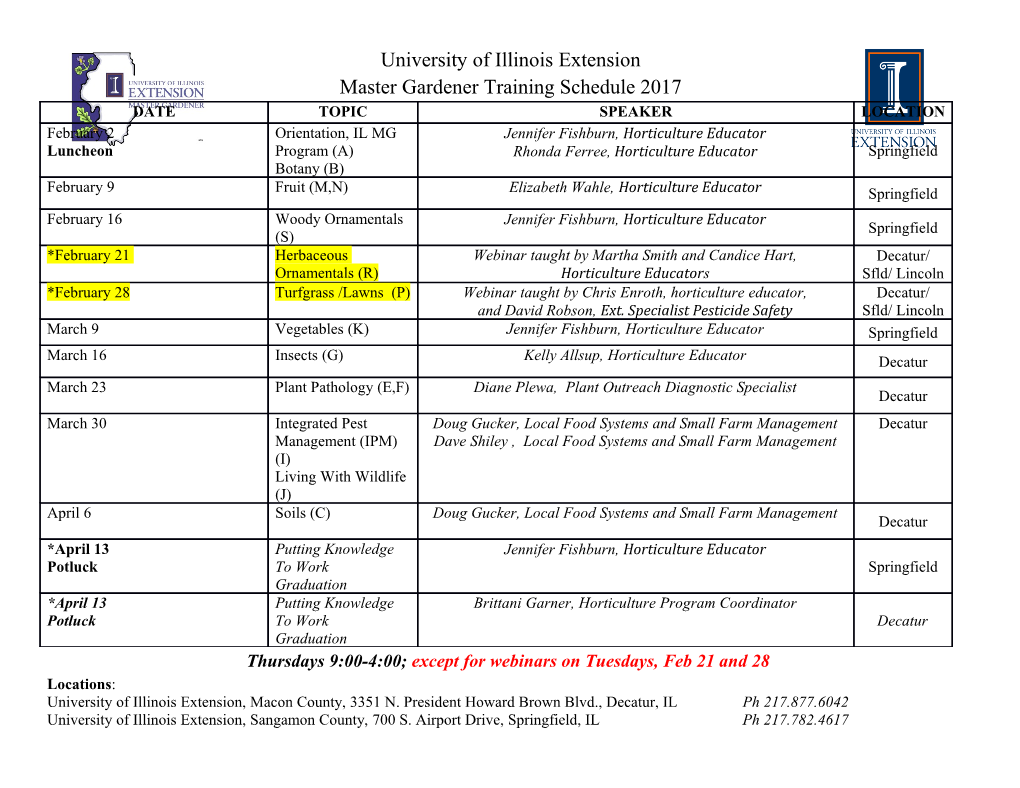
Identification of Versions of the Same Musical Composition by Processing Audio Descriptions Joan Serrà Julià TESI DOCTORAL UPF / 2011 Director de la tesi: Dr. Xavier Serra i Casals Dept. of Information and Communication Technologies Universitat Pompeu Fabra, Barcelona, Spain Copyright c Joan Serrà Julià, 2011. Dissertation submitted to the Deptartment of Information and Communica- tion Technologies of Universitat Pompeu Fabra in partial fulfillment of the requirements for the degree of DOCTOR PER LA UNIVERSITAT POMPEU FABRA, with the mention of European Doctor. Music Technology Group (http://mtg.upf.edu), Dept. of Information and Communica- tion Technologies (http://www.upf.edu/dtic), Universitat Pompeu Fabra (http://www. upf.edu), Barcelona, Spain. Als meus avis. Acknowledgements I remember I was quite shocked when, one of the very first times I went to the MTG, Perfecto Herrera suggested that I work on the automatic identification of versions of musical pieces. I had played versions (both amateur and pro- fessionally) since I was 13 but, although being familiar with many MIR tasks, I had never thought of version identification before. Furthermore, how could they (the MTG people) know that I played song versions? I don’t think I had told them anything about this aspect... Before that meeting with Perfe, I had discussed a few research topics with Xavier Serra and, after he gave me feedback on a number of research proposals I had, I decided to submit one related to the exploitation of the temporal information of music descriptors for music similarity. Therefore, when Perfe suggested the topic of version identification I initially thought that such a suggestion was not related to my proposal at all. However, subsequent meetings with Emilia Gómez and Pedro Cano made me realize that I was wrong, up to the point that if now I had to talk about the work in this thesis I would probably use some of the words of my original proposal: “temporal information”, “music descriptors”, and “music similarity”. Being in close contact with these people I have mentioned has been extremely important, not only for the work related to this thesis, but also for my educa- tion as a researcher in general (not to mention the personal side!). I am really happy to have met them. And I am specially grateful to Xavier for giving me the opportunity to join the MTG. One day, while talking with Xavier, he mentioned a course on time series analysis given in the UPF by some guy called Ralph, who had quite an un- pronounceable surname (Andrzejak). My research at that time was already pivoting around nonlinear time series analysis tools, so I managed to attend to Ralph’s course and off-line told him about my research. This turned out to be the starting point of a very fruitful collaboration between Ralph and myself. I must confess I have learned A LOT from him. Another day, at Ralph’s office, I saw quite a deteriorated (by use) copy of a book by some guys called Kantz & Schreiber. Ralph told me that this was “the bible”, so I bought it and started reading. It was himself who, after seeing that my Kantz & Schreiber book was nearly as deteriorated as his, suggested doing a research stay abroad. We decided to contact Holger Kantz and, to my surprise, he agreed on a collaboration. So I went to work at the MPIPKS for four months under Holger’s supervision. That was a great experience! Some time before, Pedro had invited Massimiliano Zanin to give a talk at the MTG. I do not remember if we had already had a short conversation at v vi that time, but for the subsequent months he remained being just “the complex networks guy with very very long hair”, that is until I had some research problem related to complex networks. Then I contacted him and we started collaborating (and furthermore became friends). Now “the complex networks guy with very very long hair” has been substantially reduced to “Max”. All the people I have mentioned are just a small part of the relevant inter- actions that have shaped this thesis. There are many more people from the MTG that I would like to acknowledge, and whose work, advice and frien- ship I really appreciate. These are Vincent Akkermans, Eduard Aylon, Dmitry Bogdanov, Jordi Bonada, Òscar Celma, Graham Coleman, Maarten de Boer, Ferdinand Fuhrmann, Jordi Funollet, Cristina Garrido, Enric Guaus, Salvador Gurrera, Martín Haro, Jordi Janer, Markus Koppenberger, Cyril Laurier, Os- car Mayor, Ricard Marxer, Owen Meyers, Hendrik Purwins, Gerard Roma, Justin Salamon, Mohamed Sordo, and Nicolas Wack (sorry if I am forgetting someone!). In addition, I have been in contact with people outside the MTG, specially with Josep Lluís Arcos, Juan Pablo Bello, Mathieu Lagrange, Matija Marolt, and Meinard Müller. I would also like to acknowledge Jean Arroyo for proofreading this thesis. Last, but not least, I want to mention my friends and my family, who have supported me in all aspects. vii This thesis has been carried out at the Music Technology Group of Universitat Pompeu Fabra (UPF) in Barcelona, Spain from Sep. 2007 to Jan. 2010 and from Jun. 2010 to Dec. 2010, and at the Max Planck Institute for the Physics of Com- plex Systems (MPIPKS) in Dresden, Germany from Feb. 2010 to May 2010. This work has been supported by an R+D+I scholarship from UPF, by the European Commission projects CANTATA (FIT-350205-2007-10), SALERO (IST-2007-0309BSCW) and PHAROS (IST-2006-045035), by the project of the Spanish Ministry of Industry, Tourism and Trade MUSIC 3.0 (TSI-070100- 2008-318) and by the project of the Spanish Ministry of Science and Innova- tion DRIMS (TIN-2009-14247-C02-01). The research stay at the MPIPKS was funded by the German Academic Exchange Service (DAAD; A/09/96235) and the MPIPKS. Abstract Automatically making sense of digital information, and specially of music dig- ital documents, is an important problem our modern society is facing. In fact, there are still many tasks that, although being easily performed by humans, cannot be effectively performed by a computer. In this work we focus on one of such tasks: the identification of musical piece versions (alternate renditions of the same musical composition like cover songs, live recordings, remixes, etc.). In particular, we adopt a computational approach solely based on the information provided by the audio signal. We propose a system for version identification that is robust to the main musical changes between versions, including timbre, tempo, key and structure changes. Such a system exploits nonlinear time series analysis tools and standard methods for quantitative mu- sic description, and it does not make use of a specific modeling strategy for data extracted from audio, i.e. it is a model-free system. We report remarkable accuracies for this system, both with our data and through an international evaluation framework. Indeed, according to this framework, our model-free approach achieves the highest accuracy among current version identification systems (up to the moment of writing this thesis). Model-based approaches are also investigated. For that we consider a number of linear and nonlinear time series models. We show that, although model-based approaches do not reach the highest accuracies, they present a number of advantages, specially with regard to computational complexity and parameter setting. In addition, we explore post-processing strategies for version identification systems, and show how unsupervised grouping algorithms allow the characterization and enhancement of the output of query-by-example systems such as the version identification ones. To this end, we build and study a complex network of versions and apply clustering and community detection algorithms. Overall, our work brings automatic version identification to an unprecedented stage where high accuracies are achieved and, at the same time, explores promising directions for future research. Although our steps are guided by the nature of the considered signals (music recordings) and the characteristics of the task at hand (version identification), we believe our methodology can be easily trans- ferred to other contexts and domains. ix Resum Racionalitzar o donar significat de manera automàtica a la informació digital, especialment als documents digitals de música, és un problema important que la nostra societat moderna està afrontant. De fet, encara hi ha moltes tasques que, malgrat els humans les puguem fer fàcilment, encara no poden ser rea- litzades per un ordinador. En aquest treball ens centrem en una d’aquestes tasques: la identificació de versions musicals (interpretacions alternatives d’u- na mateixa composició de música tals com ‘covers’, enregistraments en directe, remixos, etc.). Basant-nos en un enfocamen computacional, i utilitzant única- ment la informació que ens proporciona el senyal d’àudio, proposem un sistema per a la identificació de versions que és robust als principals canvis musicals que hi pot haver entre elles, incloent canvis en el timbre, el tempo, la tonalitat o l’estructura del tema. Aquest sistema explota eines per a l’anàlisi no linial de sèries temporals i mètodes estàndard per a la descripció quantitativa de la música. A més a més, no utilitza cap estratègia de modelat de les dades extretes de l’àudio; és un sistema ‘lliure de model’. Amb aquest sistema obte- nim molt bons resultats, tant amb les nostres dades com a través d’un entorn d’avaluació internacional. De fet, d’acord amb aquestes últimes avaluacions, el nostre sistema lliure de model obté a dia d’avui els millors resultats d’entre tots els sistemes avaluats. També investiguem sistemes basats en models. A tal efecte, considerem un seguit de models de sèries temporals, tant linials com no linials.
Details
-
File Typepdf
-
Upload Time-
-
Content LanguagesEnglish
-
Upload UserAnonymous/Not logged-in
-
File Pages185 Page
-
File Size-