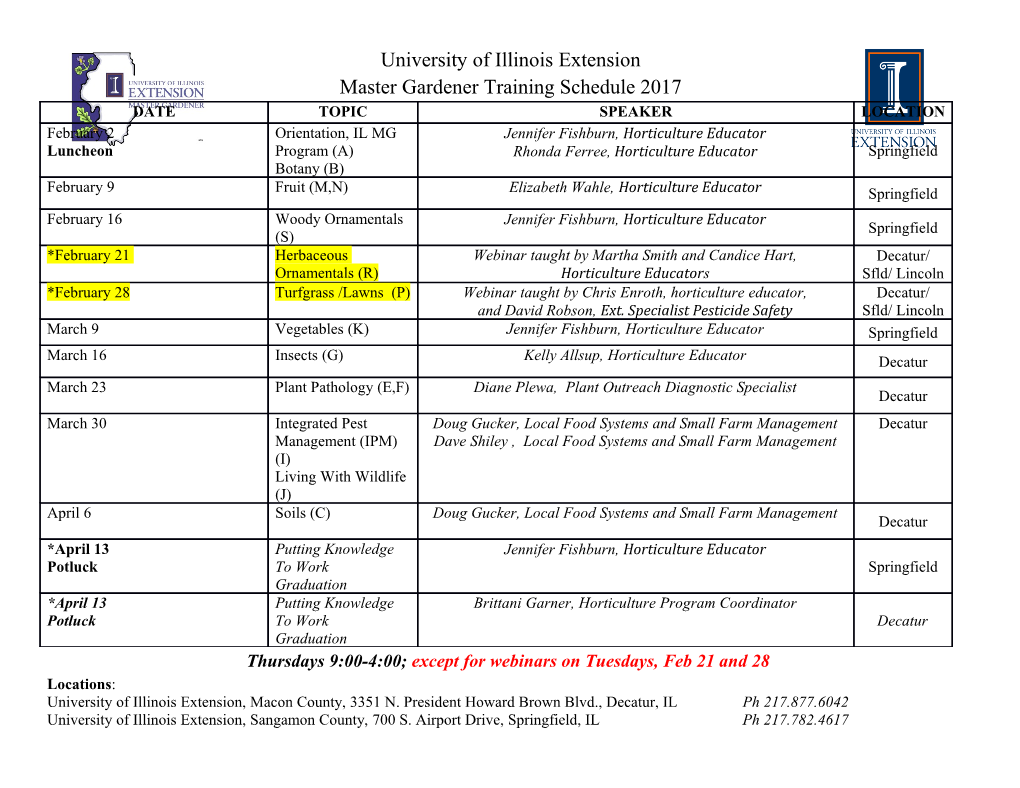
Noname manuscript No. (will be inserted by the editor) Modeling return to education in heterogeneous populations Angelo Mazza · Michele Battisti · Salvatore Ingrassia · Antonio Punzo the date of receipt and acceptance should be inserted later Keywords Indicators of return to education · Mincer’s earnings function · mixtures of regression models JEL classification: C14, J24, J31 Abstract The Mincer human capital earnings function is a regression model that relates individual’s earnings to schooling and experience. It has been used to explain individual behavior with respect to educational choices and to in- dicate productivity on a large number of countries and across many different demographic groups. However, recent empirical studies have shown that often the populations of interest embed latent homogeneous subpopulations, with different returns to education across subpopulations, rendering a single Min- cer’s regression inadequate. Moreover, whatever (concomitant) information is available about the nature of such a heterogeneity, it should be incorporated in an appropriate manner. We propose a mixture of Mincer’s models with con- comitant variables: it provides a flexible generalization of the Mincer model, a breakdown of the population into several homogeneous subpopulations, and an explanation of the unobserved heterogeneity. The proposal is motivated and illustrated via an application to data provided by the Bank of Italy’s Survey of Household Income and Wealth in 2012. 1 Introduction Earnings functions are used by social scientists to explain individual behavior with respect to educational choices and to indicate productivity (Oreopoulos M. Battisti Dipartimento di Scienze Giuridiche, della Societ`ae dello Sport, Universit`adi Palermo, Via Maqueda, 172, 90134 Palermo, Italy, E-mail: [email protected] S. Ingrassia, A. Mazza and A. Punzo Dipartimento di Economia e Impresa, Universit`adi Catania, Corso Italia, 55, 95129 Catania, Italy, E-mail: [email protected], E-mail: [email protected], E-mail: [email protected] 2 Short form of author list and Petronijevic, 2013). They provide an indicator of returns to schooling, typ- ically in the form of projected future wages, which helps individuals to decide how to invest in their own human capital (Patrinos, 2016). These indicators are also used as a basis for setting public policy with respect to investment in education (Davis and Noland, 2003). Introduced by Jacob Mincer, a pioneer of the New Labor Economics, in his seminal work Schooling, Experience and Earnings, the“human capital earn- ings function” is arguably the most popular earning function (Mincer, 1974; Chiswick, 2007; Machin, 2007). It is a single-equation model that explains the natural logarithm of earnings as a linear function of years of education, years of potential labor market experience, and the square of years of potential experience; in formula, 2 ln(y)= µ (x; β)+ ε = β0 + β1x1 + β2x2 + β3x2 + ε, (1) where y denotes earnings, x1 and x2 represent years of education and years of potential labor market experience respectively1, while ε ∼ N (0,σ), with σ ′ being the (conditional) standard deviation of ln (y). In (1), x =(x1,x2) and ′ β =(β0,β1,β2,β3) . The Mincer equation owes its popularity to the straightforward interpre- tation of the coefficient β1 as approximated rate of return to education (see Bj¨orklund and Kjellstr¨om, 2002, for a critical discussion). It has been examined on many datasets, involving a large number of countries and many different demographic groups and, as stated by Lemieux (2006), it is “one of the most widely used models in empirical economics”. Within income inequality stud- ies, it has been used to study wage differentials due to gender (Smith and Westergard-Nielsen, 1988) and for predicting the wage that a self employed worker in a certain sector of the economy would have received on average as a paid employee on the same sector of economy (Atkinson, 1986, p. 163; Amarante, 2014). The literature on educational mismatches uses several dif- ferent specifications of equation (1) for quantifying the effect of educational mismatch on wages (Nieto and Ramos, 2016). However, recent empirical studies (see, e.g., Nordin, 2008, Henderson et al, 2011, Kopf et al, 2013 and Battisti, 2013) have shown the relevance of unob- served heterogeneity. That is, often the populations of interest are constituted by latent groups bearing different returns to educations and characterized by different socio-demographic profiles, in a way that regression coefficients (and dispersion parameters) cannot be assumed to be the same for all observations, making the use of a single Mincer’s regression inadequate. Heterogeneity and segmentation has been shown for Italy by Cipollone, 2001 and by Battisti, 2013. In fact, the Italian labor market has been traditionally characterized by rigid institutions, with employment protection legislation imposing strict rules and constraints regarding the ability to hire and fire workers. Reforms that started at the end of the ’90s have increasingly introduced more flexibility, but these new rules mostly apply only to newly hired workers; this has lead to the 1See Card (1999, 2001) for a discussion on the use of polynomial terms for experience. Modeling return to education in heterogeneous populations 3 formation of a two-tier labor market (see for instance Boeri and Garibaldi, 2007). Finite mixtures of linear regression models, introduced by Quandt and Ramsey (1978) in the general form of “switching regression”, constitute a ref- erence framework of analysis when no information about group membership is available and the modeling aim is to find groups of observations with sim- ilar regression coefficients (Gr¨un and Leisch, 2008a). Furthermore, whatever (concomitant) socio-demographic information is available about the nature of such a heterogeneity, it should be incorporated in the model in an appropriate manner. To deal with these issues, based on Dayton and Macready (1988), in Section 2 we introduce a finite mixture of Mincer’s regression models with concomitant variables: the proposed model simultaneously provides a flexi- ble generalization of the Mincer regression, a breakdown of the population into several homogeneous subpopulations, and an explanation of the unob- served heterogeneity also based on the considered concomitant variables. The expectation-maximization (EM) algorithm is used for parameter estimation and the Bayesian information criterion (BIC) is adopted to select the number of groups (or clusters or mixture components). In Section 3, the model is applied to disposable household income, as obtained from the Bank of Italy’s Survey of Household Income and Wealth (SHIW) in 2012. In addition to illustrate the use of the model, this real data application demonstrates, based on the BIC, how a single Mincer’s regression is inadequate for the data at hand. 2 The model Given a d-dimensional vector W of concomitant variables (individual charac- teristics), and based on Dayton and Macready (1988), we propose to generalize equation (1) via a finite mixture of k Mincer’s regressions with concomitant variables; being a mixture, the proposed model can be defined from the con- ditional density of ln (y), given x and w, in the following way k p [ln(y) |x, w; ϑ]= πj (w; α) φ ln(y) |x; µ x; β ,σj , (2) X j j=1 where πj (w; α), j = 1,...,k, are positive weights (depending on the pa- rameters α) summing to one for each w, φ (·; µ,σ) denotes the density of a Gaussian random variable with mean µ and standard deviation σ, µ x; β , j j = 1,...,k, is defined as in (1), and ϑ contains all of the parameters of the model. The multinomial logit model ′ exp(αj0 + α w) π (w; α)= j1 (3) j k ′ exp(α + α w) X h0 h1 h=1 4 Short form of author list ′ is assumed for the mixture weights in (2), where αj1 = (αj1,...,αjd) , αj = ′ ′ d+1 ′ ′ ′ αj , α ∈ IR , and α = (α ,..., α ) , with α ≡ 0 for identifiability 0 j1 1 k 1 sake (see Gr¨un and Leisch, 2008b, p. 4). Being a mixture, model (2) may serve two different purposes (Titterington et al, 1985, pp. 2–3). First, it can be used as a semi-parametric competitor of nonparametric estimation techniques for the conditional density of ln(y) since, under regularity conditions, any density can be consistently estimated by a mixture of normal densities (Ghosal and van der Vaart, 2001). Second, and more importantly, model (2) can be used as a powerful device for clustering by assuming that each mixture component represents a group underlying the overall population (McLachlan and Basford, 1988). Advantageously, by means of the mixture weights in (3), the concomitant variables w can be used to explain the profiles of the different groups. Here, it is important to stress that, based on the mixture model (2), we do not specify the groups a priori, but we let the data identify homogeneous groups with respect to the relationship between ln(y) and x. 2.1 Maximum likelihood estimation: the EM algorithm To find maximum likelihood (ML) estimates for ϑ in (2), we adopt the EM al- gorithm of Dempster et al (1977), as implemented by the stepFlexmix() func- tion of the flexmix package (Leisch, 2004; Gr¨un and Leisch, 2008b) for R (R ′ ′ ′ ′ ′ ′ Core Team, 2013). In detail, given a random sample (y1, x1, w1) ,..., (yn, xn, wn) of (Y, X, W ) from model (2), and once k is assigned, the algorithm basically takes into account the complete-data log-likelihood n k n k lc (ϑ)= zij ln[πj (wi; α)] + zij ln p yi|xi; β ,σj , (4) X X X X j i=1 j=1 i=1 j=1 ′ ′ ′ where zij =1if(yi, xi, wi) comes from component j and zij = 0 otherwise. The EM algorithm iterates between two steps, one E-step and one M-step, until convergence; their schematization, with respect to model (2), is given below (see Wedel and DeSarbo, 1995 and Wedel and Kamakura, 2000, pp.
Details
-
File Typepdf
-
Upload Time-
-
Content LanguagesEnglish
-
Upload UserAnonymous/Not logged-in
-
File Pages11 Page
-
File Size-