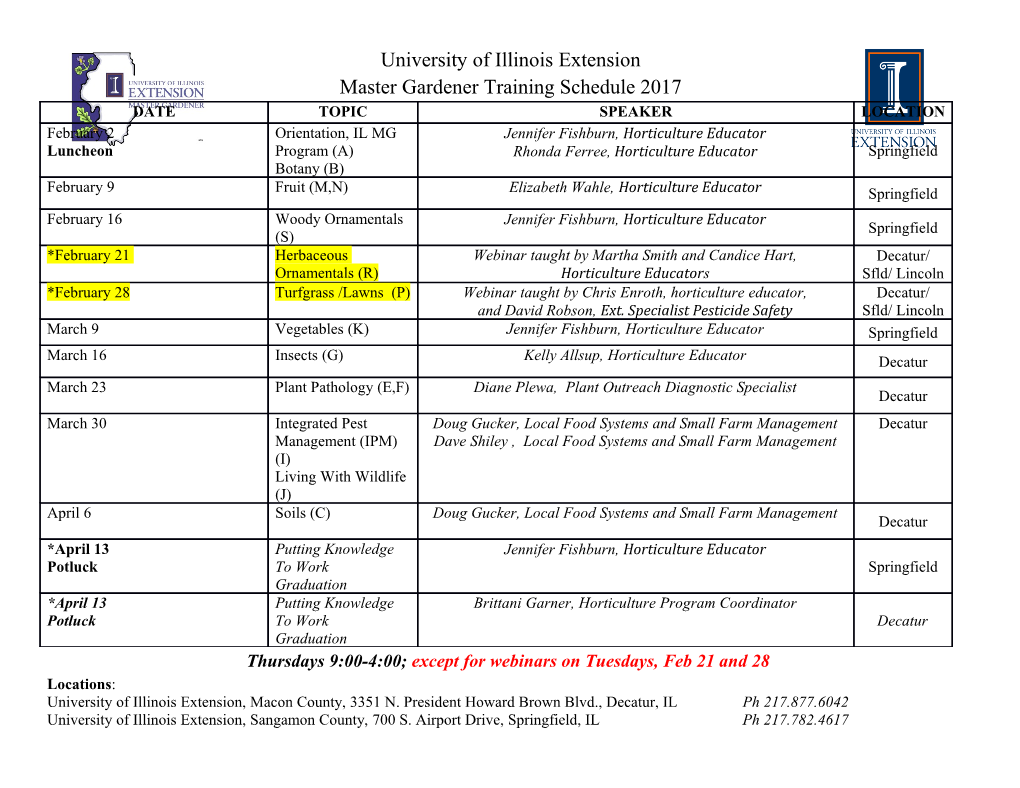
HPC and AI Middleware for Exascale Systems and Clouds Talk at HPC-AI Advisory Council UK Conference (October ‘20) by Dhabaleswar K. (DK) Panda The Ohio State University E-mail: [email protected] Follow us on http://www.cse.ohio-state.edu/~panda https://twitter.com/mvapich High-End Computing (HEC): PetaFlop to ExaFlop 100 PetaFlops in 415 Peta 2017 Flops in 2020 (Fugaku in Japan with 7.3M cores 1 ExaFlops Expected to have an ExaFlop system in 2021! Network Based Computing Laboratory HPC-AI-UK (Oct ‘20) 2 Increasing Usage of HPC, Big Data and Deep/Machine Learning Big Data (Hadoop, Spark, HPC HBase, (MPI, PGAS, etc.) Memcached, etc.) Convergence of HPC, Big Deep/Machine Data, and Deep/Machine Learning Learning! (TensorFlow, PyTorch, BigDL, cuML, etc.) Increasing Need to Run these applications on the Cloud!! Network Based Computing Laboratory HPC-AI-UK (Oct ‘20) 3 Converged Middleware for HPC, Big Data and Deep/Machine Learning? Physical Compute Network Based Computing Laboratory HPC-AI-UK (Oct ‘20) 4 Converged Middleware for HPC, Big Data and Deep/Machine Learning? Network Based Computing Laboratory HPC-AI-UK (Oct ‘20) 5 Converged Middleware for HPC, Big Data and Deep/Machine Learning? Network Based Computing Laboratory HPC-AI-UK (Oct ‘20) 6 Converged Middleware for HPC, Big Data and Deep/Machine Learning? Hadoop Job Deep/Machine Learning Job Spark Job Network Based Computing Laboratory HPC-AI-UK (Oct ‘20) 7 Presentation Overview • MVAPICH Project – MPI and PGAS (MVAPICH) Library with CUDA-Awareness • HiBD Project – High-Performance Big Data Analytics Library • HiDL Project – High-Performance Deep Learning – High-Performance Machine Learning • Optimizations and Deployments in Public Cloud – AWS and Azure • Conclusions Network Based Computing Laboratory HPC-AI-UK (Oct ‘20) 8 Designing (MPI+X) for Exascale • Scalability for million to billion processors – Support for highly-efficient inter-node and intra-node communication (both two-sided and one-sided) • Scalable Collective communication – Offloaded – Non-blocking – Topology-aware • Balancing intra-node and inter-node communication for next generation multi-/many-core (128-1024 cores/node) – Multiple end-points per node • Support for efficient multi-threading • Integrated Support for GPGPUs and Accelerators • Fault-tolerance/resiliency • QoS support for communication and I/O • Support for Hybrid MPI+PGAS programming • MPI + OpenMP, MPI + UPC, MPI + OpenSHMEM, CAF, MPI + UPC++… • Virtualization • Energy-Awareness Network Based Computing Laboratory HPC-AI-UK (Oct ‘20) 9 Overview of the MVAPICH2 Project • High Performance open-source MPI Library • Support for multiple interconnects – InfiniBand, Omni-Path, Ethernet/iWARP, RDMA over Converged Ethernet (RoCE), and AWS EFA • Support for multiple platforms – x86, OpenPOWER, ARM, Xeon-Phi, GPGPUs (NVIDIA and AMD (upcoming)) • Started in 2001, first open-source version demonstrated at SC ‘02 • Used by more than 3,100 organizations in 89 countries • Supports the latest MPI-3.1 standard • More than 900,000 (> 0.9 million) downloads from the • http://mvapich.cse.ohio-state.edu OSU site directly • Additional optimized versions for different systems/environments: • Empowering many TOP500 clusters (June ‘20 ranking) – MVAPICH2-X (Advanced MPI + PGAS), since 2011 – 4th , 10,649,600-core (Sunway TaihuLight) at NSC, Wuxi, China – MVAPICH2-GDR with support for NVIDIA GPGPUs, since 2014 – 8th, 448, 448 cores (Frontera) at TACC – MVAPICH2-MIC with support for Intel Xeon-Phi, since 2014 – 12th, 391,680 cores (ABCI) in Japan – MVAPICH2-Virt with virtualization support, since 2015 – 18th, 570,020 cores (Nurion) in South Korea and many others – MVAPICH2-EA with support for Energy-Awareness, since 2015 – MVAPICH2-Azure for Azure HPC IB instances, since 2019 • Available with software stacks of many vendors and – MVAPICH2-X-AWS for AWS HPC+EFA instances, since 2019 Linux Distros (RedHat, SuSE, OpenHPC, and Spack) • Tools: • Partner in the 8th ranked TACC Frontera system – OSU MPI Micro-Benchmarks (OMB), since 2003 • Empowering Top500 systems for more than 15 years – OSU InfiniBand Network Analysis and Monitoring (INAM), since 2015 Network Based Computing Laboratory HPC-AI-UK (Oct ‘20) 10 Architecture of MVAPICH2 Software Family for HPC and DL/ML High Performance Parallel Programming Models Message Passing Interface PGAS Hybrid --- MPI + X (MPI) (UPC, OpenSHMEM, CAF, UPC++) (MPI + PGAS + OpenMP/Cilk) High Performance and Scalable Communication Runtime Diverse APIs and Mechanisms Point-to- Remote Collectives Energy- I/O and Fault Active Introspection point Job Startup Memory Virtualization Algorithms Awareness Tolerance Messages & Analysis Primitives Access File Systems Support for Modern Networking Technology Support for Modern Multi-/Many-core Architectures (InfiniBand, iWARP, RoCE, Omni-Path, Elastic Fabric Adapter) (Intel-Xeon, OpenPOWER, Xeon-Phi, ARM, NVIDIA GPGPU) Transport Protocols Modern Features Transport Mechanisms Modern Features SR- Multi Shared RC SRD UD DC UMR ODP CMA IVSHMEM XPMEM Optane* NVLink CAPI* IOV Rail Memory * Upcoming Network Based Computing Laboratory HPC-AI-UK (Oct ‘20) 11 MVAPICH2 Software Family Requirements Library MPI with IB, iWARP, Omni-Path, and RoCE MVAPICH2 Advanced MPI Features/Support, OSU INAM, PGAS and MPI+PGAS MVAPICH2-X with IB, Omni-Path, and RoCE MPI with IB, RoCE & GPU and Support for Deep/Machine Learning MVAPICH2-GDR HPC Cloud with MPI & IB MVAPICH2-Virt Energy-aware MPI with IB, iWARP and RoCE MVAPICH2-EA MPI Energy Monitoring Tool OEMT InfiniBand Network Analysis and Monitoring OSU INAM Microbenchmarks for Measuring MPI and PGAS Performance OMB Network Based Computing Laboratory HPC-AI-UK (Oct ‘20) 12 Converged Middleware for HPC, Big Data and Deep/Machine Learning Big Data HPC (Hadoop, Spark, (MPI, PGAS, HBase, etc.) Memcached, etc.) Deep/Machine Learning (TensorFlow, PyTorch, BigDL, cuML, etc.) Network Based Computing Laboratory HPC-AI-UK (Oct ‘20) 13 Startup Performance on TACC Frontera MVAPICH2 2.3.4 Intel MPI 2020 MVAPICH2 2.3.4 Intel MPI 2020 25 2000 20 1500 15 1000 10 14X 46X Time (s) 5 Time (s) 500 0 0 56 112 224 448 896 1792 3584 7168 14336 28672 57344 114688229376 Number of Processes Number of Processes • MPI_Init takes 31 seconds on 229,376 processes on 4,096 nodes • All numbers reported with 56 processes per node New designs available in MVAPICH2-2.3.4 Network Based Computing Laboratory HPC-AI-UK (Oct ‘20) 14 Hardware Multicast-aware MPI_Bcast on TACC Frontera 20 1000 Default Multicast Default Multicast 15 750 10 500 Latency (us) 250 5 Latency (us) 1.9X 2X (Nodes=2K, PPN=28) 0 0 2 8 32 128 512 2K 8K 32K 128K 512K Message Size Message Size 15 80 Default Multicast Default Multicast Size=16B, PPN=28 Size=32kB, PPN=28 10 60 1.8X 40 2X 5 Latency (us) Latency (us) 20 0 0 2 4 8 16 32 64 128 256 512 1k 2 4 8 16 32 64 128 256 512 1K Number of Nodes Number of Nodes • MCAST-based designs improve latency of MPI_Bcast by up to 2X at 2,048 nodes • Use MV2_USE_MCAST=1 to enable MCAST-based designs Network Based Computing Laboratory HPC-AI-UK (Oct ‘20) 15 Performance of Collectives with SHARP on TACC Frontera 120 1000 100 MVAPICH2-X 80 100 MVAPICH2-X-SHARP 6X 60 40 10 MVAPICH2-X 5X Latency (us) Latency (us) MPI_Reduce MPI_Allreduuce 20 MVAPICH2-X-SHARP (PPN=1, 7861) Nodes = 0 (PPN=1, 7861) Nodes = 1 Message size Message size 250 Optimized SHARP designs in MVAPICH2-X 200 MVAPICH2-X Up to 9X performance improvement with SHARP over MVAPICH2-X default for 1ppn 150 MVAPICH2-X-SHARP MPI_Barrier, 6X for 1ppn MPI_Reduce and 5X for 1ppn MPI_Allreduce MPI_Barrier 100 9X Latency (us) 50 B. Ramesh , K. Suresh , N. Sarkauskas , M. Bayatpour , J. Hashmi , H. Subramoni , and D. K. Panda, Scalable MPI Collectives using 0 SHARP: Large Scale Performance Evaluation on the TACC Frontera 4 8 16 32 64 128 256 512 System, ExaMPI2020 - Workshop on Exascale MPI 2020, Nov 2020, 1024 2048 4096 7861 Number of nodes Accepted to be presented. Optimized Runtime Parameters: MV2_ENABLE_SHARP = 1 Network Based Computing Laboratory HPC-AI-UK (Oct ‘20) 16 Performance of MPI_Ialltoall using HW Tag Matching 4000 2000 1.6 X 1.7 X 3000 1500 8 Nodes 16 Nodes 2000 1000 1000 500 Latency (us) Latency (us) 0 0 16K 32K 64K 128K 256K 512K 1M 16K 32K 64K 128K 256K 512K 1M Message Size (byte) Message Size (byte) MVAPICH2 MVAPICH2+HW-TM MVAPICH2 MVAPICH2+HW-TM 8000 1.8 X 15000 1.5 X 6000 32 Nodes 64 Nodes 10000 4000 5000 2000 Latency (us) Latency (us) 0 0 16K 32K 64K 128K 256K 512K 1M 16K 32K 64K 128K 256K 512K 1M Message Size (byte) Message Size (byte) • Up to 1.8x Performance Improvement, Sustained benefits as system size increases M. Bayatpour , M. Ghazimirsaeed , S. Xu , H. Subramoni , and D. K. Panda, Design and Characterization of InfiniBand Hardware Tag Matching in MPI, CCGrid ‘20. Will be available in upcoming MVAPICH2-X Network Based Computing Laboratory HPC-AI-UK (Oct ‘20) 17 Neighborhood Collectives – Performance Benefits • SpMM • NAS DT up to 34x speedup up to 15% improvement M. Ghazimirsaeed, Q. Zhou, A. Ruhela, M. Bayatpour, H. Subramoni, and D. K. Panda, A Hierarchical and Load-Aware Design for Large Message Neighborhood Collectives, SC ’20, Will be available in upcoming MVAPICH2-X Accepted to be presented Network Based Computing Laboratory HPC-AI-UK (Oct ‘20) 18 GPU-Aware (CUDA-Aware) MPI Library: MVAPICH2-GPU • Standard MPI interfaces used for unified data movement • Takes advantage of Unified Virtual Addressing (>= CUDA 4.0) • Overlaps data movement from GPU with RDMA transfers At Sender: MPI_Send(s_devbuf, size, …); inside MVAPICH2
Details
-
File Typepdf
-
Upload Time-
-
Content LanguagesEnglish
-
Upload UserAnonymous/Not logged-in
-
File Pages58 Page
-
File Size-