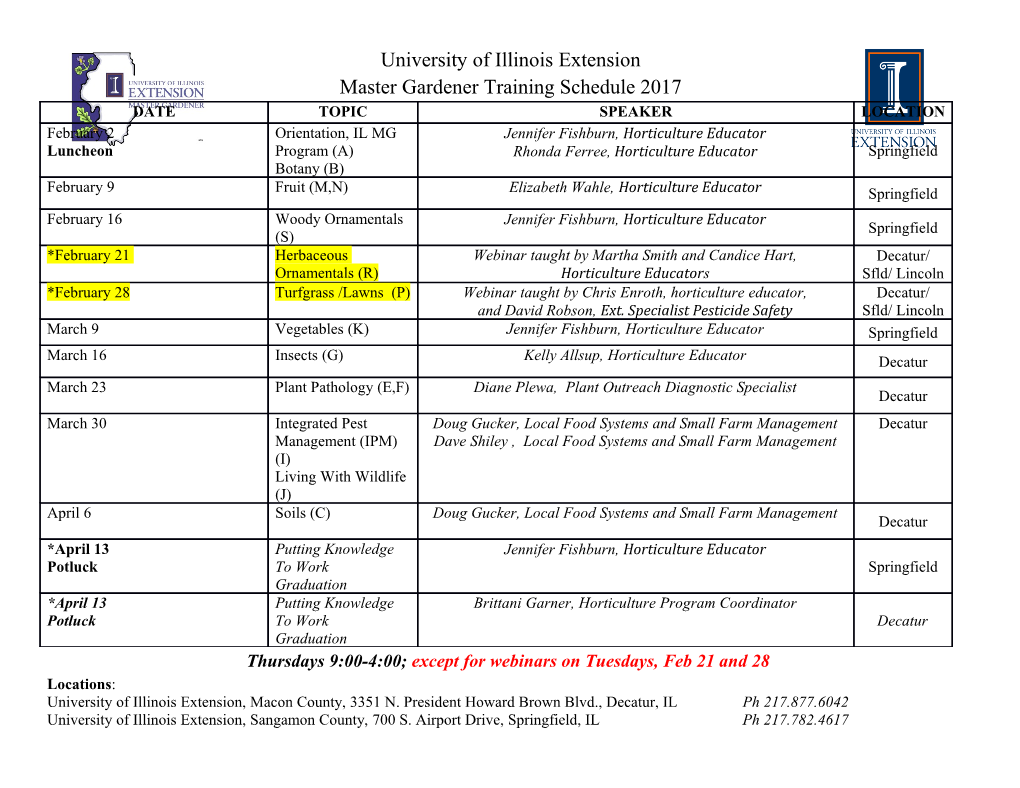
FARE2019 WINNERS Sorted By Institute Ling Zhang Postdoctoral Fellow CC Radiology/Imaging/PET and Neuroimaging Convolutional Invasion and Expansion Networks for Tumor Growth Prediction This study investigates the possibility of using deep learning to model and predict tumor growth, which has long been formulated by mathematical modeling. Tumor growth is associated with two fundamental processes: cell invasion and mass-effect. Medical imaging data provides non-invasive and in vivo- measurements of these underlying tumor physiological parameters over time. We propose a two- stream end-to-end convolutional neural network (ConvNet) to directly represent and learn the cell invasion and mass-effect from longitudinal medical images, and to predict the subsequent involvement regions of a tumor. The network architecture can easily be trained on population data and personalized to a target patient, unlike most previous mathematical modeling methods that fail to incorporate population data. Experiments are taken on a longitudinal pancreatic tumor data set with comparison with a state-of-the-art mathematical model-based approach. To the best of our knowledge, this is the first time to use learnable ConvNet models for explicitly capturing the two fundamental processes of tumor growth. The invasion network can make its prediction based on the metabolic rate, cell density and tumor boundary, all derived from the multi-model imaging data. Mass-effect â the mechanical force exerted by the growing tumor â can be approximated by the expansion/shrink motion (magnitude and orientation) of the tumor mass. This expansion/shrink cue is captured by optical flow computing, based on which the expansion network is trained to infer tumor growth. To exploit the inherent correlations among the invasion and expansion information, we study and evaluate three different network architectures, named: early-fusion, late-fusion, and end-to-end fusion. Our proposed ConvNet architectures can be both trained using population data and personalized to a target patient. The proposed ConvNets are tested on a NVIDIA TITAN X Pascal GPU of 12 GB of memory. Quantitative experiments on a pancreatic tumor data set show that the proposed method has a Dice coefficient of 86.8%, and RVD (relative volume difference) of 6.6%, substantially outperforms a state-of-the-art mathematical model-based approach (Dice=84.4%, RVD=13.9%). The proposed ConvNets requires ~ 5 mins for training and personalization, and 15 s for prediction per patient - significantly faster than the state-of-the-art model-based approach (~ 24 hrs â model personalization; 21 s â simulation). __________________________________________________________________________________ Laetitia Gorisse Visiting Fellow CC Biochemistry - General, Proteins, and Lipids IQGAP1 Binds The Axl Receptor Tyrosine Kinase And Inhibits Its Signaling IQGAP1 is a scaffold protein that integrates cell signaling pathways by binding several growth factor receptors and intracellular signaling molecules (Trends Cell Biol 2015; 25:171-184). The Axl tyrosine kinase receptor is important in hematopoiesis, the innate immune response, platelet aggregation, engulfment of apoptotic cells and cell survival (Cold Spring Harb Perspect Biol 2013; 5:a009076). Binding of growth arrest-specific protein 6 (Gas6) to immunoglobulin-like (Ig-like) domains of Axl activates intracellular signaling. However, the mechanism of inactivation of the Axl receptor is poorly understood. Here, we investigate the interaction between IQGAP1 and Axl. Using GST pulldown assays and immunoprecipitation from mammalian cell lysates, we found that IQGAP1 associates with Axl in cells. Analysis with multiple discrete fragments of each protein revealed that the binding is direct and mediated by the IQ domain of IQGAP1 and the Ig-like domains of Axl. We observed by proximity ligation assays that Axl:IQGAP1 complexes are located in the cytoplasm, and this interaction is reduced by 64% when cells are incubated with Gas6. Consistent with these findings, reducing IQGAP1 levels in cells by CRISPR/Cas9 enhanced the ability of Gas6 to stimulate both Axl phosphorylation and activation of Akt. Moreover, reduction of IQGAP1 in cells promoted Gas6-stimulated transcription of matrix metalloproteinase (MMP)-2, MMP-3, MMP-9 and urokinase-type plasminogen activator genes. Finally, we observed by confocal microscopy that IQGAP1 downregulates the heterodimerization of Axl with the epidermal growth factor receptor. In summary, we identify IQGAP1 as a previously undescribed suppressor of Axl. These findings differ from the previously reported functions for IQGAP1 as a scaffold that promotes signaling by growth factor receptors (J Biol Chem 2007; 292:3273-3289; J Biol Chem 2011; 286:29734-29747; J Biol Chem 2011; 286:15010-15021). Our data expand the repertoire of binding partners for both Axl and IQGAP1, and provide additional insight into the regulation of Axl function. __________________________________________________________________________________ Youbao Tang Postdoctoral Fellow CC Radiology/Imaging/PET and Neuroimaging Semi-Automatic RECIST Labeling on CT Scans with Cascaded Convolutional Neural Networks Response evaluation criteria in solid tumors (RECIST) is the standard measurement for tumor extent to evaluate treatment responses in cancer patients. The quality of RECIST annotations will affect the assessment result. To perform RECIST annotations, a radiologist first selects an axial image slice and then he or she measures the diameters of the longest axis and the orthogonal short axis. Using RECIST annotation faces two main challenges: 1) measuring tumor diameters requires a lot of professional knowledge and is time-consuming. 2) RECIST marks are often subjective and prone to inconsistency among different observers. However, consistency is critical in assessing actual lesion growth rates, which directly impacts patient treatment options. To overcome these problems, we propose a RECIST estimation method based on a cascaded convolutional neural network. Specifically, the stacked hourglass networks (SHN) is employed for RECIST estimation, where a relationship constraint loss is introduced to improve the estimation accuracy. Regardless of class, the lesion regions may have large variability in sizes, locations and orientations in different images. To make our method robust to these variations, the lesion region first needs to be normalized before feeding into SHN. The spatial transformer network is used for lesion region normalization, where a localization network is designed for lesion region and transformation parameter prediction. Thus, given a region of interest cropped using a bounding box roughly drawn by a radiologist, our method directly outputs RECIST annotations. As a result, our method is semi-automatic, drastically reducing annotation time while keeping the âhuman in the loopâ. In addition, our method can be readily made fully automatic as it can be trivially connected with any lesion localization framework. We train our system on a large scale lesion dataset where RECIST annotations are performed by multiple radiologists over a multi-year period. Experimental results compare our method to the multi-rater annotations in that dataset, plus annotations from two additional radiologists. The mean and standard deviation of inter-reader variation of long diameter are 3.40mm and 5.24mm, while those for the variation between our system and the manual annotations are 2.67mm and 3.95mm, which demonstrate that our system performs more stably and with less variability, suggesting that RECIST annotations can be reliably obtained with reduced labor and time. __________________________________________________________________________________ Kirill Gorshkov Postdoctoral Fellow NCATS Clinical and Translational Research, and Gene Therapy A novel high-throughput assay for Niemann-Pick disease type A small molecule therapeutics discovery Niemann-Pick disease type A (NPA) is a rare and fatal lysosomal storage disease caused by a deficiency of acid sphingomyelinase (ASM) that results in lysosomal sphingomyelin (SM) accumulation. There is no treatment available for NPA. In this study, we have developed a washless, 384-well plate, quantitative high-throughput screening (qHTS) fluorescence assay to identify compounds that alleviate the SM accumulation phenotype by restoring ASM function or other mechanisms. We first loaded NPA patient fibroblasts with green-fluorescent BODIPY-FL-labeled sphingomyelin, followed by treatment with drug compounds at 37 degrees C for 72 hours. The red-fluorescent LysoTracker dye and DRAQ5 nuclear dye were added at the end of the experiment one hour prior to fixation with Mirskyâs fixative, enabling the detection of individual cells using the Mirrorball fluorescence cytometer, which uses fluorometric microvolume assay technology to exclude background signal. The cellular imaging data consisting of cell count and fluorescent intensity measurements were analyzed using the Cellista software. Our primary and follow-up compound screen used wild-type fibroblasts as a control cell line. DMSO and delta tocopherol were used as negative and positive controls, respectively. We first screened the LOPAC drug library consisting of 1280 bioactive compounds producing 19 positive hits. The hit selection criteria consisted of greater than 30% reduction green fluorescence (decreased SM accumulation) without a decrease in cell counts. Seven hits clustered into two distinct classes targeting Receptor
Details
-
File Typepdf
-
Upload Time-
-
Content LanguagesEnglish
-
Upload UserAnonymous/Not logged-in
-
File Pages159 Page
-
File Size-