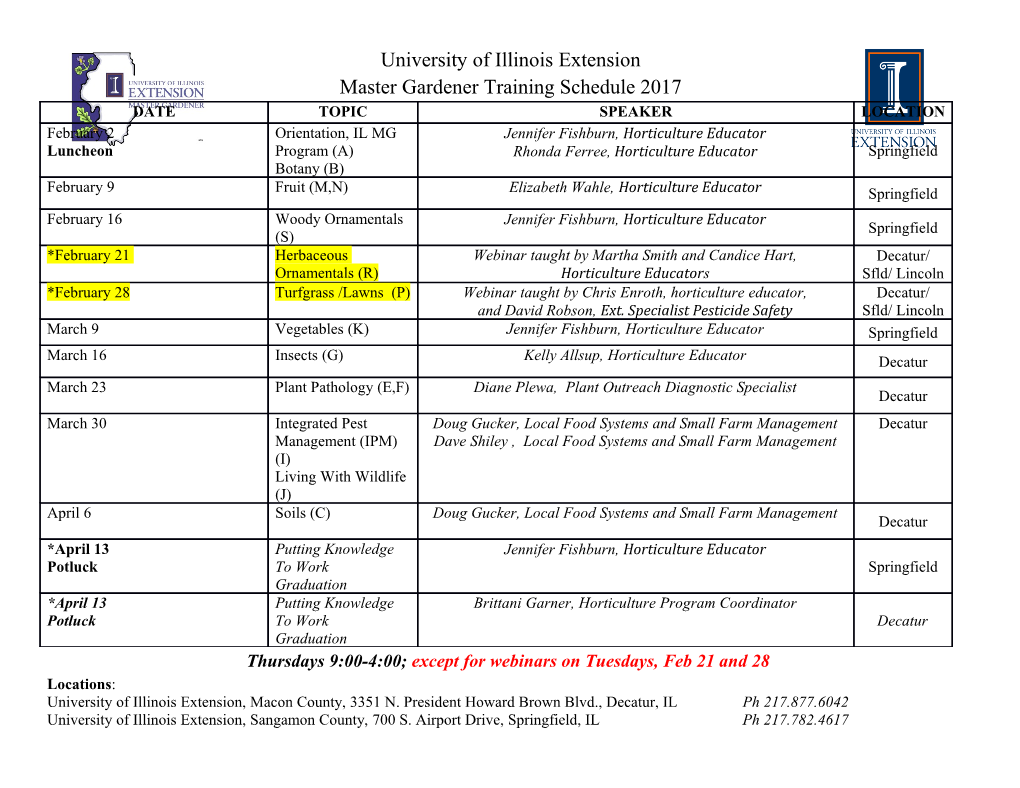
Automatic interlinear glossing as two-level sequence classification Tanja Samardžic´ Robert Schikowski Sabine Stoll Corpus Lab Department of Department of URPP Language and Space Comparative Linguistics Comparative Lingistics University of Zurich University of Zurich University of Zurich tanja.samardzic | robert.schikowski | [email protected] Abstract (1) Kofi tso ati- fo Yao (yi). Interlinear glossing is a type of annotation Kofi take stick-DEF hit Yao P of morphosyntactic categories and cross- Kofi took the stick and hit Yao with it. linguistic lexical correspondences that al- With the development of annotated linguistic lows linguists to analyse sentences in lan- corpora of various languages, glosses are starting guages that they do not necessarily speak. to be used in a new way. Traditionally, only in- Automatising this annotation is necessary dividual sentences or small text collections were in order to provide glossed corpora big glossed to illustrate examples. Nowadays glosses enough to be used for quantitative studies. are systematically added to large corpora in or- In this paper, we present experiments on der to provide structural information necessary for the automatic glossing of Chintang. We quantitative cross-linguistic research. decompose the task of glossing into steps Despite their great value for linguistic research, suitable for statistical processing. We first glossed corpora often remain rather small. The perform grammatical glossing as standard main reason for this is the fact that glossing re- supervised part-of-speech tagging. We quires a high level of linguistic expertise and is then add lexical glosses from a stand-off currently performed manually by trained experts. dictionary applying context disambigua- This practice makes the creation of glossed cor- tion in a similar way to word lemmatisa- pora extremely time-consuming and expensive. In tion. We obtain the highest accuracy score order to obtain glossed corpora large enough for of 96% for grammatical and 94% for lexi- reliable quantitative analysis, the process of gloss- cal glossing. ing needs to be automatised. 1 Introduction In this paper, we present a series of experi- ments performed with this precise aim.2 We di- The annotation type known as interlinear gloss- vide the traditional glossing procedure into several ing allows linguists to describe the morphosyn- steps and define an automatic processing pipeline, tactic makeup of words concisely and language- which consists of some standard and some cus- independently. While glosses as a linguistic met- tom natural language processing tasks. The data alanguage have a long tradition, systematic stan- we use for our experiments come from the Chin- dards for interlinear glossing have only developed tang Language Corpus (Bickel et al., 2004 2015), relatively recently – cf. e.g. the Leipzig glossing an exceptionally large glossed corpus, which has rules.1 been developed since 2004 and is presently hosted An example for an interlinear gloss is shown in at the Department of Comparative Linguistics at (1), which is an Ewe serial verb construction taken the University of Zurich.3 from (Collins, 1997) with glosses in boldface. The combination of segmentation with English meta- 2 Related work language labels for both lexical and grammatical segments allows linguists to observe how exactly Data for comprehensive linguistic research need to the Ewe serial verb construction differs from the be collected in a wide range of languages. Glosses corresponding English construction. 2This work is partially supported by the S3IT computing 1 facilities. Available at https://www.eva.mpg.de/ 3 lingua/resources/glossing-rules.php http://www.clrp.uzh.ch 68 Proceedings of the 9th SIGHUM Workshop on Language Technology for Cultural Heritage, Social Sciences, and Humanities, pages 68–72, Beijing, China, July 30, 2015. c 2015 Association for Computational Linguistics and The Asian Federation of Natural Language Processing are especially important for research in under- record ID rabbit.047 resourced languages, the analysis of which re- transcription mande aba katha quires more detailed information than it is the case segmentation mand -e abo katha with well documented and processed languages. glosses be.over -ind.pst now story Approaches to under-resourced languages in- language C -C C/N N clude developing tools to support manual rule lexicon ID 281 -1234 596 4505 crafting and deep rule-based analysis (Bender et PoS vi -gm adv n al., 2014; Snoek et al., 2014), data collection by English Now the story is over. experts (Ulinski et al., 2014; Hanke and Bird, Table 1: Example for record structure in the Chin- 2013) and through crowd-sourcing (Bird et al., tang Language Corpus 2014; Dunham et al., 2014), automatic transla- tion and cross-linguistic projection using parallel lexeme mand corpora (Yarowsky et al., 2001; Scherrer, 2014; ID 281 Scherrer and Sagot, 2014; Aepli et al., 2014), and variant mai part-of-speech tagging (Garrette and Baldridge, PoS vi 2013). valency S-NOM(1) V-s(S) These tasks target different representations, but English gloss be.finished; be.over; be.used.up the resources that they produce are not suitable for language C corpus-based quantitative linguistic research. Our approach to automatic linguistic glossing is in- Table 2: Example for entry structure in the Chin- tended to fill this gap. Like Garrette and Baldridge tang dictionary (2013), we learn our target representation from a relatively small sample of manually developed re- sources in the target language. However, we tackle a harder task and rely on more resources, which are becoming increasingly available through work to the fifth element on the gloss tier). on language documentation. Table 1 shows a simplified example of an anal- ysed record. Beside segmentation and interlinear 3 The structure of the Chintang corpus glosses, the analysis also includes POS tags, lan- and glossing strategy guage labels, and lexical IDs for every morpheme. The language labels are needed because mixing The corpus consists of about 290 hours (1,232,161 with other languages (Nepali and Bantawa) is fre- words) of video materials transcribed in broad quent in Chintang (Stoll et al., 2015). IPA. 214 hours (955,025 words) have been trans- The lexical IDs provide a unique link to the en- lated to English and Nepali by native research as- tries of an electronic lexicon of Chintang, which sistants and glossed by trained non-native student contains rich information both on free and on de- assistants. The primary data are MPG-1 videos pendent morphemes. A simplified example of a and WAV audio files. Morphological Transcrip- lexicon entry is given in Table 2. tions and translations were done in Transcriber Lexical IDs ensure good communication be- and ELAN, glossing in Toolbox. tween the corpus and the lexicon, allowing for The basic unit of a Toolbox text is the record, queries involving both resources at the same time which in oral corpora usually corresponds to one (e.g. combining valency or etymology information utterance. Records are separated from each other from the lexicon with corpus counts) as well as by double newlines. Each record may contain sev- systematic updates and synchronisation of both re- eral tiers, each of which is coded as a line ended by sources. a single newline. Within each tier, both words and morphemes are separated by whitespace. Free and 4 Automatic glossing pipeline bound morphemes are distinguished by adding a As shown in the previous section, linguistic an- corresponding separator (prefixes end and suffixes notation of the Chintang corpus consists of word begin with a “-”). Elements are implicitly aligned segmentation and proper glossing. across tiers based on their position on a tier (e.g. Word segmentation can be seen as a pre- the fifth element on the segment tier corresponds processing step that creates basic units of analysis 69 to which glosses are assigned. Once the words are trated in (2), where the lexical glosses be.over, segmented into morphemes that encode either lex- now, and story are replaced by their correspond- ical content or grammatical categories, assigning ing part-of-speech tags vi, adv, and n. glosses to the word segments reduces to a one-to- one mapping: each segment in a sentence is as- (2) mand -e abo katha signed exactly one gloss and vice versa. vi -ind.pst adv n We take manual segmentation as input and learn automatically the mappings between segments and In this way, we obtain a corpus annotated with glosses. This mapping includes referring to the 233 distinct labels that describe relevant mor- corresponding lexicon and importing the informa- phosyntactic categories in Chintang. tion from lexical entries. We then split the corpus into a train and a A simple way to learn this mapping would be test set and apply a standard supervised part-of- to treat glossing as word-by-word translation from speech tagging. We train and test a general- original text to an artificial language that consists purpose state-of-the-art statistical tagger (Ges- of words and grammatical tags. Word order in the mundo, 2011; Gesmundo and Samardžic,´ 2012). artificial language would be exactly the same as in To assess how the quantity of training data influ- the original text, so that the task would reduce to ences the performance of the tagger, we run the ex- learning segment translation probabilities. periment several times using increasing amounts The main disadvantage of the translation-based of data for training. The results of the tagging ex- approach is that it requires large corpora for train- periments are presented in Figure 1. ing. This approach does not generalise beyond examples seen in the training set, which is why 4.2 Lexical annotation as lemmatisation good coverage of a new text can be obtained only To recover the original glosses, we replace part- if the model is trained on a large corpus.
Details
-
File Typepdf
-
Upload Time-
-
Content LanguagesEnglish
-
Upload UserAnonymous/Not logged-in
-
File Pages5 Page
-
File Size-