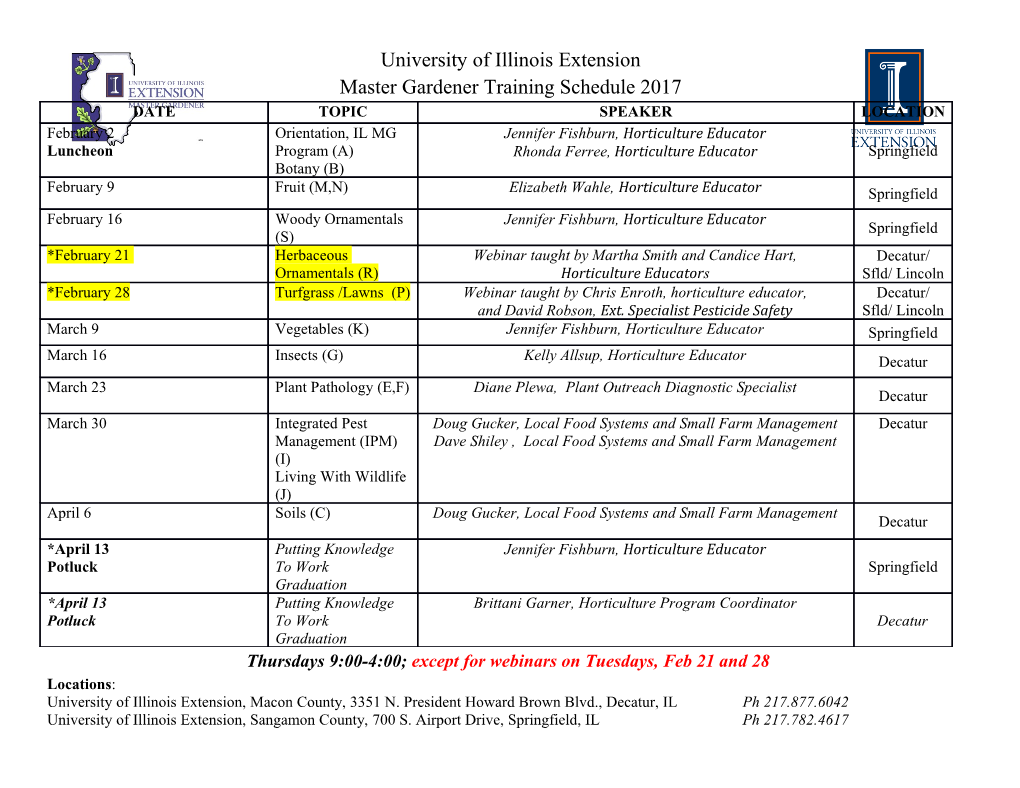
TWO-STAGE STOCHASTIC PROGRAMMING FOR ADAPTIVE INTERDISCIPINARY PAIN MANAGEMENT by NA WANG Presented to the Faculty of the Graduate School of The University of Texas at Arlington in Partial Fulfillment of the Requirements for the Degree of DOCTOR OF PHILOSOPHY THE UNIVERSITY OF TEXAS AT ARLINGTON May 2015 Copyright © by NA WANG 2015 All Rights Reserved ii Acknowledgements I would first like to express my deepest gratitude to my dissertation advisor Dr. Jay Rosenberger. I thank him for his outstanding guidance, invaluable support and encouragement during my Ph.D study. I also thank him for passing his knowledge in optimization area to me. I would like to thank my committee members, Dr. Chen, Dr. Gatchel and Dr. Zeng for their invaluable advice and support throughout this research. It’s a fortune to have such knowledgeable experts as my committee members. I would like to thank the UTSW Medical Center at Dallas for providing data. I would also like to thank friends at Center On Stochastic Modeling, Optimization, & Statistics for support and sharing their knowledge. In addition, I would like to thank my parents, Wenquan Wang and Sumei Yin, for their endless support and love. Finally, I would like to thank my husband and my son, Yan Wang and Andrew Wang. Thank my son for bringing so much fun to my life. Thank my husband for his love, encouragement and endless support. April 21, 2015 iii Abstract TWO-STAGE STOCHASTIC PROGRAMMING FOR ADAPTIVE INTERDISCIPLINARY PAIN MANAGEMENT Na Wang, PhD The University of Texas at Arlington, 2015 Supervising Professor: Jay Rosenberger Chronic pain is a global health problem. About 100 million adult Americans suffering from chronic pain, which costs $560 - $635 billion per year. The Eugene McDermott Center for Pain Management at the University of Texas Southwestern Medical Center at Dallas conducted a two-stage pain management program for patients who suffer from chronic pain. Data information such as patient’s parameter, past treatment, pain outcome at different evaluation point and so on has been collected by the McDermott Center. The University of Texas at Arlington is collaborating with the McDermott Center to find optimal treatment strategies for individual patients. A treatment strategy of the two-stage pain management program is the decision made at two different stages. For this two stage decision problem, it is formulated as a two- stage stochastic programming (2SP) model. The two-stage stochastic model incorporates outcome and state transition (system prediction) models as a part of its constraints. These system prediction models have the non-convex mixed integer nonlinear property. This research proposes a linear approximation method to approximate the non-convex nonlinear constraints by piecewise linear functions. By discretizing the continuous random variables in the linear approximation 2SP , an equivalent MILP model is obtained, which is then solved very quickly by branch and bound algorithm in a mature. iv An alternative approach to solve the original 2SP model is using the spatial branch and bound algorithm in a non-convex MINLP solver COUENNE to solve its equivalent deterministic model directly. However, this approach is computationally intensive. Within elapsed real time limit of 6 minutes that is considered as a reasonable waiting time for patients getting recommendations from the decision support system by the McDermott Center, COUENNE cannot find a solution for the MINLP problem. The policies generated by MILP models (MILP policies) and the policies generated by MINLP models (MINLP policies) are cross evaluated by two different evaluators, MILP evaluator and MINLP evaluator. The MILP evaluator shows that policies generated by MILP models could achieve the objectives better. However, MINLP evaluator could not evaluate the policies fairly because (1) the number of scenarios in the MINLP evaluator is not enough to simulate “real/true” situation and (2) the MINLP evaluator cannot find optimal solutions for most patients within elapsed time of 15 minutes. v Table of Contents Acknowledgements .............................................................................................................iii Abstract .............................................................................................................................. iv List of Illustrations .............................................................................................................. ix List of Tables ...................................................................................................................... xi Chapter 1 Introduction......................................................................................................... 1 1.1 Background ............................................................................................................... 1 1.2 Research Methodology Overview/Contribution ........................................................ 3 Chapter 2 Literature Review and Contribution .................................................................... 7 2.1 Overview of Pain and Its Impacts ............................................................................. 8 2.1.1 Outcome Measurements ................................................................................... 9 2.1.2 Treatment Options ........................................................................................... 10 2.2 Interdisciplinary Pain Management Program ......................................................... 11 2.3 Adaptive Treatment Strategies ............................................................................... 13 2.3.1 Experiment Design .......................................................................................... 14 2.3.1.1 The Multiphase Optimization Strategy ..................................................... 14 2.3.1.2 Sequential Multiple Assignment Randomized Trial ................................. 16 2.3.2 Issues of the Data from Observational Studies ............................................... 17 2.3.3 Algorithms for Optimizing Adaptive Treatment Strategies .............................. 18 2.3.3.1 Stochastic Dynamic Programming ........................................................... 18 2.3.3.2 Two-stage Stochastic (Mixed-) Integer Programming ............................. 21 2.3.3.3 Global Optimization on MILPs ................................................................. 26 2.3.3.4 Global Optimization on Non-convex MINLPs .......................................... 27 2.3.3.4.1 Symbolic Reformulation and Linearization ....................................... 28 2.3.3.4.2 Bounds Tigtening .............................................................................. 30 vi 2.3.3.4.3 Branching Techniques ...................................................................... 31 2.4 Contribution ............................................................................................................ 32 Chapter 3 Piecewise Linear Approximation Approach ..................................................... 34 3.1 Introduction ............................................................................................................. 34 3.1.1 Problem Description ........................................................................................ 34 3.1.2 Modeling Approaches ...................................................................................... 38 3.2 Model Formulation .................................................................................................. 39 3.3 Linear Approximation of Non-convex Nonlinear Function ...................................... 41 3.3.1 Refitting Two Continuous Variables Interaction Term ..................................... 47 3.4 Objective Function .................................................................................................. 51 3.4.1 Penalty Function for Pain Outcome................................................................. 51 3.4.2 Treatment Cost Function ................................................................................. 53 3.5 Feasibility Discussion and Induced Constraints ..................................................... 54 3.6 Treatment Interaction Restriction ........................................................................... 55 3.7 Extensive Form of 2SP Model ................................................................................ 56 3.8 Extensive Form of Linear Approximation 2SP Model ............................................. 56 Chapter 4 Implementation and Computation Results ....................................................... 58 4.1 2SP System Prediction Models .............................................................................. 59 4.2 Study on Parameters of 2SP Model ....................................................................... 63 4.2.1 Treatment Coefficient ρ ................................................................................... 64 4.2.2 Sampling Size and Evaluation Procedure ....................................................... 64 4.2.2.1 Evaluation Procedure ............................................................................... 65 4.2.2.2 Determination of Sampling Size .............................................................. 66 4.3 Treatment Usage Analysis ..................................................................................... 68 vii 4.4 Final Pain Outcome Comparison between MILP Model and Observed Data .............................................................................................................................
Details
-
File Typepdf
-
Upload Time-
-
Content LanguagesEnglish
-
Upload UserAnonymous/Not logged-in
-
File Pages126 Page
-
File Size-