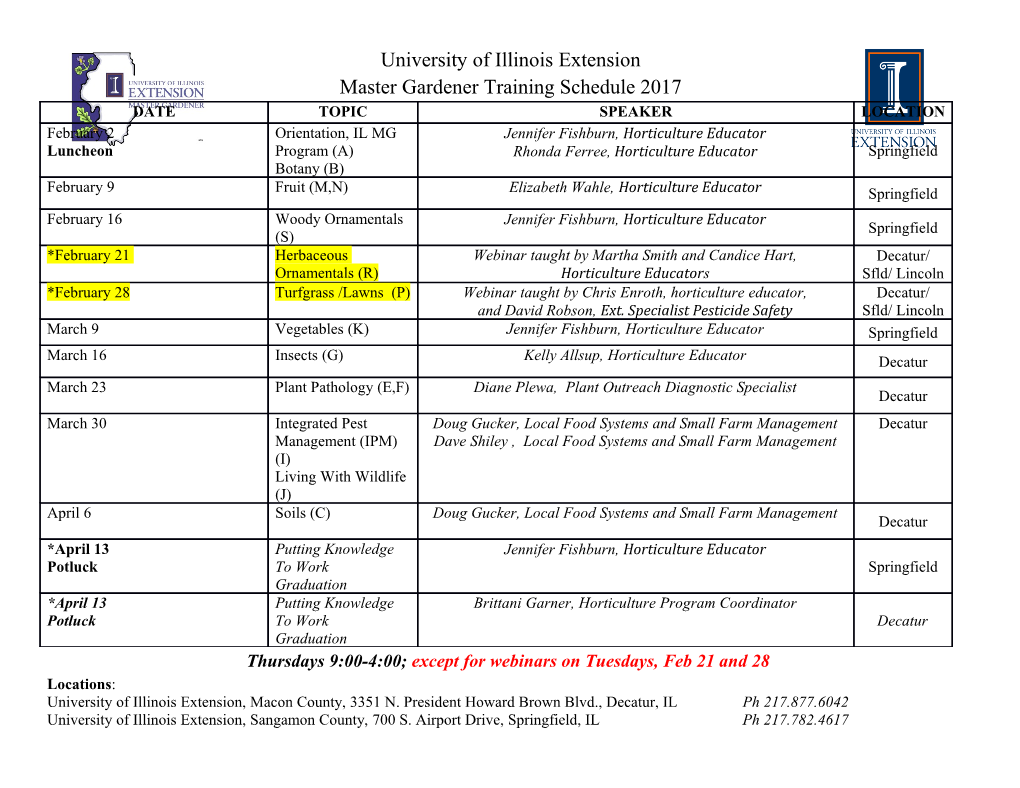
Scalable and Declarative Information Extraction in a Parallel Data Analytics System DISSERTATION zur Erlangung des akademischen Grades Doktor-Ingenieur (Dr.-Ing.) im Fach Informatik eingereicht an der Mathematisch-Naturwissenschaftlichen Fakultät Humboldt-Universität zu Berlin von Dipl.-Inf. Astrid Rheinländer Präsidentin der Humboldt-Universität zu Berlin: Prof. Dr.-Ing. habil. Dr. Sabine Kunst Dekan der Mathematisch-Naturwissenschaftlichen Fakultät: Prof. Dr. Elmar Kulke Gutachter: 1. Prof. Dr.-Ing. Ulf Leser 2. Prof. Dr. Felix Naumann 3. Prof. Dr.-Ing. Norbert Ritter eingereicht am: 24.01.2017 Tag der mündlichen Prüfung: 23.06.2017 Zusammenfassung Die Menge unstrukturierter Daten ist in den letzten Jahren enorm gewachsen und in diesem Zusammenhang hat sich auch die Analysekomplexität solcher Daten wesentlich erhöht. Informationsextraktion (IE) ist ein bedeutendes Verfahren für viele Anwendun- gen, in denen unstrukturierte Texte in strukturierte Daten transformiert werden, jedoch erfordert die systematische Anwendung von IE-Techniken auf sehr große Datenmengen hochkomplexe, skalierbare und anpassungsfähige Systeme. Obwohl bereits eine umfan- greiche Sammlung von IE-Werkzeugen und Algorithmen für verschiedene IE-Aufgaben existiert, ist die nahtlose und erweiterbare Kombination dieser Werkzeuge in einem skalierbaren end-to-end IE-System immer noch eine große Herausforderung. Diese Dissertation untersucht genau diese Problemstellung, d.h., es wird ein anfrage- basiertes IE-System innerhalb einer parallelen Datenanalyseplattform erforscht und en- twickelt, das für konkrete Anwendungsdomänen konfigurierbar ist und für Textsamm- lungen im Terabyte-Bereich skaliert. Innerhalb dieses Forschungsfeldes werden vier konsekutive Forschungsfragen bearbeitet. Zuerst werden konfigurierbare, algebra- ische Operatoren für alle grundlegenden IE-Aufgaben und für Web Text Analytics (WA) definiert. Es wird gezeigt wie diese Operatoren genutzt werden können um kom- plexe IE-Aufgaben in Form von Queries innerhalb der deklarativen Anfragesprache Meteor auszudrücken. Solche Queries werden in algebraische Data Flows übersetzt, analysiert, logisch und physikalisch optimiert und schließlich in parallele Data Flow- Programme übersetzt, die mit der parallelen Datenanalyseplattform Stratosphere aus- geführt werden. Alle Operatoren werden hinsichtlich ihrer physikalischen, algebrais- chen und Laufzeiteigenschaften charakterisiert um sowohl das Potenzial als auch die Bedeutung der Optimierung der Ausführungsreihenfolge nicht-relationaler, benutzer- definierter Operatoren für Data Flows (UDFs) hervorzuheben. Als zweite Forschungs- frage wird der Stand der Technik in der Optimierung nicht-relationaler Data Flows untersucht. Relevante Optimierungstechniken, die in verschiedenen Phasen des Op- timierungsprozesses in parallelen Datenanalysesystemen eingesetzt werden, werden vorgestellt und existierende Data Flow-Anfragesprachen werden umfassend hinsichtlich der verfügbaren Optimierungstechniken analysiert. Die Analyse kommt zu dem Schluss, dass eine umfassende Optimierung von UDFs für viele Systeme immer noch eine Her- ausforderung ist. Basierend auf dieser Beobachtung schließt sich die dritte Forschungs- frage an, in der ein erweiterbarer, logischer Optimierer erforscht und entwickelt wird, der die Semantik von UDFs mit in den Optimierungsprozess mit einbezieht (SOFA). SOFA analysiert eine kompakte Menge von Eigenschaften, die die Semantik der UDFs beschreiben und kombiniert die automatisierte Analyse mit manuellen UDF-Annotatio- nen, um eine umfassende Optimierung von Data Flows zu ermöglichen. SOFA ist in der Lage, beliebige Data Flows aus unterschiedlichen Anwendungsbereichen logisch zu op- timieren, was zu erheblichen Laufzeitverbesserungen im Vergleich mit anderen Tech- niken führt. Als Viertes wird die Anwendbarkeit des vorgestellten IE-Systems auf real- weltliche Textsammlungen im Terabyte-Bereich untersucht, in dem Inhalte des World Wide Webs zu gesundheitsrelevanten Themen mit wissenschaftlichen Veröffentlichun- gen verglichen werden. Im Rahmen dieser Studie wird systematisch die Skalierbarkeit und Robustheit der eingesetzten Methoden und Werkzeuge untersucht sowie die Qual- ität der extrahierten Daten analysiert um schließlich die kritischsten Herausforderun- gen beim Aufbau eines IE-Systems für sehr große Datenmenge zu charakterisieren. iii Abstract In recent years, the size of unstructured data has grown tremendously and the com- plexity of the analysis of such data has increased significantly. In many domains, infor- mation extraction (IE) is an important technique to turn unstructured texts into struc- tured fact databases, but systematically applying IE techniques to very large inputs requires highly complex, adaptable, and scalable systems. Although a number of tools for different IE tasks exist, their seamless, extensible, and scalable combination into a large-scale end-to-end text analytics system still is a true challenge. This thesis addresses exactly this problem, i.e., we research and develop a query- based IE system that is accurate, configurable towards concrete application domains, and scalable to Terabyte-scale text collections inside a parallel data analytics system. Within this topic, we conduct four consecutive research tasks: First, we introduce a set of domain-independent, algebraic operators, which address all fundamental tasks in IE and web text analytics (WA) and which can be used to express complex IE tasks in form of queries inside the declarative data flow language Meteor. Such queries are parsed into algebraic data flows, which are logically and physically optimized, translated into parallel data flow programs, and executed with the parallel processing system Strato- sphere. We characterize all operators with physical, algebraic, and runtime properties to highlight both the potential and importance of optimizing the execution order non- relational, user-defined data flow operators (UDFs). Second, we survey the state-of-the- art in optimization techniques for data flows with UDFs, which are applied at different stages of the optimization process in parallel data analytics systems. We provide a com- prehensive overview on declarative data flow languages for parallel data analytics sys- tems from the perspective of their build-in optimization techniques and conclude that comprehensive optimization of UDFs and non-relational operators still is a true chal- lenge for many systems. Third, we introduce a semantics-aware and extensible logical optimizer for data flows with UDFs based on this observation. Our optimizer builds on a concise set of properties for describing the UDF’s semantics and combines automated analysis of UDFs with manual annotations to enable comprehensive data flow optimiza- tion. We show that our approach is capable of reordering data flows of arbitrary shape from different application domains, leading to considerable runtime improvements and clearly outperforming plans found by other techniques. Fourth, we study the real-life applicability of our system to Terabyte-scale text collections in a challenging setting to compare the "web view" on health-related topics with that derived from a controlled scientific corpus. We systematically evaluate scalability, quality, and robustness of the employed methods and tools and also pinpoint the most critical challenges in building such a system. iv Acknowledgments After a period of almost six years, today is the day: writing this note of thanks is the finishing touch on my dissertation. It has been a period of intense learning for me, not only scientifically, but also on a personal level and I would like to thank the people who have supported me most during this thime. First and foremost, I would like to express my sincere gratitude to my advisor Ulf Leser for his continuous support and encouragement during my academic life over the past 10 years. Ever since I was an undergraduate student, he gave me the opportunity to work on amazing projects, which raised my interest in computer science research in general and in large-scale data management in particular. His guidance, patience, and immense knowledge helped me in all this time and especially during researching and writing this thesis. Thank you, Ulf! I greatly appreaciate the inspiring environment provided by all members of the DFG research group Stratosphere – Information Management on the Cloud. Many thanks to all principal investigators and my fellow graduate students, who listened and engaged in many fruitful discussions during our project meetings. Especially Arvid Heise, Fabian Hueske, and Felix Naumann helped a lot to sharpen and improve results presented here by asking the right questions and by challenging me constantly. I am grateful for the dedication of my student assistants Anja Kunkel, Martin Beckmann, and Jörg Meier, who worked tirelessly to ensure that our system kept up to speed with the development of Stratosphere’s core components. I would also like to thank my fellow graduate students and co-workers at WBI, who made sure that I always enjoyed driving to Adlershof in the morning. I thank Stefan Kröger for being the best office mate I could imagine having for more than five years. Especially spending many coffee breaks with Karin Zimmermann, Philippe Thomas, Marc Bux, and Birgit Heene and chatting about life-related topics provided just the right amount of distraction whenever I needed it. Most importantly, I would like to thank my family for their continuous support and love. Especially Christian, who encouraged
Details
-
File Typepdf
-
Upload Time-
-
Content LanguagesEnglish
-
Upload UserAnonymous/Not logged-in
-
File Pages195 Page
-
File Size-