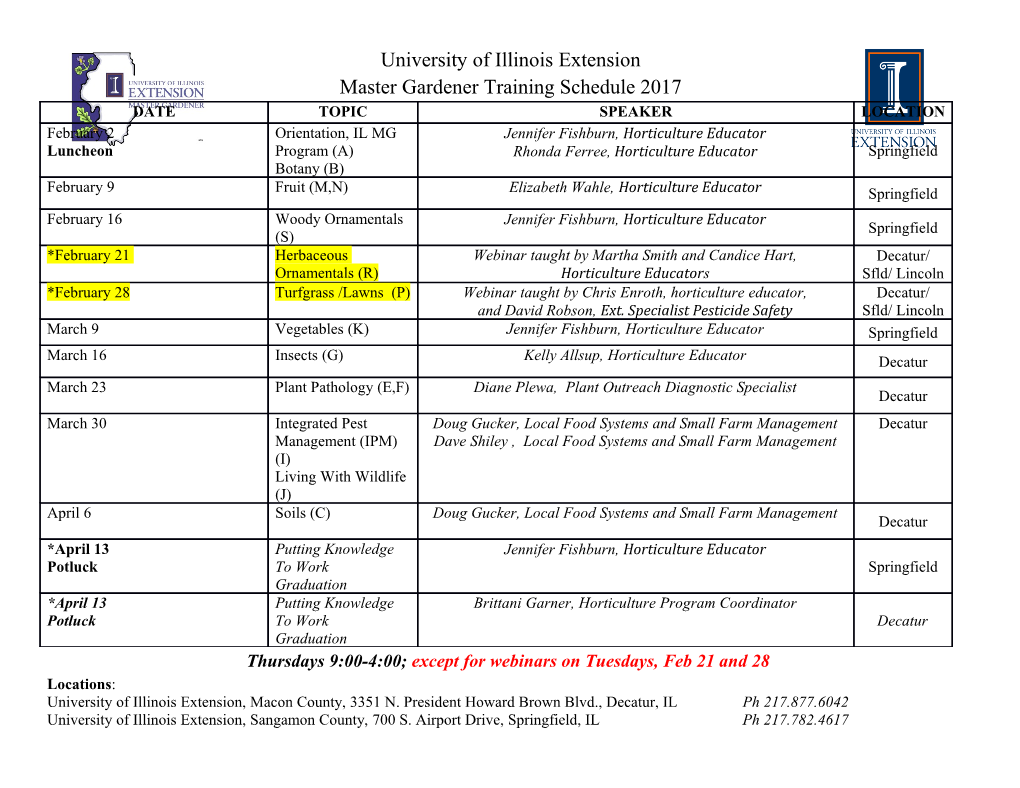
remote sensing Article A Spatio-Temporal Analysis of Rainfall and Drought Monitoring in the Tharparkar Region of Pakistan Muhammad Usman 1 and Janet E. Nichol 2,* 1 Centre for Geographical Information System, University of the Punjab, Lahore 54590, Pakistan; [email protected] 2 Department of Geography, School of Global Studies, University of Sussex, Brighton BN19RH, UK * Correspondence: [email protected]; Tel.: +852-9363-8044 Received: 6 January 2020; Accepted: 5 February 2020; Published: 10 February 2020 Abstract: The Tharpakar desert region of Pakistan supports a population approaching two million, dependent on rain-fed agriculture as the main livelihood. The almost doubling of population in the last two decades, coupled with low and variable rainfall, makes this one of the world’s most food-insecure regions. This paper examines satellite-based rainfall estimates and biomass data as a means to supplement sparsely distributed rainfall stations and to provide timely estimates of seasonal growth indicators in farmlands. Satellite dekadal and monthly rainfall estimates gave good correlations with ground station data, ranging from R = 0.75 to R = 0.97 over a 19-year period, with tendency for overestimation from the Tropical Rainfall Monitoring Mission (TRMM) and underestimation from Climate Hazards Group Infrared Precipitation with Stations (CHIRPS) datasets. CHIRPS was selected for further modeling, as overestimation from TRMM implies the risk of under-predicting drought. The use of satellite rainfall products from CHIRPS was also essential for derivation of spatial estimates of phenological variables and rainfall criteria for comparison with normalized difference vegetation index (NDVI)-based biomass productivity. This is because, in this arid region where drought is common and rainfall unpredictable, determination of phenological thresholds based on vegetation indices proved unreliable. Mapped rainfall distributions across Tharparkar were found to differ substantially from those of maximum biomass (NDVImax), often showing low NDVImax in zones of higher annual rainfall, and vice versa. This mismatch occurs in both wet and dry years. Maps of rainfall intensity suggest that low yields often occur in areas with intense rain causing damage to ripening crops, and that total rainfall in a season is less important than sustained water supply. Correlations between rainfall variables and NDVImax indicate the difficulty of predicting drought early in the growing season in this region of extreme climatic variability. Mapped rainfall and biomass distributions can be used to recommend settlement in areas of more consistent rainfall. Keywords: biomass; CHIRPS; NDVI; satellite rainfall; Tharparkar 1. Introduction The Thar Desert, located to the northwest of the Indian subcontinent, is one of the largest subtropical deserts [1]. It is regarded as the only fertile desert in the world, with a population dependent on rain-fed agriculture and livestock rearing, and it was declared by the World Food Program as the most food-insecure region of Pakistan [2]. Pakistan itself is the fifth most affected country in the long-term Climate Risk Index [3]. The climate of the Thar is characterized by low and erratic rainfall, high temperature, and long spells of dry weather, which threaten agricultural livelihoods. Tharparkar is the largest district in Sindh province, but climatic stations in Sindh are sparsely distributed between 100 and 150 km apart. Mithi, the only continuous rain gauge station within Tharparkar (Figure1), has 277 mm of rainfall annually, but this varies greatly from year Remote Sens. 2020, 12, 580; doi:10.3390/rs12030580 www.mdpi.com/journal/remotesensing Remote Sens. 2020, 12, 580 2 of 16 Remote Sens. 2020, 12, x FOR PEER REVIEW 2 of 16 toyear. year. Pressure Pressure on on water water resources resources is iscompounded compounded by by population population growth, growth, as as the populationpopulation ofof TharparkarTharparkar increased from from 0.4 0.4 million million in in the the 1960s 1960s to to0.9 0.9 million million in 1998 in 1998 [4], [and4], and1.649 1.649 million million in 2017 in 2017[5], almost [5], almost doubling doubling over overthe last the two last decades two decades (Table (Table 1). 1). FigureFigure 1. 1.Tharparkar Tharparkar regionregion andand itsits locationlocation inin Pakistan,Pakistan, showing location of continuous continuous rain rain gauges. gauges. Table 1. Table 1. HumanHuman populationpopulation growthgrowth inin TharparkarTharparkar districtdistrict [[5].5]. 1960s1960s 1970s1970s 1980s 1998 1998 2017 2017 400,000400,000 622,233622,233 774,617 907,000 907,000 1,649,000 1,649,000 0.40.4 m m 0.620.62 m m 0.77 mm 0.91 0.91 m 1.64 1.64 m m TheThe increaseincrease inin frequencyfrequency andand severityseverity of droughts in recent decades reduced agricultural yield, leadingleading toto increasedincreased povertypoverty andand foodfood insecurity.insecurity. InIn fact,fact, inin FebruaryFebruary 2014,2014, droughtdrought duedue toto extremelyextremely lowlow rainfallrainfall inin TharparkarTharparkar resultedresulted inin 167167 humanhuman fatalitiesfatalities andand greatgreat lossloss ofof animalanimal lifelife [[2].2]. AA recentrecent studystudy [[1]1] challengedchallenged prevailingprevailing notionsnotions about aridityaridity and changing rainfall pattern in Tharparkar. TheyThey foundfound thatthat lowerlower agriculturalagricultural yield,yield, leadingleading toto foodfood scarcityscarcity inin thethe last four decades, was actually accompaniedaccompanied byby increasedincreased annualannual rainfallrainfall atat thethe raterate ofof 6.356.35 mmmm/year./year. Thus,Thus, theythey attributeattribute recentrecent hardshipshardships ofof foodfood insecurityinsecurity andand waterwater scarcityscarcity inin TharparkarTharparkar toto erraticerratic rainfallrainfall ratherrather thanthan overalloverall rainfallrainfall deficit.deficit. With anan averageaverage winterwinter temperaturetemperature ofof approximatelyapproximately 2020 ◦°C,C, temperaturetemperature isis nevernever aa limitinglimiting factorfactor toto plantplant productivity.productivity. TheThe scenarioscenario ofof increasing hungerhunger andand starvationstarvation inin recent yearsyears demandsdemands aa better response fromfrom authoritiesauthorities inin thethe future,future, forfor whichwhich identifyingidentifying thethe spatialspatial andand temporal dimensions ofof drought inin any seasonseason isis required.required. AA number number of of studies studies used used satellite satellite datasets datasets to to identify identify and and characterize characterize drought drought by comparingby comparing rainfall rainfall products products [6, 7[6,7]] with with vegetation vegetation indices indices over over time time [8 [8–10].–10]. However, However, the the abilityability toto detectdetect patternspatterns ofof pastpast droughtdrought usingusing time-seriestime-series satellitesatellite datadata doesdoes notnot automaticallyautomatically translatetranslate intointo droughtdrought predictionprediction ability.ability. Indeed,Indeed, AzizAziz etet al.al. [[11]11] working in Pakistan demonstrated satellites’ ability toto detectdetect droughtdrought overover largelarge areasareas inin PakistanPakistan usingusing vegetationvegetation indices,indices, butbut diddid notnot show how drought oror itsits impactsimpacts couldcould bebe predictedpredicted inin advance.advance. MoreMore recentrecent developmentsdevelopments usingusing satellitesatellite datadata forfor phenologicalphenological analysisanalysis showshow moremore promisepromise ifif spatialspatial relationshipsrelationships betweenbetween totaltotal cropcrop yields and seasonal phenological variables can be established. TheseThese variablesvariables include include the the start, start, length, length, and and end end of the of growingthe growing season, season, drought drought periods, periods, and the and nature the ofnature rainfall, of whetherrainfall, early,whether late, early, frequent, late, intense, frequent, or well-distributed.intense, or well-distributed. Such variables Such can potentiallyvariables can be potentially be mapped using satellite images and their trends identified [12–14]. However, different methodologies have been shown to give significantly different seasonal transition dates [14] and Remote Sens. 2020, 12, 580 3 of 16 mapped using satellite images and their trends identified [12–14]. However, different methodologies have been shown to give significantly different seasonal transition dates [14] and algorithms effective in one study area may not apply in others. White et al. [15] compared 10 different techniques for defining the start of the growing season (SOS), finding average differences of 60 days and standard ± deviation of 20 days among the different methods. Since temperature is the most common limiting ± factor to growth, many studies used temperature thresholds to define growing season days such as daily average above 5 ◦C[16]. Landscapes in more arid regions and/or those with low seasonal amplitude of vegetation indices were shown by several studies to give the lowest agreement between satellite normalized difference vegetation index (NDVI) and ground-measured phenology [12–14]. Kang et al. [12] also demonstrated uncertainty in phenology measurement arising from the spatial resolutions of different satellite sensors. Thus, satellite-based precipitation estimates may be able to provide a viable alternative to sparsely distributed
Details
-
File Typepdf
-
Upload Time-
-
Content LanguagesEnglish
-
Upload UserAnonymous/Not logged-in
-
File Pages16 Page
-
File Size-