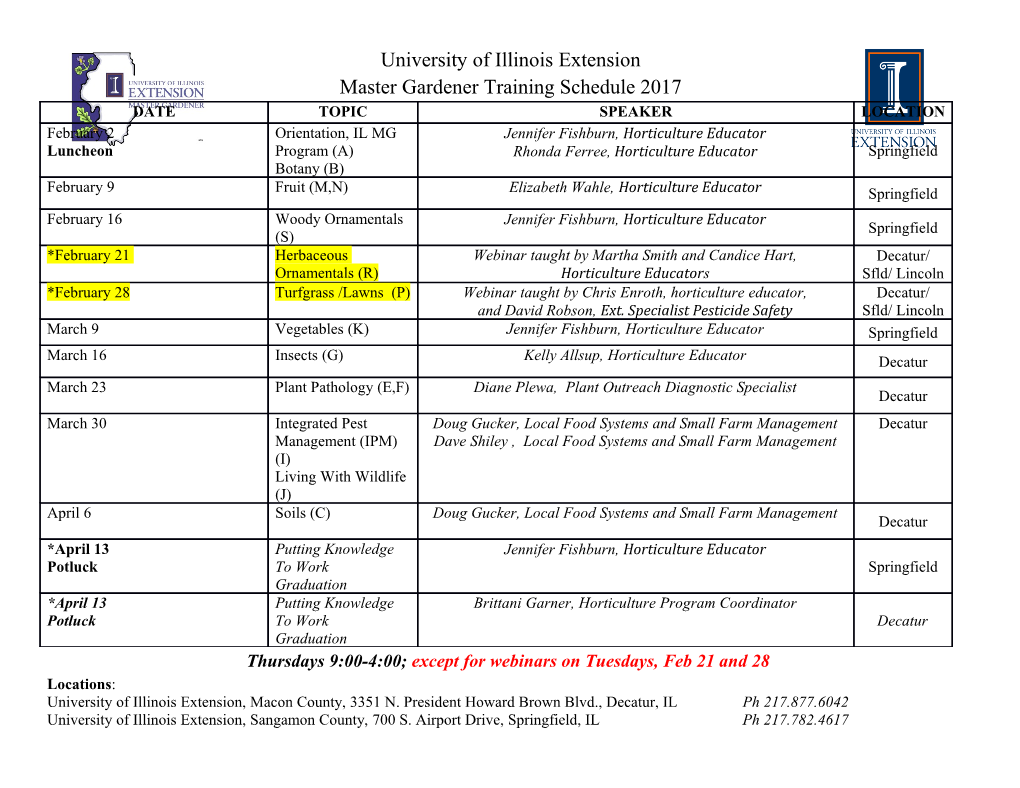
Insight from other regions: Development of air quality forecasts for winter-time PM2.5 episodes occurring on multiple cities in south-central Chile Pablo Saide Department of Atmospheric & Oceanic Science, Institute of the Environment and Sustainability, UCLA Co-authors: Marcelo Mena-Carrasco, Sebastian Tolvett, Pablo Hernandez (Chilean Ministry of the Environment) and Gregory R. Carmichael (U of Iowa) Trivia: What city is this and how big is it? 800 500 Hourly [µg/m3]PM2.5 Hourly City: Osorno. Population: ~150k inhabitants Severe PM2.5 episodes in south-central Chile • Produced by a combination of: – Complex topography – Episodic meteorological conditions – Emissions due to anthropogenic activities • Episodes are declared to warn the public and to try to reduce the impact by invoking temporary measures Santiago during en episode Wood burning stoves in Temuco 3 South America Forecasting system and SE Pacific • WRF-Chem model at high spatial resolution to resolve met conditions, topography and emissions et al., JGR 2016 Saide 4 24 hour mean PM2.5 vs CO PM2.5 modeling • CO and PM are highly correlated during episodes • Use CO tagged tracers (“traffic” and “wood burning stoves”) and an empirically calibration for 2014 • The conversion factors are chosen to match observed episode statistics. • Factors are introduced to include physical processes including % contribution of stoves by city, weekend effect, and temperature dependence Saide et al., Atmos Env 2011 Saide et al., JGR 2016 5 Episode’s Temperature Temperature histograms (Temuco) dependence • Episodes associated to colder temperatures (statistically significant) • This is due to emissions from wood burning stoves for heating • Including temperature correction helps reproduce histograms Factor Temperature [C] 6 WRF-Chem configuration was tuned to resolve episodes • No vertical diffusion thresholds for Chem • No horizontal diffusion on the inner domain • Sensitivity analysis was done for selecting vertical resolution (first two layers 10m thick, 6 levels below 100m), PBLH scheme (MYNN) and Meteorological Boundary conditions (GFS) Saide et al., Atmos Env 2011 Episode evolution (Osorno) 8 Results Temuco (Padre de las Casas II) PM2.5 24h mean • Large variability within forecasts due to different meteorological initialization. Ensemble forecasting should be explored in the future • Overall skill of 53–72% of episodes accurately forecasted which is generally better than persistence. 9 Saide et al., JGR 2016 Emission influence on different days • Episodes are 1 hour PM10 declared to reduce emissions to avoid bad air quality 24 hr average PM10 • 48 forecasts needed to declare episodes in advance Saide et al., Atmos Env 2011 10 Forecasting for 2015 • System in place since 2015 pollution season • Provided good guidance with similar skill as the 2014 calibration Emergency in Santiago Emergency in Osorno 11 How would a forecasting system for AQUARIUS could look like? • Two (or more?) nested domains to capture each sampling region • Similar configuration as Chilean system to predict episode occurrence 2 km • Ensemble forecasts to get a probabilistic 10 km forecast of episode occurrence • Post-campaign simulations would be 2 km performed with full chemistry for analysis 12 Science questions • Can a forecasting system developed for other regions predict winter-time air quality episodes in the western US? • Does an ensemble system improve predictability? • Once the accumulation of pollutants is properly modeled, can air quality models predict the chemical evolution during episodes? • Can data assimilation of atmospheric constituents (AOD, CO, NO₂) help in the predictability of these events? Does the addition of geostationary satellites data play a role? Previous work on data assimilation/inverse modeling: Saide et al., PNAS 2012; Saide et al., GRL 2014; Saide et al., GRL 2015a,b; Saide et al., JGR 2016; Saide et al., ES&T 2018; 13 Questions or comments? [email protected] Supplemental slides 15 24 hour mean PM2.5 vs CO PM2.5 modeling • CO and PM are highly correlated during episodes • Use CO tagged tracers (“traffic” and “wood burning stoves”) and an empirically calibration for 2014 • The conversion factors are chosen to match observed episode statistics. • Factors are introduced to include physical processes Weekend effect • There were 137%, 34% and 38% more episodes on average for weekend days than for weeks-days in Santiago, Rancagua and Talca, respectively. • Activity increases in Friday and Saturday nights, which generates episodes on Saturday and Sundays • Inflation factors: – 1.4 for Santiago – 1.2 for Rancagua – 1.3 for Talca and Chillán • Improvement in model performance: – 67% to 72% in Santiago – 64% to 69% in Rancagua – 57% to 63% in Talca – 63% to 67% in Chillan 17 Results Temuco (Padre de las Casas II) • Large variability within forecasts due Temperature 24h mean to different meteorological initialization • Variability in PM2.5 much larger than T and wind speed Wind speed 24h mean • Ensemble forecasting should be explored in the future PM2.5 • Overall skill of 53– 24h mean 72% of episodes accurately forecasted (61–76% for the best initialization) which is generally better than persistence. 18 Saide et al., JGR 2016 Wind evaluation OBS MODEL OBS MODEL OBS MODEL19 Precipitation Temuco Centro 20 Forecast skill • Forecasts one, two and three days in advance all have skill in forecasting • Latest forecast no necessarily the best • Forecast generally beat persistence 21 Episode evolution (Temuco) 22 Saide et al., JGR 2016.
Details
-
File Typepdf
-
Upload Time-
-
Content LanguagesEnglish
-
Upload UserAnonymous/Not logged-in
-
File Pages22 Page
-
File Size-