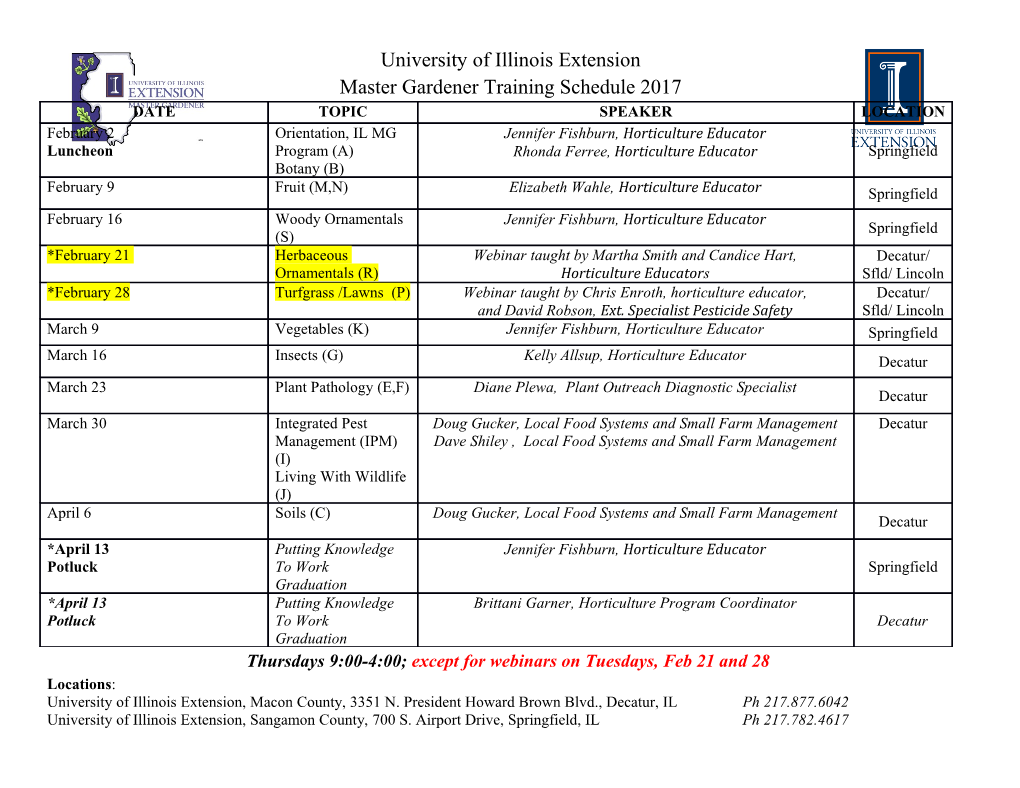
Overview • Forums provide a wealth of information • Semi structured data not taken advantage of by popular search software Board Search • Despite being crawled, many An Internet Forum Index information rich posts are lost in low page rank Forum Examples vBulletin •vBulletin • phpBB •UBB • Invision •YaBB • Phorum • WWWBoard phpBB UBB 1 gentoo evolutionM bayareaprelude warcraft Paw talk Current Solutions • Search engines • Forum’s internal search 2 Google lycos internal boardsearch Evaluation Metric boardsearch Metrics: Recall - C/N, Precision C/E Rival system: • Rival system is the search engine / forum internal search combination • Rival system lacks precision Evaluations: • How good our system is at finding forums • How good our system is at finding relevant posts/threads Problems: • Relevance is in the eye of the beholder • How many correct extractions exist? 3 Implementation • Lucene Improving Software Package • Mysql • Ted Grenager’s Crawler Source Search Quality • Jakarta HTTPClient Dan Fingal and Jamie Nicolson The Problem Sourceforge.org • Search engines for softare packages typically perform poorly • Tend to search project name an blurb only • For example… Gentoo.org Freshmeat.net 4 How can we improve this? Better Sources of Information • Better keyword matching • Every package is associated with a • Better ranking of the results website that contains much more detailed • Better source of information about the information about it package • Spidering these sites should give us a • Pulling in nearest neighbors of top richer representation of the package matches • Freshmeat.net has data regarding popularity, vitality, and user ratings Building the System How do we measure success? • Will spider freshmeat.net and the project • Create a gold corpus of queries to relevant webpages, put into mySQL database packages • Also convert gentoo package database to • Measure precision within the first N results mySQL • Compare results with search on • Text indexing done with Lucene packages.gentoo.org, freshmeat.net, and • Results generator will combine this with google.com other available metrics Any questions? Incorporating Social Clusters in Email Classification By Mahesh Kumar Chhaparia 5 Previous Work Email Classification • Previous work on email classification focus mostly on: • Emails: – Binary classification (spam vs. Non-spam) – Usually small documents – Supervised learning techniques for grouping into multiple existing – Keyword sharing across related emails may be small or indistinctive folders – Hence, on-the-fly training may be slow • Rule-based learning, naïve-Bayes classifier, support vector machines – Classifications change over time, and • Sender and recipient information usually discarded – Different for different users !! • Some existing classification tools • Motivation: – POPFile : Naïve-Bayes classifier – The sender-receiver link mostly has a unique role (social/professional) – RIPPER : Rule-Based learning for a particular user – MailCat : TF-IDF weighting – Hence, it may be used as one of the distinctive characteristics of classification Incorporating Social Clusters Evaluation • Identify initial social clusters (unsupervised) • Recently Enron Email Dataset made public • Weights to distinguish – The only substantial collection of “real” email that is public – From and cc fields, – Fast becoming a benchmark for most of the experiments in – Number of occurrences in distinct emails • Social Network Analysis • Email Classification • Textual Analysis … • Study effects of incorporating sender and recipient information: – Can it substitute part of the training required ? • Study/Comparison of aforementioned metrics with the already – Can it compensate for documental evidence of similarity ? available folder classification on Enron Dataset – Quality of results vs. Training time tradeoff ? – How does it affect regular classification if used as terms too ? Extensions References • Role discovery using Author-Topic-Recipient Model to facilitate • Provost, J. “Naïve-Bayes vs. Rule-Learning in Classification of Email”, classification The University of Texas at Austin, Artificial Intelligence Lab. Technical Report AI-TR-99-284, 1999. • Lexicon expansion to capture similarity in small amounts of data • E. Crawford, J. Kay, and E. McCreath, “Automatic Induction of Rules for • Using past history of conversation to relate messages E-mail Classification,” in Proc. Australasian Document Computing Symposium 2001. • Kiritchenko S. & Matwin S. “Email Classification with Co-Training”, CASCON’02 (IBM Center for Advanced Studies Conference), Toronto, 2002. • Nicolas Turenne. “Learning Semantic Classes for improving Email Classification”, Proc. IJCAI 2003, Text-Mining and Link-Analysis Workshop, 2003. • Manu Arey & Sharma Chakravarthy. “eMailSift: Adapting Graph Mining Techniques for Email Classification”, SIGIR 2004. 6 Outline A research literature search engine • Motivation with abbreviation recognition • Approach – Architecture • Technology Group members • Evaluation Cheng-Tao Chu Pei-Chin Wang Motivation • Existing research literature search engines don’t perform well in author, conference, proceedings abbreviation • Ex: search “C. Manning, IJCAI” in Citeseer, Google Scholar Search result in Google Scholar Goal • Instead of searching by only index, identify the semantic in query • Recognize abbreviation for author and proceedings names 7 Approach Architecture • Crawl DBLP as the data source • Index the data with fields of authors, proceedings, etc. DBLP • Train the tagger to recognize authors and proceedings Crawler • Use the probabilistic model to calculate the probability of each possible name Tagger • Use the tailored edit distance function to calculate the Database weight of each possible proceeding Query • Combine these weights to the score of each selected Search Browser result Retrieved Engine Documents Probabilistic Tailored Model Edit Distance Technology Evaluation • Crawler: UbiCrawler • 1. We will ask for friends to participate in the • Tagger: LingPipe or YamCha evaluation (estimated: 2000 queries/200 friends). • 2. Randomly sample 1000 data from DBLP, • Search Engine: Lucene extract the authors and proceedings info, query • Bayesian Network: BNJ with abbreviated info, check how well the • Web Server: Tomcat retrieved documents match the result from the Google scholar • Database: MySQL • Programming Language: J2SE 1.4.2 Outline • QA Background A Web-based Question • Introduction to our system Answering System • System architecture – Query classification Yu-shan & Wenxiu – Query rewriting 01.25.2005 – Pattern learning • Evaluation 8 QA Background Our QA System • Traditional Search Engine • Open domain – Google, Yahoo, MSN,… • Massive web documents based – Users construct keywords query – Users go through the HitPages to find answer – redundancy guarantee effective • Question Answering SE • Question classification – Askjeeve, AskMSR, … – Users ask in natural language pattern – focus on numeric, definition, human… – Return short answers • Exact answer pattern – Maybe support by reference System Architecture Question Classifier • Given a question, map it to one of the predefined classes. • 6 coarse classes (Abbreviation, Entity, Description, Human, Location, and Numeric Value) and 50 fine classes. • Also show syntactic analysis result such as POS Tagging, Name Entity Tagging, and Chunking. • http://l2r.cs.uiuc.edu/~cogcomp/demo.php?dkey=QC Query Rewrite Answer Pattern Learning • Use the syntactic analysis result to decide • Supervised machine learning approach which part of question to be expanded with • Select correct answers/patterns manually synonym. • Statistics answer pattern rule • Use WordNet for synonyms. 9 Evaluation • Use TREC 2003 QA set. Answers are retrieved from the Web, not from TREC corpus. • Metrics - MRR(Mean Peciprocal Rank) of the first correct Streaming XPath Engine answer - NAns(Number of Questions Correctly Answered), and Oleg Slezberg - %Ans(the proportion of Questions Correctly Amruta Joshi Answered) Traditional XML Processing Streaming XML Processing • Parse whole document into a DOM tree structure • XML parser is event-based, • Query engine search the in-memory such as SAX tree to get the result • XPath processor performs the • Cons: online event-based matching – Extensive memory overhead • Pros: – Unnecessary multiple traversals of the – Less memory overhead document fragment – Only process necessary • E.G. /Descendent::x/ancestor::y/child::z part of input document – Can not return result as early as – Result returned on-the-fly, possible efficient support for non- • E.G. Non-blocking query blocking query What is XPath? A Simple Example • A syntax used for selecting parts of an XML document An XML document SAX API Event <doc> Start element: doc doc • Describes paths to elements similar to an os <para1> Start element: para1 Hello world! data: Hello world! para1 para2 describing paths to files </para1> End element: para1 <para2> … </para2> ... • Almost a small programming language; it has </doc> End element: doc Hello, world functions, tests, and expressions • XPath query Q = /doc/para1/data() • W3C standard • Traditional processing: – Build an in-memory DOM strucuture • Not itself written as XML, but is used heavily in – Return “Hello world” after end document XSLT • Streaming processing – Match /doc in Q when start element doc – Match /doc/para1 in Q when start element para1 – Return “Hello world” when end element para1 10 Objective XPath Challenges • Build an Streaming XPath Engine using • Predicates TurboXPath algorithm • Backward axis • Common subexpressions • // + nested tags (e.g. <a> ... <a> ... </a> ... </a>) • Contributions: •* – comparison of FA-based (XSQ) and tree- • Children in predicates that are not yet seen based (TurboXPath) algorithms (e.g. a[b]/c and c is streamed before b) – performance comparison between • Simultaneous multiple XPath query processing TurboXPath & XSQ Algorithms Evaluation • Finite-Automata Based • Implementations will be evaluated for – XFilter – Feature Completeness – YFilter – Performance (QPS rate) –XSQ • Tree-Based •XMark –XAOS – XML Benchmarking Software – TurboXPath 11.
Details
-
File Typepdf
-
Upload Time-
-
Content LanguagesEnglish
-
Upload UserAnonymous/Not logged-in
-
File Pages11 Page
-
File Size-