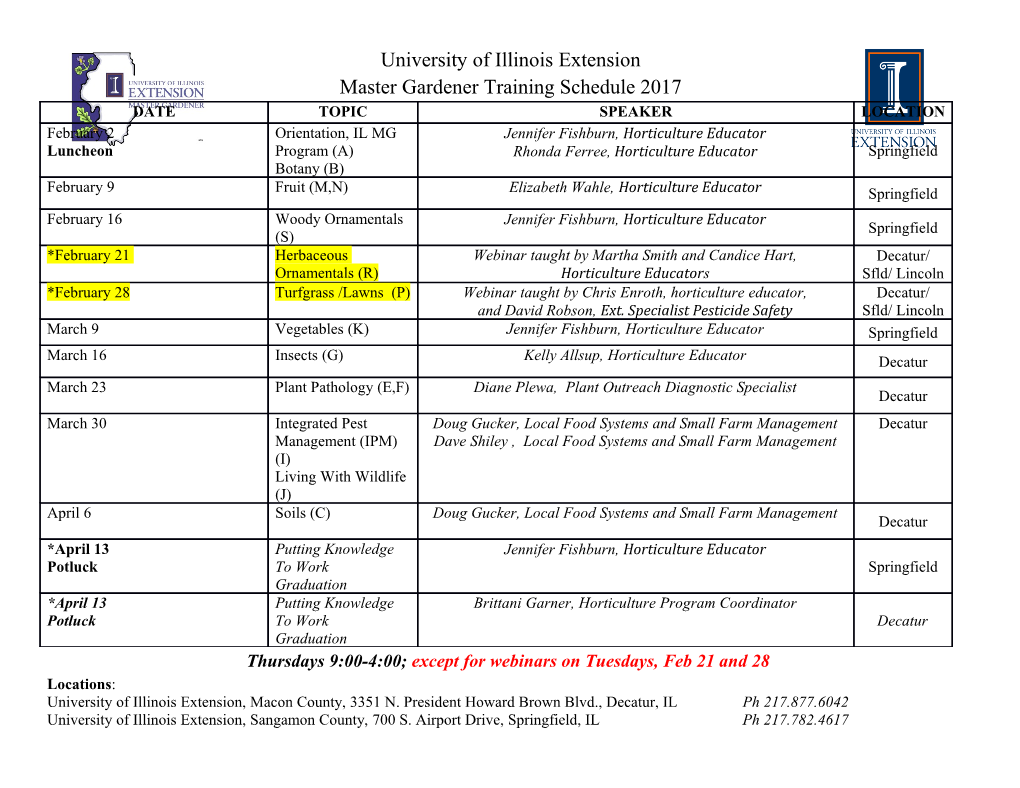
Learn to Test for Heteroscedasticity in SPSS With Data From the China Health and Nutrition Survey (2006) © 2015 SAGE Publications, Ltd. All Rights Reserved. This PDF has been generated from SAGE Research Methods Datasets. SAGE SAGE Research Methods Datasets Part 2015 SAGE Publications, Ltd. All Rights Reserved. 1 Learn to Test for Heteroscedasticity in SPSS With Data From the China Health and Nutrition Survey (2006) Student Guide Introduction This dataset example introduces readers to testing for heteroscedasticity following a linear regression analysis. Linear regression rests on several assumptions, one of which is that the variance of the residual from the model is constant and unrelated to the independent variable(s). Constant variance is called homoscedasticity, while non-constant variance is called heteroscedasticity. This example describes heteroscedasticity, discusses its consequences, and shows how to detect it using data from the 2006 China Health and Nutrition Survey (CHNS) survey of adults (http://www.cpc.unc.edu/projects/china). Specifically, we test whether systolic blood pressure is a linear function of a person’s age. After performing the regression, we show how to examine the results for evidence of heteroscedasticity. High blood pressure is associated with a number of negative health outcomes. Results from an analysis like this could therefore have implications for individual behavior and public health policy. What Is Heteroscedasticity? Linear regression analysis expresses a dependent variable as a linear function of one or more independent variables. Equation 1 shows an example of a simple Page 2 of 14 Learn to Test for Heteroscedasticity in SPSS With Data From the China Health and Nutrition Survey (2006) SAGE SAGE Research Methods Datasets Part 2015 SAGE Publications, Ltd. All Rights Reserved. 1 linear model with a single independent variable: (1) Yi = β0 + β1Xi + εi Where: • Yi = individual values of the dependent variable • Xi = individual values of the independent variable • β0 = the intercept, or constant, associated with the regression line • β1 = the slope of the regression line • εi = the unmodeled random, or stochastic, component of the dependent variable, often called the error term or the residual of the model. Linear regression models are typically estimated using ordinary least squares (OLS) regression. OLS regression rests on several assumptions, one of which is that the variance of the residuals from the regression model (εi) is constant and unrelated to the independent variable(s). Heteroscedasticity means that the variance of the residual is not constant, which means that an important assumption of OLS has been violated. Consequences of Heteroscedasticity The presence of heteroscedasticity does not affect the estimated values of the intercept or slope coefficients of a linear regression model. Those estimates remain unbiased. However, heteroscedasticity does affect the estimated standard errors for those coefficients. It can make them either too large or to small, but most often it makes them too small. Page 3 of 14 Learn to Test for Heteroscedasticity in SPSS With Data From the China Health and Nutrition Survey (2006) SAGE SAGE Research Methods Datasets Part 2015 SAGE Publications, Ltd. All Rights Reserved. 1 The standard error of any statistic is calculated to provide an estimate of how much that statistic might change if it were calculated again on another random sample of data of the same size taken from the same population. For regression, the standard error for each coefficient provides an estimate of uncertainty about that coefficient. We use both the coefficients and their standard errors when we test hypotheses about those coefficients. For example, we might estimate a regression model like the one presented in Equation 1 and produce an estimate of β1 of 2.5. In order to determine whether 2.5 is statistically significantly different from zero, we need to perform a hypothesis test. Specifically, we would test the null hypothesis that β1 = 0. To do so, we would: 1. Perform the regression analysis to produce our estimates of and its standard error. 2. Divide the estimate of by its standard error to produce a T-score. 3. Compare the T-score from the previous step to a Student’s T distribution, with degrees of freedom equal to the sample size minus the number of coefficients estimated as part of the original regression. 4. Determine the level of significance, or p-value, associated with the calculated T-score. Typically, if that p-value is less than 0.05, researchers would declare the result to be statistically significant. Of course, statistical software generally performs all of these steps for us automatically. However, this process and those computer programs assume that the variance of the residuals is constant. As noted above, heteroscedasticity leads to incorrect estimates of standard errors. As a result, heteroscedasticity will confound subsequent hypothesis testing. Because heteroscedasticity typically produces standard errors that are smaller than they should be, we run the risk of being over-confident in our coefficient estimates and possibly declaring a coefficient estimate to be statistically significant when it is not. Page 4 of 14 Learn to Test for Heteroscedasticity in SPSS With Data From the China Health and Nutrition Survey (2006) SAGE SAGE Research Methods Datasets Part 2015 SAGE Publications, Ltd. All Rights Reserved. 1 Detecting Heteroscedasticity There are two main strategies for detecting heteroscedasticity. The first approach is graphical. For a simple regression model, a two-way scatter plot with the residuals from the regression model plotted on the Y-axis and the independent variable plotted on the X-axis is a good place to start. For a multiple regression model, you could produce separate plots like this for each independent variable – with the residuals plotted on the Y-axis and the independent variable in question plotted on the X-axis. For either a simple or a multiple regression model, it is quite common plot the residuals on the Y-axis and the predicted value of the dependent variable based on the regression model on the X-axis. Often both the residuals and the predicted values of the dependent variable are standardized before being plotted. Figure 1 shows an example of this approach. Figure 1: Illustration comparing what homoscedasticity and heteroscedasticity look like using two-way scatter plots with the standardized residual from the regression plotted on the Y-axis and the standardized predicted value of the dependent variable from the regression plotted on the X-axis. Page 5 of 14 Learn to Test for Heteroscedasticity in SPSS With Data From the China Health and Nutrition Survey (2006) SAGE SAGE Research Methods Datasets Part 2015 SAGE Publications, Ltd. All Rights Reserved. 1 Regardless of which figures you produce, you should see the same level of vertical spread among the residuals across all values plotted along the X-axis as you look from left to right at the plot if you have constant variance in the residuals. However, if you have heteroscedasticity, you will see changes in the vertical spread among the residuals as you look across the figure from left to right. That spread could be steadying growing, steadily shrinking, or showing a more complex pattern such as less variance in the center of the data than at both the lower and upper extremes. The panel on the left in Figure 1 shows how the vertical spread of the standardized residuals is roughly the same as you look from left to right. However, the panel on the right in Figure 1 shows a smaller vertical spread for the residuals initially that spreads out wider as you move from left to right. The panel on the left shows what homoscedasticity looks like. The panel on the right shows what one of the more common forms of heteroscedasticity looks like. Graphical methods are useful for seeing the data, but they lack formal statistical Page 6 of 14 Learn to Test for Heteroscedasticity in SPSS With Data From the China Health and Nutrition Survey (2006) SAGE SAGE Research Methods Datasets Part 2015 SAGE Publications, Ltd. All Rights Reserved. 1 precision. As a result, many researchers turn to formal statistical tests for heteroscedasticity. There are many tests of the null hypothesis of homoscedasticity, but the most common is the Breusch–Pagen test (sometimes called the Breusch–Pagan–Godfrey test; also developed independently as the Cook–Weisberg test). The Breusch–Pagen test unfolds in several steps: 1. Estimate the regression model of interest and save the residuals, εi, for each observation. ^ 2 2. Compute a number we will label σ by: 2.1 squaring each residual, εi 2.2 summing up those squared residuals 2.3 dividing the result by the size of your sample from the regressions model you estimated. ε2 3. i Compute a new variable named ρi as equal to ^ 2 . σ 4. Run a new auxiliary regression with ρi as the dependent variable and all of the same independent variables that were part of your original regression. 5. Compute the explained sum of squares from this auxiliary regression and divide it by 2. 6. Compare the result from the previous step to a Chi-squared probability distribution with degrees of freedom equal to the number of independent variables included in the auxiliary regression. 7. If the level of significance, or p-value, associated with the result from the previous step is small – typically below 0.05 – you can reject the null hypothesis of homoscedasticity and declare that you do in fact have evidence of heteroscedasticity. Page 7 of 14 Learn to Test for Heteroscedasticity in SPSS With Data From the China Health and Nutrition Survey (2006) SAGE SAGE Research Methods Datasets Part 2015 SAGE Publications, Ltd. All Rights Reserved. 1 Fortunately, most (but not all) statistical software programs have this and other formal tests for heteroscedasticity built in.
Details
-
File Typepdf
-
Upload Time-
-
Content LanguagesEnglish
-
Upload UserAnonymous/Not logged-in
-
File Pages14 Page
-
File Size-