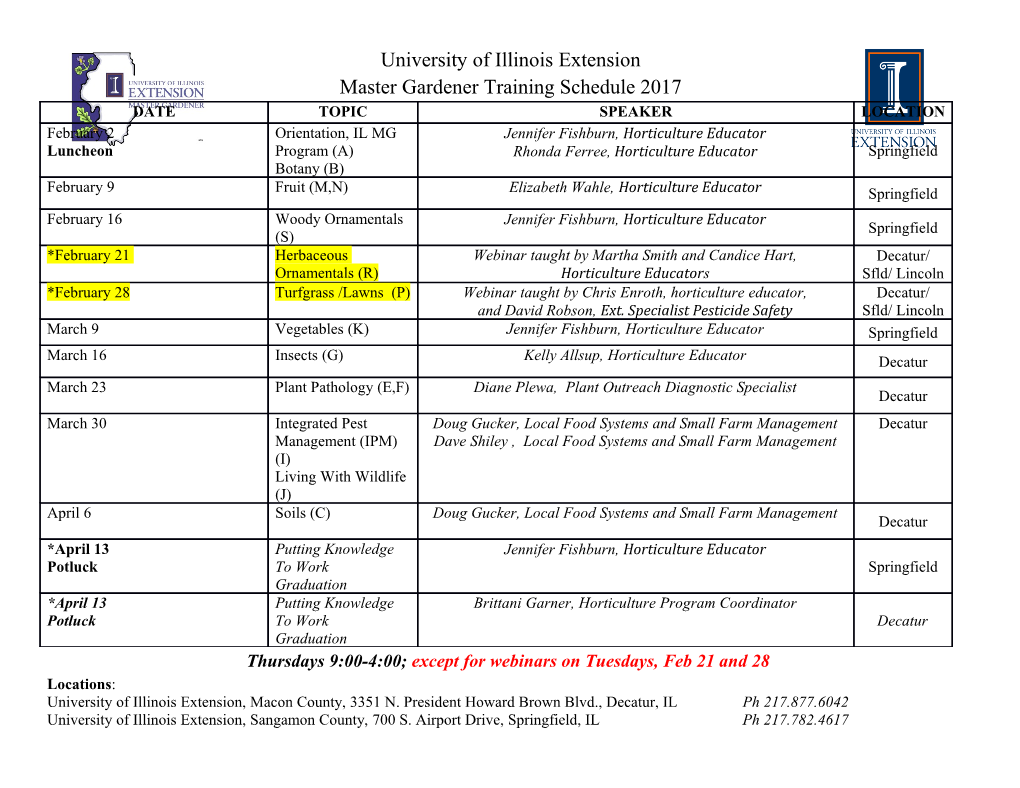
Bayesian Linear Regression (Hyperparameter Estimation, Sparse Priors), Bayesian Logistic Regression Piyush Rai Topics in Probabilistic Modeling and Inference (CS698X) Jan 21, 2019 Prob. Mod. & Inference - CS698X (Piyush Rai, IITK) Bayesian Linear Regression (Hyperparameter Estimation, Sparse Priors), Bayesian Logistic Regression 1 Assuming hyperparameters as fixed, the posterior is Gaussian p(wjy; X; β; λ) = N (µN ; ΣN ) N X > −1 > −1 ΣN = (β x nx n + λID ) = (βX X + λID ) (posterior's covariance matrix) n=1 " N # X h > i > λ −1 > µ = ΣN β ynx n = ΣN βX y = (X X + ID ) X y (posterior's mean) N β n=1 The posterior predictive distribution is also Gaussian Z > −1 > p(y∗jx ∗; X; y; β; λ) = p(y∗jw; x ∗; β)p(wjy; X; β; λ)dw = N (µN x ∗;β + x ∗ ΣN x ∗) Gives both predictive mean and predictive variance (imp: pred-var is different for each input) Recap: Bayesian Linear Regression QN > −1 −1 Assume Gaussian likelihood: p(yjX; w; β) = n=1 N (ynjw x n; β ) = N (yjXw; β IN ) QD −1 −1 Assume zero-mean spherical Gaussian prior: p(wjλ) = d=1 N (wd j0; λ ) = N (wj0; λ ID ) Prob. Mod. & Inference - CS698X (Piyush Rai, IITK) Bayesian Linear Regression (Hyperparameter Estimation, Sparse Priors), Bayesian Logistic Regression 2 N X > −1 > −1 ΣN = (β x nx n + λID ) = (βX X + λID ) (posterior's covariance matrix) n=1 " N # X h > i > λ −1 > µ = ΣN β ynx n = ΣN βX y = (X X + ID ) X y (posterior's mean) N β n=1 The posterior predictive distribution is also Gaussian Z > −1 > p(y∗jx ∗; X; y; β; λ) = p(y∗jw; x ∗; β)p(wjy; X; β; λ)dw = N (µN x ∗;β + x ∗ ΣN x ∗) Gives both predictive mean and predictive variance (imp: pred-var is different for each input) Recap: Bayesian Linear Regression QN > −1 −1 Assume Gaussian likelihood: p(yjX; w; β) = n=1 N (ynjw x n; β ) = N (yjXw; β IN ) QD −1 −1 Assume zero-mean spherical Gaussian prior: p(wjλ) = d=1 N (wd j0; λ ) = N (wj0; λ ID ) Assuming hyperparameters as fixed, the posterior is Gaussian p(wjy; X; β; λ) = N (µN ; ΣN ) Prob. Mod. & Inference - CS698X (Piyush Rai, IITK) Bayesian Linear Regression (Hyperparameter Estimation, Sparse Priors), Bayesian Logistic Regression 2 " N # X h > i > λ −1 > µ = ΣN β ynx n = ΣN βX y = (X X + ID ) X y (posterior's mean) N β n=1 The posterior predictive distribution is also Gaussian Z > −1 > p(y∗jx ∗; X; y; β; λ) = p(y∗jw; x ∗; β)p(wjy; X; β; λ)dw = N (µN x ∗;β + x ∗ ΣN x ∗) Gives both predictive mean and predictive variance (imp: pred-var is different for each input) Recap: Bayesian Linear Regression QN > −1 −1 Assume Gaussian likelihood: p(yjX; w; β) = n=1 N (ynjw x n; β ) = N (yjXw; β IN ) QD −1 −1 Assume zero-mean spherical Gaussian prior: p(wjλ) = d=1 N (wd j0; λ ) = N (wj0; λ ID ) Assuming hyperparameters as fixed, the posterior is Gaussian p(wjy; X; β; λ) = N (µN ; ΣN ) N X > −1 > −1 ΣN = (β x nx n + λID ) = (βX X + λID ) (posterior's covariance matrix) n=1 Prob. Mod. & Inference - CS698X (Piyush Rai, IITK) Bayesian Linear Regression (Hyperparameter Estimation, Sparse Priors), Bayesian Logistic Regression 2 The posterior predictive distribution is also Gaussian Z > −1 > p(y∗jx ∗; X; y; β; λ) = p(y∗jw; x ∗; β)p(wjy; X; β; λ)dw = N (µN x ∗;β + x ∗ ΣN x ∗) Gives both predictive mean and predictive variance (imp: pred-var is different for each input) Recap: Bayesian Linear Regression QN > −1 −1 Assume Gaussian likelihood: p(yjX; w; β) = n=1 N (ynjw x n; β ) = N (yjXw; β IN ) QD −1 −1 Assume zero-mean spherical Gaussian prior: p(wjλ) = d=1 N (wd j0; λ ) = N (wj0; λ ID ) Assuming hyperparameters as fixed, the posterior is Gaussian p(wjy; X; β; λ) = N (µN ; ΣN ) N X > −1 > −1 ΣN = (β x nx n + λID ) = (βX X + λID ) (posterior's covariance matrix) n=1 " N # X h > i > λ −1 > µ = ΣN β ynx n = ΣN βX y = (X X + ID ) X y (posterior's mean) N β n=1 Prob. Mod. & Inference - CS698X (Piyush Rai, IITK) Bayesian Linear Regression (Hyperparameter Estimation, Sparse Priors), Bayesian Logistic Regression 2 Gives both predictive mean and predictive variance (imp: pred-var is different for each input) Recap: Bayesian Linear Regression QN > −1 −1 Assume Gaussian likelihood: p(yjX; w; β) = n=1 N (ynjw x n; β ) = N (yjXw; β IN ) QD −1 −1 Assume zero-mean spherical Gaussian prior: p(wjλ) = d=1 N (wd j0; λ ) = N (wj0; λ ID ) Assuming hyperparameters as fixed, the posterior is Gaussian p(wjy; X; β; λ) = N (µN ; ΣN ) N X > −1 > −1 ΣN = (β x nx n + λID ) = (βX X + λID ) (posterior's covariance matrix) n=1 " N # X h > i > λ −1 > µ = ΣN β ynx n = ΣN βX y = (X X + ID ) X y (posterior's mean) N β n=1 The posterior predictive distribution is also Gaussian Z > −1 > p(y∗jx ∗; X; y; β; λ) = p(y∗jw; x ∗; β)p(wjy; X; β; λ)dw = N (µN x ∗;β + x ∗ ΣN x ∗) Prob. Mod. & Inference - CS698X (Piyush Rai, IITK) Bayesian Linear Regression (Hyperparameter Estimation, Sparse Priors), Bayesian Logistic Regression 2 Recap: Bayesian Linear Regression QN > −1 −1 Assume Gaussian likelihood: p(yjX; w; β) = n=1 N (ynjw x n; β ) = N (yjXw; β IN ) QD −1 −1 Assume zero-mean spherical Gaussian prior: p(wjλ) = d=1 N (wd j0; λ ) = N (wj0; λ ID ) Assuming hyperparameters as fixed, the posterior is Gaussian p(wjy; X; β; λ) = N (µN ; ΣN ) N X > −1 > −1 ΣN = (β x nx n + λID ) = (βX X + λID ) (posterior's covariance matrix) n=1 " N # X h > i > λ −1 > µ = ΣN β ynx n = ΣN βX y = (X X + ID ) X y (posterior's mean) N β n=1 The posterior predictive distribution is also Gaussian Z > −1 > p(y∗jx ∗; X; y; β; λ) = p(y∗jw; x ∗; β)p(wjy; X; β; λ)dw = N (µN x ∗;β + x ∗ ΣN x ∗) Gives both predictive mean and predictive variance (imp: pred-var is different for each input) Prob. Mod. & Inference - CS698X (Piyush Rai, IITK) Bayesian Linear Regression (Hyperparameter Estimation, Sparse Priors), Bayesian Logistic Regression 2 A visualization of the posterior predictive of a Bayesian linear regression model Small predictive Line showing variance here predictive mean y Large predictive variance here x A Visualization of Uncertainty in Bayesian Linear Regression Posterior p(wjX; y) and lines (w0 intercept, w1 slope) corresponding to some random w's Prior (N=0) Posterior (N=1) Posterior (N=2) Posterior (N=20) Prob. Mod. & Inference - CS698X (Piyush Rai, IITK) Bayesian Linear Regression (Hyperparameter Estimation, Sparse Priors), Bayesian Logistic Regression 3 Small predictive Line showing variance here predictive mean y Large predictive variance here x A Visualization of Uncertainty in Bayesian Linear Regression Posterior p(wjX; y) and lines (w0 intercept, w1 slope) corresponding to some random w's Prior (N=0) Posterior (N=1) Posterior (N=2) Posterior (N=20) A visualization of the posterior predictive of a Bayesian linear regression model Prob. Mod. & Inference - CS698X (Piyush Rai, IITK) Bayesian Linear Regression (Hyperparameter Estimation, Sparse Priors), Bayesian Logistic Regression 3 Posterior predictive: Red curve is predictive mean, shaded region denotes predictive uncertainty y y y y x x x x A Visualization of Uncertainty (Contd) We can similarly visualize a Bayesian nonlinear regression model Figures below: Green curve is the true function and blue circles are observations (xn; yn) Posterior of the nonlinear regression model: Some curves drawn from the posterior y y y y x x x x Prob. Mod. & Inference - CS698X (Piyush Rai, IITK) Bayesian Linear Regression (Hyperparameter Estimation, Sparse Priors), Bayesian Logistic Regression 4 y y y y x x x x A Visualization of Uncertainty (Contd) We can similarly visualize a Bayesian nonlinear regression model Figures below: Green curve is the true function and blue circles are observations (xn; yn) Posterior of the nonlinear regression model: Some curves drawn from the posterior y y y y x x x x Posterior predictive: Red curve is predictive mean, shaded region denotes predictive uncertainty Prob. Mod. & Inference - CS698X (Piyush Rai, IITK) Bayesian Linear Regression (Hyperparameter Estimation, Sparse Priors), Bayesian Logistic Regression 4 Estimating Hyperparameters for Bayesian Linear Regression Prob. Mod. & Inference - CS698X (Piyush Rai, IITK) Bayesian Linear Regression (Hyperparameter Estimation, Sparse Priors), Bayesian Logistic Regression 5 Example: For the linear regression model, the full set of parameters would be (w; λ, β) Can assume priors on all these parameters and infer their \joint" posterior distribution p(yjX; w; β; λ)p(w; λ, β) p(yjX; w; β; λ)p(wjλ)p(β)p(λ) p(w; β; λjX; y) = = p(yjX) R p(yjX; w; β)p(wjλ)p(β)p(λ) dw dλdβ Infering the above is usually intractable (rare to have conjugacy). Requires approximations. Also, What priors (or \hyperpriors") to choose for β and λ? What about the hyperparameters of those priors? Learning Hyperparameters in Probabilistic Models Can treat hyperparams as just a bunch of additional unknowns Can be learned using a suitable inference algorithm (point estimation or fully Bayesian) Prob. Mod. & Inference - CS698X (Piyush Rai, IITK) Bayesian Linear Regression (Hyperparameter Estimation, Sparse Priors), Bayesian Logistic Regression 6 Can assume priors on all these parameters and infer their \joint" posterior distribution p(yjX; w; β; λ)p(w; λ, β) p(yjX; w; β; λ)p(wjλ)p(β)p(λ) p(w; β; λjX; y) = = p(yjX) R p(yjX; w; β)p(wjλ)p(β)p(λ) dw dλdβ Infering the above is usually intractable (rare to have conjugacy).
Details
-
File Typepdf
-
Upload Time-
-
Content LanguagesEnglish
-
Upload UserAnonymous/Not logged-in
-
File Pages116 Page
-
File Size-