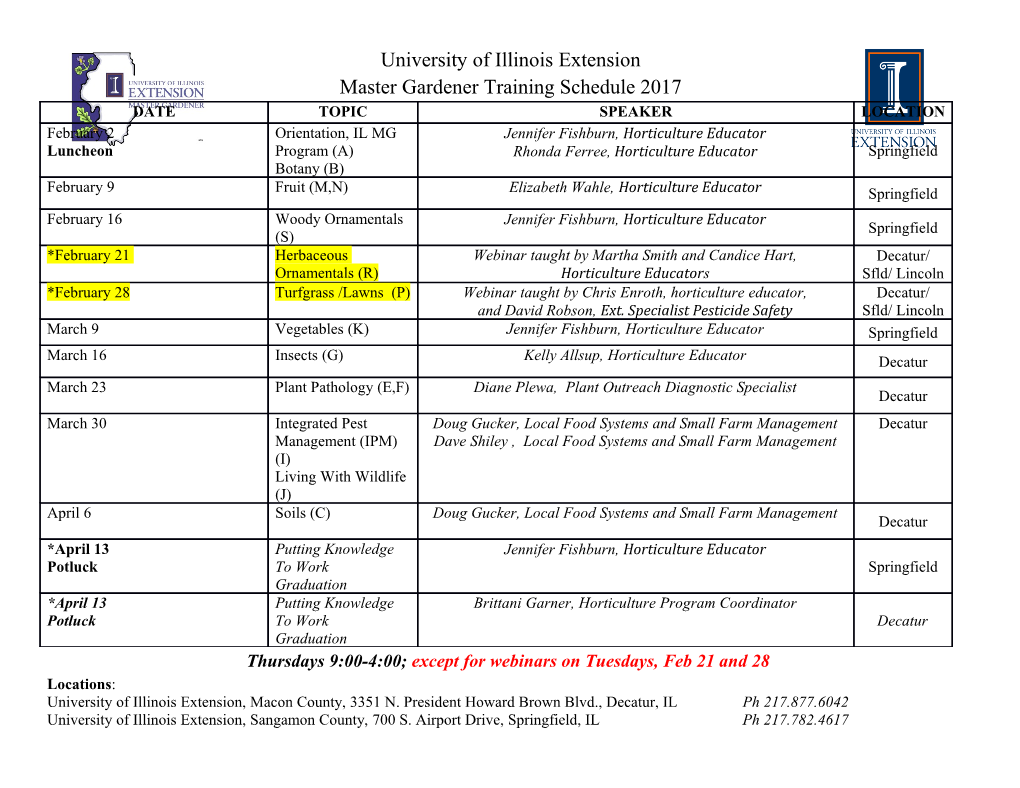
The Kelly Growth Criterion Niels Wesselhöt Wolfgang K. Härdle International Research Training Group 1792 Ladislaus von Bortkiewicz Chair of Statistics HumboldtUniversität zu Berlin http://irtg1792.hu-berlin.de http://lvb.wiwi.hu-berlin.de Motivation 1-1 Portfolio choice Playing Blackjack Figure 1: 'Ed' Thorp Figure 2: Matlab GUI Motivation 1-2 Portfolio choice Wealth for discrete returns k Xi 2 R n 0 k 1 Y X Wn(f ) = W0 @1 + fj Xj;i A (1) i=1 j=1 + I W0 2 R starting wealth + I k 2 N assets with index j + I n 2 N periods with index i k How to chose fraction vector f 2 R ? Motivation 1-3 Managing Portfolio Risks Two main strands 1. Mean-Variance approach: Markowitz (1952), Tobin (1958), Sharpe (1964) and Lintner (1965) 2. Kelly growth-optimum approach: Kelly (1956), Breiman (1961) and Thorp (1971) Leo Breiman on BBI: Outline 1. Motivation X 2. Bernoulli - Kelly (1956) 3. Gaussian - Thorp (2006) 4. General i.i.d. - Breiman (1961) 5. Appendix Bernoulli - Kelly (1956) 2-1 Arithmetic mean maximization Consider n favorable Bernoulli games with probability 1 2 < p ≤ 1 (q = 1 − p) and outcome X = 1 (−1) For P(X = 1) = p = 1, investor bets everything, f = 1 n Wn = W02 (2) Uncertainty - maximizing the expectation of wealth implies f = 1 n X E(Wn) = W0 + (p − q) E (fWn−1) ; (3) i=1 Leads to ruin asymptotically n n o P (fWn ≤ 0g) = P lim (1 − p ) ! 1 (4) n!1 Bernoulli - Kelly (1956) 2-2 Minimizing risk of ruin Alternative: minimize the probability of ruin For f = 0 P (fWn ≤ 0g) = 0 (5) Minimum ruin strategy leads also to the minimization of the expected prots as no investment takes place Bernoulli - Kelly (1956) 2-3 Geometric mean maximization Gambler bets a fraction of his wealth with m games won m n−m Wn = W0(1 + f ) (1 − f ) (6) Exponential rate of growth per trial (log of the geometric mean) 1 Wn n n m n−m o Gn(f ) = log = log (1 + f ) n (1 − f ) n (7) W0 m n − m = log(1 + f ) + log(1 − f ) (8) n n Bernoulli - Kelly (1956) 2-4 Geometric mean maximization By Borel's law of large numbers E fGn(f )g = g(f ) = p · log(1 + f ) + q · log(1 − f ) (9) Maximizing g(f ) w.r.t. f : p q p − q − f g 0(f ) = − = = 0 1 + f 1 − f (1 + f )(1 − f ) (10) ≺ f = f ∗ = p − q; p ≥ q > 0 (11) Second derivative according to f p q g 00(f ) = − − < 0 (12) (1 + f )2 (1 − f )2 Bernoulli - Kelly (1956) 2-5 Closed form for Bernoulli trials Growth optimal fraction, under Bernoulli trials: f ∗ = p − q (13) Maximizes the expected value of the logarithm of capital at each trial g(f ∗) = p · log(1 + p − q) + q · log(1 − p − q) (14) = p · log(p) + q · log(q) + log(2) > 0 (15) A link to information theory Bernoulli - Kelly (1956) 2-6 Bernoulli example, p = 0:6 Exponential rate of asset growth for binary channel with p=0.6 0 0.02 -0.5 0.015 0.01 -1 0.005 -1.5 0 0 0.5 1 0 0.2 f f Figure 3: Bernoulli Exponential growth rate g(f) Bernoulli - Kelly (1956) 2-7 Bernoulli 1 0 0.5 -1 -2 0 -3 -4 -0.5 1 1 0.5 0.5 -1 0 p 0 f Figure 4: Bernoulli - Exponential growth rate g(f,p) Gaussian - Thorp (2006) 3-1 Gaussian (One-dimensional) 2 X ∼ F with E(X ) = µ and Var(X ) = σ Return of the risk free asset r > 0 Wealth given investment fractions and restriction Pk 1 j=1 fj = W (f ) = W0 f1 + (1 − f )r + fX g (16) = W0 f1 + r + f (X − r)g (17) Gaussian - Thorp (2006) 3-2 Gaussian (One-dimensional) Maximize g(f ) = E flog Wn(f )g = E fG(f )g = E log fWn(f )=W0g (18) Wealth after n periods n Y Wn(f ) = W0 f1 + r + f (Xi − r)g (19) i=1 Taylor expansion of " n # Wn(f ) X E log = E log f1 + r + f (Xi − r)g (20) W0 i=1 Gaussian - Thorp (2006) 3-3 Gaussian (One-dimensional) Given log 1 x2 ( + x) = x − 2 + ··· 2 log 1 fr + f (X − r)g f + r + f (X − r)g = r + f (X − r) − 2 + ··· (21) 2 2 X f (22) ≈ r + f (X − r) − 2 Taking sum and expectation " n # 2 2 E X log 1 σnf f + r + f (Xi − r)g ≈ r + f (µn − r) − 2 i=1 (23) Myopia: taking Pn has no impact on the solution i=1 Xi Gaussian - Thorp (2006) 3-4 Gaussian (One-dimensional) Result of the Taylor expansion g(f ) = r + f (µ − r) − σ2f 2=2 + O(n−1=2): (24) −1=2 For n −! 1, O(n ) −! 0 2 2 g1(f ) = r + f (µ − r) − σ f =2: (25) Dierentiating g(f ) according to f @g (f ) µ − r 1 = µ − r − σ2f = 0 ≺ f ∗ = = σ−1MPR (26) @f σ2 ∗ Betting the optimal fraction f leads to growth rate (µ − r)2 g (f ∗) = + r: (27) 1 2σ2 is parabolic around ∗ with range 0 ∗ 2 ∗ g1(f ) f ≤ f ≤ f Gaussian - Thorp (2006) 3-5 Gaussian - µ = 0:03; σ = 0:15; r = 0:01 0.035 0.03 0.025 0.02 0.015 0.01 0.005 0 0 0.5 1 1.5 2 2.5 f Figure 5: Gaussian approximation - Exponential growth rate g(f) Gaussian - Thorp (2006) 3-6 Gaussian (Multi-dimensional) X ∼ N(µ, Σ) and risk free rate r > 0 n > o Wn(f ) = W0 1 + r + f (X − r) (28) Taking logarithm and expectations on both sides leads via Taylor series to 1 1 g(f ) = E log(1 + r) + (µ − 1r)>f − f >Σf 1 + r 2(1 + r)2 (29) From quadratic optimization (Härdle and Simar, 2015) f ∗ = Σ−1(µ − 1r) (30) ∗ ∗> ∗ g1(f ) = r + f Σf =2 (31) Gaussian - Thorp (2006) 3-7 Gaussian - µ = [0:03 0:08]; σ = [0:15 0:15]; ρ = 0; r = 0:01 0.14 0.12 0.15 0.1 0.1 0.08 0.05 0.06 0 0.04 3 3 2 0.02 2 1 1 0 f 0 0 f 2 1 Figure 6: Gaussian approximation - Exponential growth rate g(f) General i.i.d. - Breiman (1961) 4-1 General i.i.d. Asymptotic dominance (in terms of wealth) of the Kelly strategy in a general i.i.d. setting in discrete time Figure 7: Warren Buf- fett Figure 8: Matlab GUI General i.i.d. - Breiman (1961) 4-2 General i.i.d. 2 3 fi;j ··· fn;j . Investment strategy 6 . .. 7 Λ = 4 . 5 = [fi ··· fn] fi;k ··· fn;k investment fractions from time to + I fi i n 2 N + I opportunities j to k 2 N 2 3 pi;j . Security price vector 6 . 7 pi = 4 . 5 pi;k 2 pi;j 3 p 1 i−. ;j Return per unit invested 6 . 7. xi = 4 . 5 pi;k pi−1;k General i.i.d. - Breiman (1961) 4-3 Discrete i.i.d. setting Wealth of the investor in period n n o > (32) Wn(fn) = Wn−1(fn−1) fn xn increases exponentially Wn(fn) Log-optimal fraction through growth rate maximization at each trial ∗ f = argmax E flog(Wn)g (33) f 2Rk General i.i.d. - Breiman (1961) 4-4 Asymptotic outperformance Theorem ∗ ∗ ∗ Myopic log-optimal strategy Λ = [f ··· f ] Signicantly dierent strategy Λ ∗ E flog Wn(Λ )g − E flog Wn(Λ)g −! 1; (34) Kelly investor dominates asymptotically W (Λ∗) lim n −!a:s: 1 (35) n!1 Wn(Λ) Leo Breiman on BBI: General i.i.d. - Breiman (1961) 4-5 Minimize time to reach goal g Theorem Let be the smallest , such that 0 N(g) n Wi ≥ g; g > If equation (34) holds, 0 (36) 9α ≥ = Λ; g j such that E fN∗(g)g − E fN(g)g ≤ α; (37) - independent of = j ∗ Λ asymptotically minimizes the time to reach goal g General i.i.d. - Breiman (1961) 4-6 Time invariance Theorem Given a xed set of opportunities the strategy is I xed fraction I independent of the number of trials n ∗ ∗ ∗ ∗ ∗ (38) Λ = [f1 ··· fn ]; f1 = ··· = fn General i.i.d. - Breiman (1961) 4-7 Bernoulli revisited Theorem Two investors with equal initial endowment, investment fractions f1 and f2 For exponential growth rates Gn(f1) > Gn(f2) (39) the Kelly bet dominates asymptotically W (f1) lim n −!a:s: 1 (40) n!1 Wn(f2) General i.i.d. - Breiman (1961) 4-8 Bernoulli revisited Proof. n o 1 Dierence in exponential growth rates G (f ) = log Wn(f ) n n W0 1 1 1 W (f1) n W (f2) n W (f1) n log n − log n = log n (41) W0 W0 Wn(f2) by Borel strong law of large numbers " 1 # W (f1) n P lim log n > 0 −!a:s: 1: (42) n!1 Wn(f2) General i.i.d. - Breiman (1961) 4-9 Bernoulli revisited Proof. For ! 2 Ω, there exists N(!) such that for n ≥ N(!), W0 exp fnG(f1)g > W0 exp fnG(f2)g (43) Wn(f1) > Wn(f2) (44) Asymptotically W (f1) lim n −!a:s: 1 (45) n!1 Wn(f2) Utility functions - Thorp (1971) 5-1 Utility functions Three types of utility theories: Thorp (1971) I Descriptive utility - empirical data and mathematical tting I Predictive utility - derives utility functions out of hypotheses I Normative utility - describe the behavior to achieve a certain goal The logarithmic utility function is used in a normative way Utility functions - Thorp (1971) 5-2 Conclusion Comparison of risk management theories I Markowitz-approach • arithmetic mean-variance ecient • maximizing single period returns • rests on two moments I Kelly-approach • geometric mean-variance ecient • maximize geometric rate of multi-period returns • utilizes the whole distribution Information theory 6-1 Information Closed form for Bernoulli trials Self-information (uncertainty) of outcome x 1 i(x) = − log P(x) = log (46) P(x) i(x) = 0; for P(x) = 1 (47) i(x) > 1; for P(x) < 1 (48) Example: For a fair coin, the change of P (x = ftailg) = 0:5 i(x) = − log2(1=0:5) = 1 bit Information theory 6-2 Information 10 8 6 4 Information in bit 2 0 0 0.2 0.4 0.6 0.8 1 Probability p Figure 9: Self information of an outcome given probability p Information theory 6-3 Entropy Entropy as expectation of self-informations (average uncertainty), given outcomes X = fX1;:::; Xng H(X ) = E fI (X )g = − E flog P(X )g (49) X = − P(x) log2 P(x) ≥ 0 (50) x For two outcomes and p = q = 0:5 H(X ) = − (p log2 p + q log2 q) = − (1=2 log2 1=2 + 1=2 log2 1=2) = 1 bit Information theory 6-4 Entropy 1 0.8 0.6 0.4 Entropy H(X) 0.2 0 0 0.2 0.4 0.6 0.8 1 Probability p Figure 10: Entropy for two outcomes given probability p (1-p) Information theory 6-5 Entropy Joint entropy H(X ; Y ) = − E flog P(X ; Y )g (51) X = − P(x; y) log P(x; y) (52) x;y Conditional entropy H(X j Y ) = − E flog P(X j Y )g (53) X = − P(x j y) log P(x j y) (54) x;y Information theory 6-6 Noisy binary channel Figure 11: Noisy binary channel Information theory 6-7 Mutual information Mutual information I (X ; Y ) = H(X ) −
Details
-
File Typepdf
-
Upload Time-
-
Content LanguagesEnglish
-
Upload UserAnonymous/Not logged-in
-
File Pages44 Page
-
File Size-