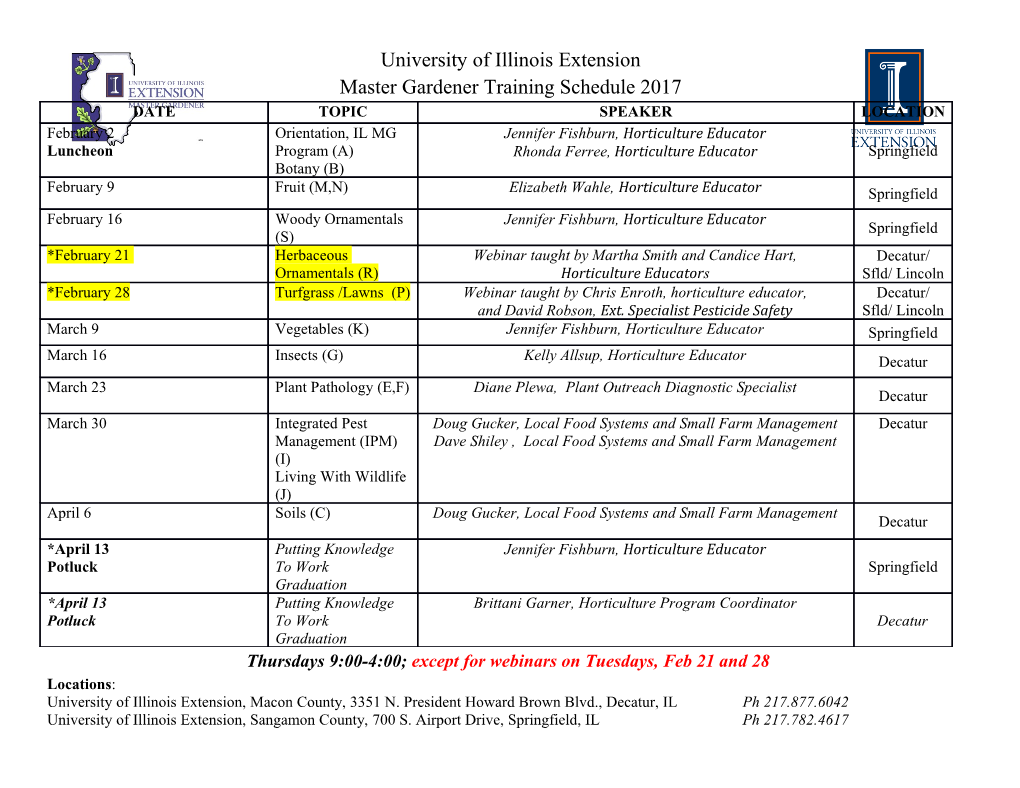
Synthese (2018) 195:2367–2386 https://doi.org/10.1007/s11229-017-1373-4 S.I. : PREDICTIVE BRAINS Predictive perceptual systems Nico Orlandi1 Received: 5 July 2016 / Accepted: 11 March 2017 / Published online: 20 March 2017 © Springer Science+Business Media Dordrecht 2017 Abstract This article attempts to clarify the commitments of a predictive cod- ing approach to perception. After summarizing predictive coding theory, the article addresses two questions. Is a predictive coding perceptual system also a Bayesian system? Is it a Kantian system? The article shows that the answer to these questions is negative. Keywords Predictive coding · Bayes · Perception · Kant · Expectations · Error · Free-energy What does a predictive coding approach to perception (or PCP) commit us to? This article suggests that predictive coding is primarily a theory of information transmis- sion. It is a theory that, in cognitive science, is geared at explaining how perceptual and sensory parts of the brain transmit information in a way that is efficient for the guidance of action. The article questions some additional commitments that the theory is assumed to have. The focus is on two such commitments. First, although PCP and Bayesian accounts of the mind and brain are usually presented hand in hand, I suggest that predictive coding and Bayesianism do not necessarily go together. A predictive coding perceptual system need not also be a Bayesian system. Second, some propo- nents of predictive coding interpret the theory as putting a Kantian and intellectualist gloss on mental processes (Gładziejewski 2016; Hohwy 2013). I explain, instead, This article develops themes from Orlandi 2014. I thank Jona Vance and Martin Thomson-Jones for assistance in understanding Bayesian decision theory. Needless to say, any mistake present in the paper is my own. I also thank two anonymous referees selected by Synthese, for constructive criticism on an earlier version of this article. B Nico Orlandi [email protected] 1 UC Santa Cruz, Santa Cruz, CA, USA 123 2368 Synthese (2018) 195:2367–2386 that predictive coding is fully compatible with embedded and ecological accounts of cognition. Why focus on these two themes? Doing so hopes to deliver a better understanding of PCP. But the themes are also interesting from a historical point of view. PCP was initially developed in cognitive science in the field of active vision, and it was thought to be good news for proponents of ecological and embodied understandings of perception (Rao and Ballard 1999). It is a curious development that it would be taken up by proponents of more intellectualist accounts. This article questions this type of uptake. In Sect. 1, I present what a predictive coding framework is, and describe how it may apply to perception. In Sect. 2, I argue that predictive perceptual systems are not necessarily Bayesian systems. In Sect. 3, I discuss the relationship between predictive coding and intellectualism, showing that the posits of predictive coding are in line with an ecological way of understanding the perceptual process. 1 Predictive coding and predictive perception Suppose that two people make a plan to meet in a certain location at a certain time. Once they make the plan, they decide that they will communicate prior to the meeting only if a problem arises. In doing this, the protagonists employ an efficient technique for transmitting information. They assume the meeting will take place, and communicate only if a change is needed. In other words, they communicate only if there is error in the original plan. This style of communication is the idea behind predictive or efficient coding. Predictive coding is originally a label for an information-transmission strategy employed, for example, in television (Harrison 1952; Oliver 1952). Television requires the continuous transmission of images. One of the problems faced by engineers in the image transmission field is the overload of the transmission medium. Transmission channels have limited capacity. To avoid overload, a strategy is to have a code that transmits information in a way that gets rid of redundancy. Consider transmitting a “still” image. We can either have a signal that transmits the still twice, risking overload, or we can have a signal that transmits the still once, and then communicates that there is no change in the image from the previous transmission (Harrison 1952, p. 766). Similarly, in transmitting a changing picture, we could have a signal that informs concerning every aspect of the changing picture, or we could have a signal that only informs concerning what changes from the initial image. This type of signal does not encode regions of the changed picture that are highly predictable given the past. This would be a predictive coding signal. A predictive code informs about error or what is unexpected, rather than what is present (Clark 2013; Shi and Sun 1999). Error, expectation and prediction are thought to be three key concepts of this approach.1 1 In this first sketch of the theory, I treat expectations and predictions as separate elements. Depending on the specific example, however, there may be no difference between the two (see, predictive coding in lateral and temporal inibition below). In Sect. 3, following the insight of an anonymous referee, I put this distinction into question. 123 Synthese (2018) 195:2367–2386 2369 When an image is transmitted, an expectation is created. The expectation is that the subsequent image will be roughly similar to the previous image. This expectation may in turn create a prediction of what the following pixel values in the incoming signal should be. The prediction is then either confirmed or disconfirmed by the incoming signal, which serves as an error sign. Starting in the 1980s, the predictive coding idea was applied to understanding the response behavior of neurons in preliminary stages of sensory processing—for example, in retinas and LGNs of flies, cats and monkeys (Barlow 1981; Dan et al. 1996; Srinivasan et al. 1982). Neurons in early sensory areas display both spatial lateral inhibition, and temporal inhibition. Inhibition means that neurons can exhibit behavior that is hampering, rather than excitatory. They can inhibit neighboring neurons, and they can self-inhibit. An example of spatial lateral inhibition is “center-surround” antagonism, where neurons in a given retinal layer are organized in such a way that the center is excitatory while the surround is inhibitory. Because of this arrangement, the behavior of excitatory neurons is not simply a function of the amount of light that hits them, but also a function of the inhibitory activity of nearby neurons. Similarly, in temporal inhibition (or self-inhibition) neurons have a “phasic” response profile. They may have an initial excitatory response, followed by a neutral or inhibitory response. A constant intensity of light may evoke virtually no sustained response other than the response due to the initial exposure. A flash of light, by con- trast, may evoke an initial excitatory response, followed by an inhibitory response (Srinivasan et al. 1982, p. 438). Several theories have been put forward to explain lateral and temporal inhibition. One hypothesis is that inhibition removes redundancy by reducing the response range of neurons, thus also enhancing their efficiency in transmitting information (Barlow 1961). Predictive coding is a framework used to spell out this idea. Lateral inhibition can be seen as the realization of a predictive code. In center-surround antagonism, surrounding neurons that have been exposed to nat- ural images generate a statistical estimate of the intensity expected at a particular point in the middle. By subtracting this best estimate (through inhibition) from the signal actually entering at the center, the center neurons communicate if there is something unexpected. In this way, redundant information is suppressed and neurons instantiate an efficient code. In temporal inhibition, a constant intensity light generates an initial response, but then virtually no response. Neurons “predict” the intensity coming in based on how they have already been excited. They subsequently stop responding unless there is a change in intensity—an error. Predictive coding is then introduced as a theory of information transmission that makes sense of the response behavior of some sensory neurons in vertebrates. Why make it into a more general idea about brains and cognitive activity? This is not always made explicit, but a number of factors likely contributed. Initially, the theory was used to understand the feedback connections between lay- ered neurons (Rao and Ballard 1999). As described so far, lateral inhibition involves the horizontal interplay of nearby neuronal elements. Inhibitory interactions, how- ever, can also occur “top-down”. The visual cortex is organized in layers of neurons 123 2370 Synthese (2018) 195:2367–2386 that often have reciprocal connections. Neurons at higher levels (for instance in V2) may send predictions down to neurons in lower levels (V1). The feedback connections serve as (inhibitory) predictions. The feedforward connections from V1 to V2 serve as error signals. Since not just the visual cortex, but the cerebral cortex in general is hierarchically structured, we can see how this theory can serve as a story of how the whole brain processes information (Rao and Ballard 1999, p. 85). Later, and perhaps more importantly, predictive coding insights were used to under- stand the effective control of action (Clark 2016, ch. 4). One example is given by skilled activity. A major puzzle in understanding how skilled activity takes place is given by information transmission problems. Information transmission problems arise because of the limitations of nerves and synapses. Such limitations produce signaling delays that would seem to impede fluid motion. In skilled (fast) reaching, for example, the brain has to receive, and respond to, a stream of proprioceptive information concerning the position and trajectory of one’s arm and hand, as well as information about the location of objects (Clark and Toribio 1994 p.
Details
-
File Typepdf
-
Upload Time-
-
Content LanguagesEnglish
-
Upload UserAnonymous/Not logged-in
-
File Pages20 Page
-
File Size-