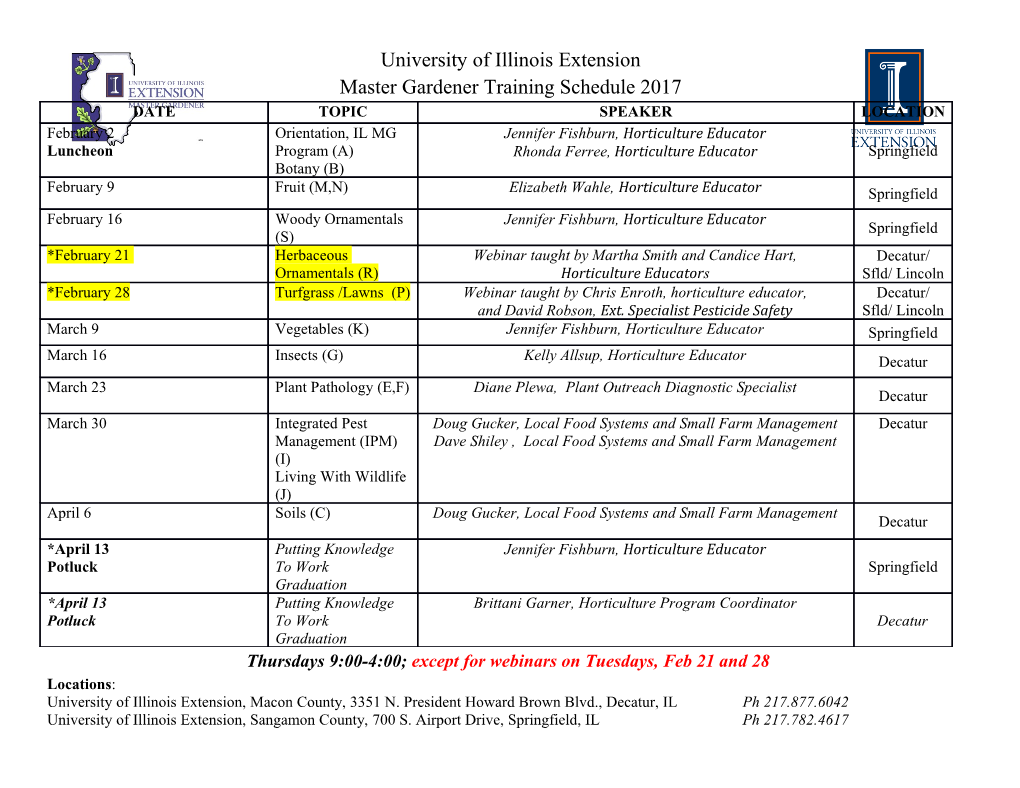
Prediction of Electron Cutout Factors Using Residual Neural Network by Chuan He Graduate Program in Medical Physics Duke University Date:_______________________ Approved: ___________________________ Qiuwen Wu, Supervisor ___________________________ Jun Lian ___________________________ Shiva Das ___________________________ Yvonne Mowery Thesis submitted in partial fulfillment of the requirements for the degree of Master of Science in the Graduate Program in Medical Physics in the Graduate School of Duke University 2019 iv ABSTRACT Prediction of Electron Cutout Factors Using Residual Neural Network by Chuan He Graduate Program in Medical Physics Duke University Date:_______________________ Approved: ___________________________ Qiuwen Wu, Supervisor ___________________________ Jun Lian ___________________________ Shiva Das ___________________________ Yvonne Mowery An abstract of a thesis submitted in partial fulfillment of the requirements for the degree of Master of Science in the Graduate Program in Medical Physics in the Graduate School of Duke University 2019 iv Copyright by Chuan He 2019 Abstract Background: Monte Carlo and square root are two commonly used calculation methods to predict electron cutout factors in clinic. Monte Carlo is accurate but time consuming, while square root is faster but less accurate for cutouts with highly irregular shapes. Purpose: Simplify and develop an efficient residual neural network model to predict electron cutout factors accurately and instantly. Methods: 281 clinical cutouts were screened, and 12 groups were designed combining four different electron energies (6, 9, 12 and 15 MeV) and three different cones (10, 14 and 20 cm). The cutout factors of 35 previously used electron cutouts were calculated by Monte Carlo simulation and also measured with a solid water phantom and an ion chamber for validation of Monte Carlo accuracy. To solve the issue of sparse training data, 600 cutout samples were created for each group based on modifications of previously screened clinical cutouts. Cutout factors of these 600 samples were calculated with Monte Carlo simulation. 400 samples were randomly selected as training data, 50 as validating and the remaining 150 as testing. 1D Distance histograms were calculated as model input instead of 2D images to accelerate the training process. 1D Residual neural network with four residual blocks and three linear blocks was used. Performance of the trained model was evaluated with testing data, and the accuracy of the model was iv compared with square root method for eight selected cutouts with highly irregular shapes. Results: The Monte Carlo calculated cutout factor agreed with the measurement within 0.7±0.5% on average. During the training process, the model started to converge within 20 epochs with 30 seconds. For model prediction, mean errors and maximum discrepancies for each energy and cone combinations were all within 1%, and the average overall error was 0.2±0.16%. Compared to square root method, the trained model performed better for cutouts with highly irregular shapes. Conclusion: An efficient residual neural network model was simplified and developed, which is capable of estimating electron cutout factors accurately and instantly. v Contents Abstract .......................................................................................................................................... iv List of Tables ................................................................................................................................. ix List of Figures ................................................................................................................................ x Acknowledgements .................................................................................................................... xii 1. Introduction ............................................................................................................................... 1 1.1 Electron beam radiation therapy .................................................................................... 2 1.1.1 Ionizing radiation ........................................................................................................ 2 1.1.2 External beam radiation therapy ............................................................................... 3 1.1.3 Dose calculation for electron beams ......................................................................... 5 1.1.4 Prediction of electron output factors in the literature ............................................ 7 1.1.4.1 Equivalent square and square root .................................................................... 8 1.1.4.2 Sector integration and CMCI ............................................................................ 10 1.1.4.3 Pencil beam ......................................................................................................... 11 1.1.4.4 Monte Carlo Simulation .................................................................................... 12 1.2 Deep learning .................................................................................................................. 13 1.2.1 Regression and classification ................................................................................... 14 1.2.2 Overfitting .................................................................................................................. 15 1.2.3 Convolutional neural network ................................................................................ 17 1.2.4 Residual neural network .......................................................................................... 19 1.2.5 Application of deep learning in medicine ............................................................. 20 vi 1.3 Summary .......................................................................................................................... 22 1.4 Project Objective ............................................................................................................. 23 2. Methods .................................................................................................................................... 24 2.1 Preparations of cutouts and cutout factors ................................................................. 24 2.1.1 Group design .............................................................................................................. 24 2.1.2 Create plans for each case in RayStation ................................................................ 27 2.1.3 Calculation of cutout factors using Monte Carlo method ................................... 30 2.2 Model development ....................................................................................................... 31 2.2.1 Distance histogram for cutouts ............................................................................... 31 2.2.2 ResNet model structure ............................................................................................ 33 2.3 Model training ................................................................................................................ 36 2.4 Model performance evaluation .................................................................................... 38 3. Results ....................................................................................................................................... 39 3.1 Monte Carlo accuracy .................................................................................................... 39 3.2 Accuracy of trained models .......................................................................................... 40 3.3 Comparisons of square root and ResNet model prediction accuracy .................... 45 4. Discussion ................................................................................................................................ 47 4.1 Is Monte Carlo simulation accurate enough? ............................................................. 47 4.2 Advantages and disadvantages of using distance histogram .................................. 47 4.3 Model performance ........................................................................................................ 49 4.4 Is deep learning a better choice compared to square root? ...................................... 50 4.5 Future work ..................................................................................................................... 51 vii 5. Conclusion ................................................................................................................................ 53 References ..................................................................................................................................... 54 viii List of Tables Table 1: Screened clinical cases for electron beam radiation therapy ................................. 25 Table 2: Summary of group design .......................................................................................... 27 Table 3: Hyperparameters used for model training. .............................................................. 37 Table 4: Measured (MS) and calculated (MC) cutout factors for eight clinical cutouts. ... 40 Table 5: Prediction errors for trained models. ........................................................................ 41 Table 6: Errors for cutout factor estimation using square root and ResNet model. (MC for Monte Carlo simulation, ML for model prediction, and
Details
-
File Typepdf
-
Upload Time-
-
Content LanguagesEnglish
-
Upload UserAnonymous/Not logged-in
-
File Pages71 Page
-
File Size-