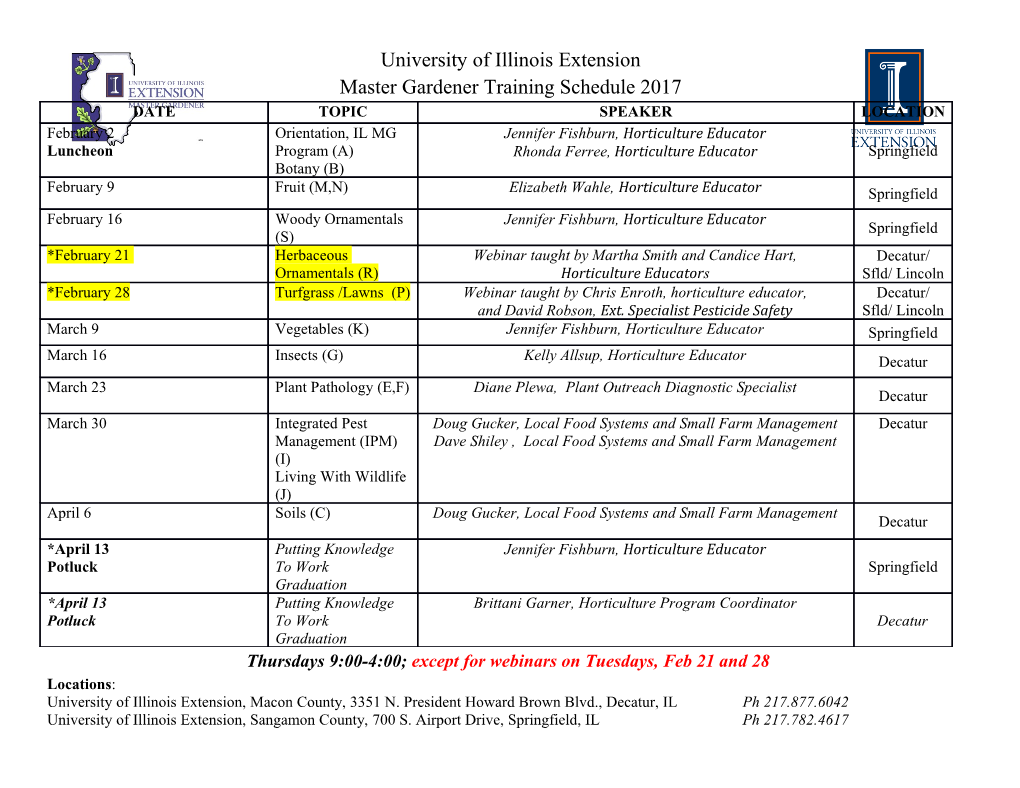
New Diagnostic Methods for Inuential Observations in Linear Regression with some Biased Estimation Methods A Ph.D. Dissertation By Muhammad Kashif Roll No.: PHDS-11-05 Session: 2011-2016 DEPARTMENT OF STATISTICS Bahauddin Zakariya University Multan - Pakistan 2019 New Diagnostic Methods for Inuential Observations in Linear Regression with some Biased Estimation Methods A Thesis submitted in partial fulllment of the requirements for the degree of Doctor of Philosophy in STATISTICS by Muhammad Kashif (Roll No. PHDS-11-05) Session: 20112016 SUPERVISED by Prof. Dr. Muhammad Aman Ullah CO-SUPERVISED by Dr. Muhammad Aslam Department of Statistics Bahauddin Zakariya University Multan 2019 New Diagnostic Methods for Influential Observations in Linear Regression with some Biased Estimation Methods by Muhammad Kashif A thesis submitted to the Department of Statistics, Bahauddin Zakariya University, Multan in fulfillment of the requirements for the Degree of Doctor of Philosophy in Statistics 2019 Author's Declaration I Muhammad Kashif, hereby state that my Ph.D. (Statistics) thesis titled New Diagnostic Methods for Inuential Observations in Linear Regression with some Biased Estimation Methods is my own work and has not been submitted previously by me for taking any degree from this University Bahauddin Zakariya University, Multan, Pakistan or anywhere else in the country/world. At any time if my statement is found to be incorrect even after my Graduate the university has the right to withdraw my Ph.D. (Statistics) degree. Muhammad Kashif Date: 27-07-2019 i Plagiarism Undertaking I solemnly declare that research work presented in the thesis titled New Diagnostic Methods for Inuential Observations in Linear Regression with some Biased Estimation Methods is solely my research work with no signicant contribution from any other person. Small contribution/help wherever taken has been duly acknowledged and that complete thesis has been written by me. I understand the zero tolerance policy of the HEC and Bahauddin Zakariya University, Multan, Pakistan towards plagiarism. Therefore I as an Author of the above titled thesis declare that no portion of my thesis has been plagiarized and any material used as reference is properly referred/cited. I undertake that if I am found guilty of any formal plagiarism in the above titled thesis even after award of Ph.D. (Statistics) degree, the University reserves the rights to withdraw/revoke my Ph.D. (Statistics) degree and that HEC and the University has the right to publish my name on the HEC/University Website on which names of students are placed who submitted plagiarized thesis. Student's Signature: Name: Muhammad Kashif ii This thesis is dedicated to The Holy Prophet Hazrat Muhammad (S.A.W.W) (Whose teaching enlightened my heart and flourished my thoughts) iii Acknowledgements First and foremost, praises and thanks to Almighty ALLAH for giving me this opportunity, the strength and the patience to complete my dissertation finally, after all the challenges and difficulties. My special praise for the messenger of Allah, the Holy Prophet Hazrat MUHAMMAD (S.A.W.W), the greatest educator, the everlasting source of guidance and knowledge for humanity. He taught the principles of morality and eternal values. I deem it a great honor to express my deep and sincere gratitude to my honorable and estimable supervisor, Prof. Dr. Muhammad Aman Ullah, Professor at Department of Statistics, Bahauddin Zakariya University, Multan for his guidance and invaluable advice. His constructive comments and suggestions throughout the thesis work have contributed to the success of this research. His timely and efficient contribution helped me shape this thesis into its final form and I express my sincere appreciation for his assistance in any way that I may have asked. I consider it my privilege to have accomplished this thesis under his right guidance. I feel great pleasure in expressing my sincerest gratitude to my Co-supervisor Dr. Muhammad Aslam, Associate Professor and Chairman, Department of Statistics, Bahauddin Zakariya University, Multan for his detailed review, constructive sugges- tions, important support and excellent advice during the preparation of this thesis. I would like to express my gratitude to all the esteemed faculty members and staff members of the Department of Statistics, Bahauddin Zakariya University, Multan. Finally, I wish to thank to my loving parents, sisters, brothers and my wife for their prayers, encouragement and support spiritual, emotional, intellectual and otherwise. Muhammad Kashif iv Abstract This thesis is concerned with the expansion of diagnostic methods in parametric regression models with some biased estimators. Of which, the Liu estimator, modified ridge estimator, improved Liu estimator and ridge estimator have been developed as an alternative to the ordinary least squares estimator in the presence of multicollinearity in linear regression models. Firstly, we introduce a type of Pena's statistic for each point in Liu regression. Using the forecast change property, we simplify the Pena's statistic in a numerical sense. It is found that the simplified Pena's statistic behaves quite well as far as detection of influential observations is concerned. We express Pena's statistic in terms of the Liu leverages and residuals. For numerical evaluation, simulated studies are given and a real data set has been analyzed for illustration. Secondly, we formulated Pena's statistic for each point while considering the modified ridge regression estimator. Using this statistic, we showed that when modified ridge regression was used to mitigate the effects of multicollinearity, the influence of some observations could be significantly changed. The normality of this statistic was also discussed and it was proved that it could detect a subset of high modified ridge leverage outliers. The Monte Carlo simulations were used for v empirical results and an example of real data was presented for illustration. Next, we introduce a type of Pena's statistic for each point in the improved Liu estimator. Using this statistic, we showed that when the improved Liu estimator was used to mitigate the effects of multicollinearity, the influence of some observations could be significantly changed. The Monte Carlo simulations were used for empirical results and an example of real data was presented for illustration. The ridge estimator having growing and wider applications in statistical data analysis as an alternative technique to the ordinary least squares estimator to combat multicollinearity in linear regression models. In regression diagnostics, a large number of influence diagnostic methods based on numerous statistical tools have been discussed. Finally, we focus on ridge version of Nurunnabi et al. (2011) method for identification of multiple influential observation in linear regression. The efficiency of the proposed method is presented through several well-known data sets, an artificial large data with high-dimension and heterogeneous sample and a Monte Carlo simulation study. vi List of Symbols and Abbreviations Abbreviation/Symbols Description b Prior information vector β Vector of slope coefficients of X1 ^ βd Liu estimate ^ β(k;b) Modified ridge estimate ^ βK;D Improved Liu estimate ^ βk Ridge estimate BACON Block adaptive computationally effective outlier nomina- tor CIP Correct identification in percentage d biasing parameter Di Cook's distance Dd;i Cook's distance with Liu estimate D(k;b)i Cook's distance with modified ridge estimate DK:D;i Cook's distance with Improved Liu estimate DR;i Cook's distance with ridge estimate vii DF F IT S(i) Difference of Fits Test DF F IT SR(i) Difference of Fits Test with ridge estimate H Hat Matrix Hd Hat Matrix with Liu estimate H(k;b) Hat Matrix with modified ridge estimate HK;D Hat Matrix with Improved Liu estimate HR Hat Matrix with ridge estimate hii Leverages hd;i Leverages with Liu estimate h(k;b)i Leverages with modified ridge estimate hK:D;i Leverages with Improved Liu estimate hRii Leverages with ridge estimate I Identity Matrix ILE Improved Liu Estimator k Ridge parameter LE Liu Estimator MAD Median absolute deviation MLR Multiple linear regression MRR Modified ridge regression Mi Nurunnabi's measure MR;i Nurunnabi's measure with ridge estimate viii OLS Ordinary least squares RR Ridge regression Si Pena's statistic Sd;i Pena's statistic with Liu estimate S(k;b)i Pena's statistic with modified ridge estimate SK;D;i Pena's statistic with Improved Liu estimate SR;i Pena's statistic with ridge estimate X1 Centered and standardized matrix of variables y Response vector ix Contents List of Symbols and Abbreviation xii List of Tables xii List of Figures xiii 1 Introduction1 1.1 Presentation................................1 1.2 Significance................................5 1.3 A brief sketch of the Research......................6 2 Influence Diagnostic Measures in Linear Regression8 2.1 Introduction................................8 2.2 The Linear Model and OLS Estimation.................9 2.3 Residuals and Hat Matrix in Linear Regression............ 10 2.4 Diagnostic Approaches in Linear Regression with No Multicollinearity 11 3 Pena's statistic for the Liu regression (Kashif et al. (2018)) 17 3.1 Introduction................................ 17 3.2 Pena's statistic.............................. 19 3.2.1 Pena's statistic using the LE................... 19 3.2.2 Properties of Pena's statistic for the Liu Regression...... 21 3.3 Simulation study............................. 28 3.3.1 Normality of Proposed
Details
-
File Typepdf
-
Upload Time-
-
Content LanguagesEnglish
-
Upload UserAnonymous/Not logged-in
-
File Pages152 Page
-
File Size-