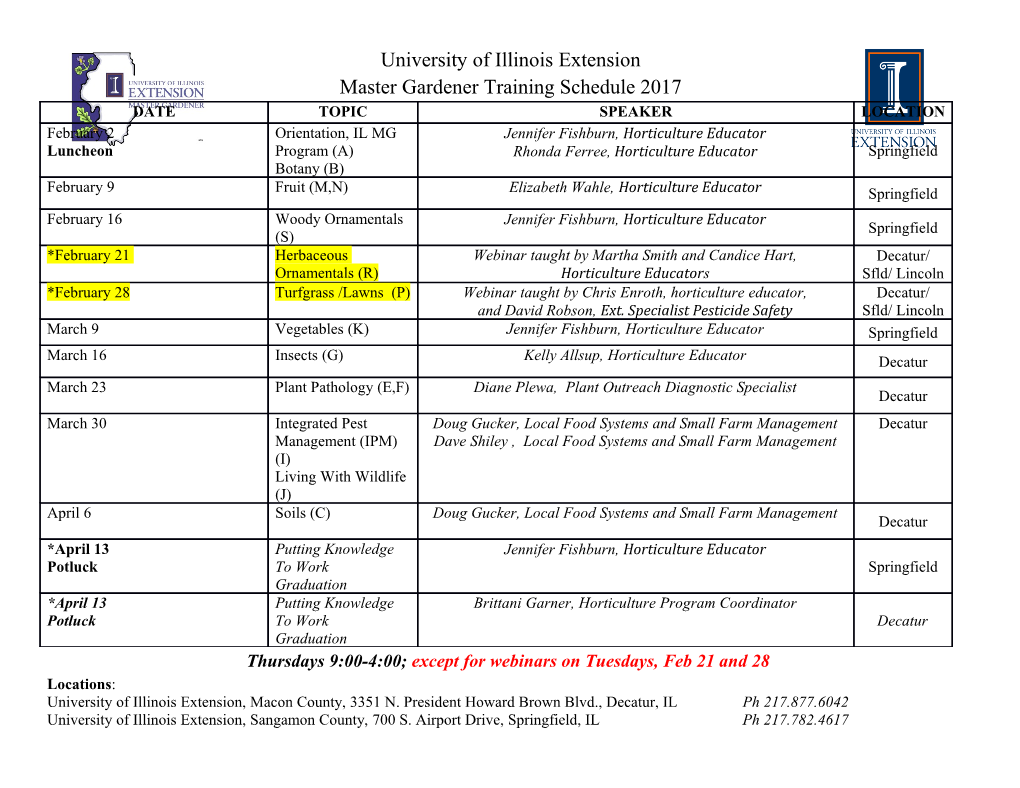
How will Deep Learning Change Internet Video Delivery? Hyunho Yeo Sunghyun Do Dongsu Han KAIST KAIST KAIST 1 INTRODUCTION practice of watching a series of shows in one sitting—is com- Internet video has experienced tremendous growth over the mon [34, 40], tradition video delivery does not take advantage last few decades and is still growing at a rapid pace. Internet of redundancy that occur at large timescales. As a result, when video now accounts for 73% of Internet traffic and is expected the network is congested, video quality degrades drastically, to quadruple in the next five years [9, 41]. Augmented reality even though similar footage is being played. To tackle the and virtual reality streaming, projected to increase twenty- problem, we design a content-aware solution that leverages fold in five years [9], will also accelerate this trend. redundancy across videos. In particular, our design leverages From content delivery networks (CDNs) [35] to HTTP image super-resolution using deep neural networks and use adaptive streaming [3, 21, 37] and data-driven optimization client computation to enhance the video quality. The content- for quality of experience [22], the networking community has aware delivery network classifies videos and generates a small brought fundamental advancements in Internet video delivery. super-resolution network (∼ 7.8 MB) using images from sim- However, video delivery still leaves large room for improve- ilar videos. We show that the content-aware video delivery ment. First, the video delivery infrastructure has largely been achieves better quality using the same amount of bandwidth. agnostic to the video content it delivers, treating it as a stream We believe this has far-reaching implications on dynamic of bits. Second, the basis of how we represent video has adaptive streaming and quality of experience optimization. remained as an unexplored topic within the networking com- Second, a video frame contains many objects that show munity. In fact, the fundamental basis of video encoding has up frequently throughout the video, but traditional streaming largely remain the same. In particular, the practice of video cannot capture this because it cannot capture common fea- encoding is to use signal processing techniques (e.g., discrete tures from these objects. Deep neural networks provide an cosine transform and inter-frame prediction) to spacial and alternative to encode object representations. To demonstrate temporal redundancies that occur at short time-scales (e.g., this, we leverage Generative Adversarial Networks (GANs), within a frame or a group of pictures). known to synthesize images that look authentic to human [17], This paper shows that advancement in deep neural net- for synthesizing objects within a video. We use GAN trained works present new opportunities that can fundamentally change using similar videos to synthesize a high-quality video from Internet video delivery. In particular, deep neural networks an alternative form that contains much less information. allow content delivery network to easily capture the content To demonstrate the feasibility of the approach, we proto- of video and thus enable content-aware video delivery. Based type the system and quantify benefits and costs of the ap- on the observation, we explore new design space for content- proach. We articulate how different parts of the video delivery aware video delivery networks. infrastructure should change to accommodate the design. In First, video contains large amounts of redundancy that oc- summary, this paper takes a first attempt to answer the fol- cur at large timescales. For example, a basketball game video lowing question: how will advances in deep neural networks shares similar background throughout the video. Moreover, change Internet video delivery? In answering the question, series of games, episodes, and streams often share common we find deep learning opens up large design space andhas features. For example, streams of the same game from Twitch far-reaching implications in the video delivery ecosystem. Fi- share large amounts of redundancy. While binge-watching— nally, we call upon the networking community to embrace recent advances in deep learning and to rethink Internet video delivery in the context of what the new technology enables. Permission to make digital or hard copies of all or part of this work for 2 MOTIVATION AND INTUITION personal or classroom use is granted without fee provided that copies are not made or distributed for profit or commercial advantage and that copies bear Limitations of conventional adaptive streaming: The tra- this notice and the full citation on the first page. Copyrights for components ditional approach to improving video stream quality includes of this work owned by others than the author(s) must be honored. Abstracting designing new bitrate selection algorithms [3, 19, 21], choos- with credit is permitted. To copy otherwise, or republish, to post on servers or to redistribute to lists, requires prior specific permission and/or a fee. Request ing better servers and CDN [2, 26, 48], and utilizing a central- permissions from [email protected]. ized control plane [27, 33]. These approaches focus on how HotNets-XVI, November 30–December 1, 2017, Palo Alto, CA, USA we could fully utilize the given network resources. However, © 2017 Copyright held by the owner/author(s). Publication rights licensed to there are two significant limitations. Association for Computing Machinery. First, recent devices including mobile devices have signifi- ACM ISBN 978-1-4503-5569-8/17/11. $15.00 cant computational power. Market reports [36] show around https://doi:org/10:1145/3152434:3152440 Content Delivery Network Client 1: Watchingching tennistenn match Cluster Module Content-aware Cache Module z Cluster videos into content DNN Module Cache videos and sets sharing common content Train DNN on each corresponding content- Client 2: Watchingng basketball basketb match A redundancy content separately aware DNN models Client 3: Watchingng basketball basketb match B : video : set of videos with same content : content-aware DNN : Low Quality Video : High Quality Video : network under congestion Figure 1: High-level vision of a DNN-Based Content-Aware Content Distribution Network 50% of users watch video on PCs, which have large com- DNN is a computational model with multiple layers of hi- putation power. Mobile devices that account for the rest are erarchy, each of which processes the input in a non-linear also equipped with power-efficient mobile graphic process- fashion and delivers its output to the upper layer. It is de- ing units (GPUs) “whose performance exceed that of older- signed to learn high-level abstract features from a complex generation game consoles” (e.g., XBox 360) [14]. The pop- low level representation of data [5]. However, developing and ularity of mobile games and the advent of emerging media, utilizing a generic model that works well across all videos is such as virtual and augmented reality, will accelerated this too expensive for practical purposes, given the amount and trend. This leaves a great opportunity for trading off com- diversity of Internet video—the size of DNN generally has putation for reduced bandwidth under network congestion. a positive correlation with its expressive power. In addition, However, the current video delivery infrastructure does not capturing all objects in a single network amounts to devising offer any way to utilize client’s computational power. Thus, a DNN-based generic video compression algorithm, which is when the network is congested, the stream quality suffers di- non-trivial. Even the quality of state-of-the-art DNN-based rectly. With client’s growing computational capacity and ever image compression is only as good as JPEG2000 only when increasing demand for bandwidth, we envision a video deliv- the compression ratio is set very high [44]. ery system in which clients take an active role in improving Instead, this paper takes a content-aware approach. The the video quality using their own computational power. content distribution network clusters videos of similar nature Second, video contains large amount of redundancy that and generates DNN models for each cluster. The model con- occur at large timescales, and its high-level features contain tains abstract representations of video by capturing high-level valuable information that can be leveraged for video coding. features rather than a pixel-level encoding. In the next two For example, meaningful objects recognized by human, such sections, we explore a concrete design realizing the vision as sport player, stadium and score board, reappear frequently. with various examples of DNN models. However, standard video coding, such as MPEG and H.26x, only captures two kinds of redundancy and lacks any mecha- 3 DNN-BASED CONTENT-AWARE CDN nisms to leverage motion picture’s semantics. Spatial redun- dancy exploits pixel-level similarity within a picture [47]. The Leveraging DNN and utilizing content redundancy are two intra-frame coding compresses a picture using discrete cosine core components of our design that mark a drastic takeoff transform (DCT), quantization, and entropy encoding [18]. from traditional video delivery. Figure 1 presents a high-level Temporal redundancy represents similarities between suc- architecture of a DNN-based content-aware video delivery cessive frames. Inter-frame coding encodes the difference that realize our vision. between adjacent frames to compresses
Details
-
File Typepdf
-
Upload Time-
-
Content LanguagesEnglish
-
Upload UserAnonymous/Not logged-in
-
File Pages8 Page
-
File Size-