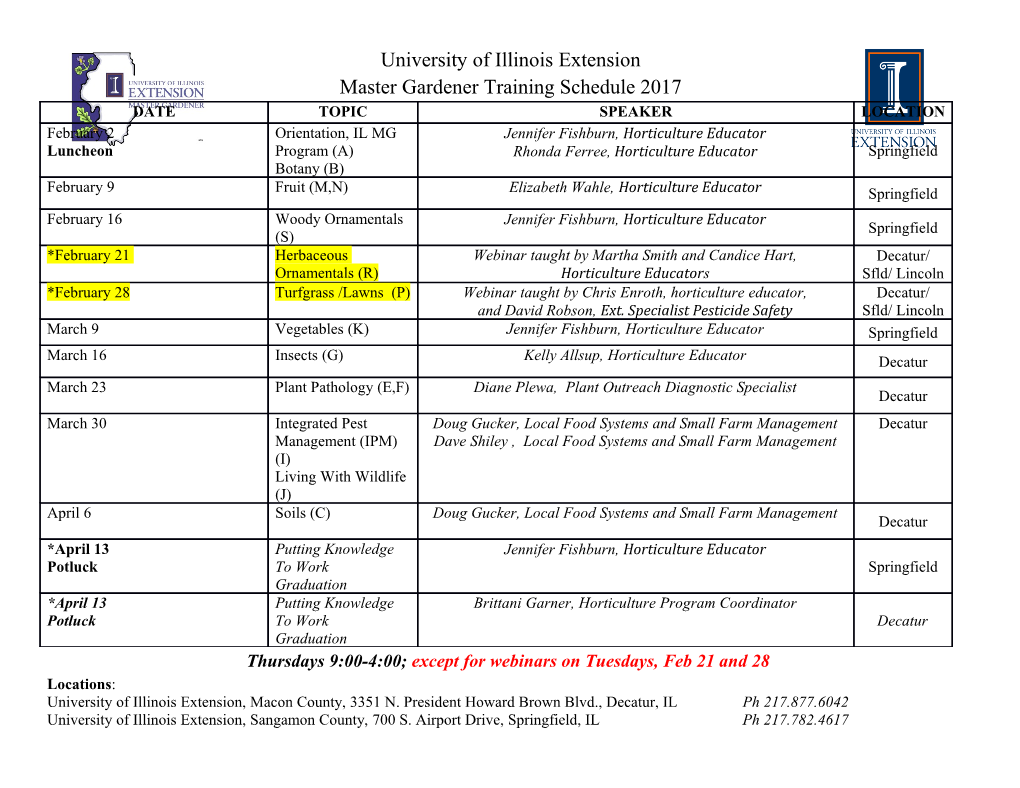
Need for Fuzzy . A Crisp \Exclusive Or" . Need for the Least . Least Sensitive For t-Norms and t- . Definition of a Fuzzy . (Most Robust) Main Result Interpretation of the . Fuzzy \Exclusive Or" Fuzzy \Exclusive Or" . Operations Home Page Title Page 1 2 Jesus E. Hernandez and Jaime Nava JJ II 1Department of Electrical and J I Computer Engineering 2Department of Computer Science Page1 of 13 University of Texas at El Paso Go Back El Paso, TX 79968 [email protected] Full Screen [email protected] Close Quit Need for Fuzzy . 1. Need for Fuzzy \Exclusive Or" Operations A Crisp \Exclusive Or" . Need for the Least . • One of the main objectives of fuzzy logic is to formalize For t-Norms and t- . commonsense and expert reasoning. Definition of a Fuzzy . • People use logical connectives like \and" and \or". Main Result • Commonsense \or" can mean both \inclusive or" and Interpretation of the . \exclusive or". Fuzzy \Exclusive Or" . Home Page • Example: A vending machine can produce either a coke or a diet coke, but not both. Title Page • In mathematics and computer science, \inclusive or" JJ II is the one most frequently used as a basic operation. J I • Fact: \Exclusive or" is also used in commonsense and Page2 of 13 expert reasoning. Go Back • Thus: There is a practical need for a fuzzy version. Full Screen • Comment: \exclusive or" is actively used in computer Close design and in quantum computing algorithms Quit Need for Fuzzy . 2. A Crisp \Exclusive Or" Operation: A Reminder A Crisp \Exclusive Or" . Need for the Least . • Fuzzy analogue of a classical logic operation op: For t-Norms and t- . { we know the experts' degree of belief a = d(A) and Definition of a Fuzzy . b = d(B) in statements A and B; Main Result { based on a and b, we want to estimate the degree Interpretation of the . of belief in \A op B", as fop(a; b). Fuzzy \Exclusive Or" . • For op = & , we get an \and"-operation (t-norm). Home Page • For op = _, we get an \or"-operation (t-conorm). Title Page • As usual, the fuzzy \exclusive or" operation must be JJ II an extension of the corresponding crisp operation ⊕. J I • In the traditional 2-valued logic, 0 ⊕ 0 = 1 ⊕ 1 = 0 and Page3 of 13 0 ⊕ 1 = 1 ⊕ 0 = 1. Go Back • Thus, the desired fuzzy \exclusive or" operation f⊕(a; b) Full Screen must satisfy the same properties: Close f⊕(0; 0) = f⊕(1; 1) = 0; f⊕(0; 1) = f⊕(1; 0) = 1: Quit Need for Fuzzy . 3. Need for the Least Sensitivity: Reminder A Crisp \Exclusive Or" . Need for the Least . • One of the main ways to elicit degree of certainty d is For t-Norms and t- . to ask to pick a value on a scale. Example: Definition of a Fuzzy . { on a scale of 0 to 10, an expert picks 8, so we get Main Result d = 8=10 = 0:8; Interpretation of the . { on a scale from 0 to 8, whatever we pick, we cannot Fuzzy \Exclusive Or" . Home Page get 0.8: 6=8 = 0:75 < 0:8; 7=8 = 0:875 > 0:8. { the expert will probably pick 6, with Title Page d0 = 6=8 = 0:75 ≈ 0:8: JJ II • It is desirable: that the result of the fuzzy operation J I not change much if we slightly change the inputs: Page4 of 13 0 0 0 0 jf(a; b) − f(a ; b )j ≤ k · max(ja − a j; jb − b j); Go Back with the smallest possible k. Full Screen • Such operations are called the least sensitive or the Close most robust. Quit Need for Fuzzy . 4. For t-Norms and t-Conorms, the Least Sensi- A Crisp \Exclusive Or" . tivity Requirement Leads to Reasonable Oper- Need for the Least . ations For t-Norms and t- . Definition of a Fuzzy . • Known results: Main Result { There is only one least sensitive t-norm (\and"- Interpretation of the . operation) Fuzzy \Exclusive Or" . f&(a; b) = min(a; b): Home Page { There is also only one least sensitive t-conorm (\or"- Title Page operation) JJ II f_(a; b) = max(a; b): J I • What we do in this presentation: we describe the least Page5 of 13 sensitive fuzzy \exclusive or" operation. Go Back Full Screen Close Quit Need for Fuzzy . 5. Definition of a Fuzzy Exclusive-Or Operation A Crisp \Exclusive Or" . Need for the Least . • Definition: A function f : [0; 1] × [0; 1] ! [0; 1] is For t-Norms and t- . called a fuzzy \exclusive or" operation if Definition of a Fuzzy . f(0; 0) = f(1; 1) = 0 and f(0; 1) = f(1; 0) = 1: Main Result Interpretation of the . • Comment: We could also require other conditions, e.g., Fuzzy \Exclusive Or" . commutativity and associativity. Home Page • However, our main objective is to select a single oper- Title Page ation which is the least sensitive. JJ II • Fact: The weaker the condition, the larger the class of J I operations that satisfy these conditions. Page6 of 13 • Thus: the stronger the result that our operation is the Go Back least sensitive in this class. Full Screen • Conclusion: We select the weakest possible condition to make our result as strong as possible. Close Quit Need for Fuzzy . 6. Main Result A Crisp \Exclusive Or" . Need for the Least . Definition: For t-Norms and t- . • Let F be a class of functions from [0; 1]×[0; 1] to [0; 1]. Definition of a Fuzzy . • We say that a function f 2 F is the least sensitive in Main Result the class F if it satisfies the following two conditions: Interpretation of the . Fuzzy \Exclusive Or" . { for some real number k, the function f satisfies the Home Page condition Title Page jf(a; b) − f(a0; b0)j ≤ k · max(ja − a0j; jb − b0j); JJ II { no other function f 2 F satisfies this condition. J I Theorem: In the class of all fuzzy \exclusive or" opera- Page7 of 13 tions, the following function is the least sensitive: Go Back f⊕(a; b) = min(max(a; b); max(1 − a; 1 − b)): Full Screen Close Quit Need for Fuzzy . 7. Interpretation of the Main Result A Crisp \Exclusive Or" . Need for the Least . • Reminder: the least sensitive operation is For t-Norms and t- . f⊕(a; b) = min(max(a; b); max(1 − a; 1 − b)): Definition of a Fuzzy . Main Result • Fact: in 2-valued logic, \exclusive or" ⊕ can be de- Interpretation of the . scribed in terms of the \inclusive or" operation _ as Fuzzy \Exclusive Or" . a ⊕ b , (a _ b)&:(a & b): Home Page • Natural idea: Title Page { replace _ with the least sensitive \or"-operation JJ II f_(a; b) = max(a; b), J I { replace & with the least sensitive \and"-operation Page8 of 13 f&(a; b) = min(a; b), and Go Back { replace : with the least sensitive negation opera- Full Screen tion f:(a) = 1 − a, Close • Result: we get the expression given in the Theorem. Quit Need for Fuzzy . 8. Proof of the Main Result: 1st Condition A Crisp \Exclusive Or" . Need for the Least . • Reminder: f (a; b) = min(max(a; b); max(1−a; 1−b)). ⊕ For t-Norms and t- . • We need to prove the following two conditions: Definition of a Fuzzy . Main Result { 1st: that this function f⊕(a; b) satisfies the follow- ing condition with k = 1: Interpretation of the . Fuzzy \Exclusive Or" . 0 0 0 0 jf(a; b) − f(a ; b )j ≤ k · max(ja − a j; jb − b j); Home Page { 2nd: that no other \exclusive or" operation satisfies Title Page this property. JJ II • 1st condition: let us prove that for every " > 0, if J I 0 0 ja − a j ≤ " and jb − b j ≤ ", then Page9 of 13 0 0 jf⊕(a; b) − f⊕(a ; b )j ≤ ": Go Back • It is known: that the functions min(a; b), max(a; b), Full Screen and 1 − a satisfy the above condition with k = 1. Close Quit Need for Fuzzy . 9. Proof of the Main Result (cont-d) A Crisp \Exclusive Or" . Need for the Least . 0 0 • Known results: if ja − a j ≤ " and jb − b j ≤ ", then the For t-Norms and t- . following three inequalities hold: Definition of a Fuzzy . 0 0 j max(a; b) − max(a ; b )j ≤ "; Main Result Interpretation of the . j(1 − a) − (1 − a0)j ≤ "; and j(1 − b) − (1 − b0)j ≤ ": Fuzzy \Exclusive Or" . • From the result above, by using the condition for the Home Page max operation, we conclude that Title Page 0 0 j max(1 − a; 1 − b) − max(1 − a ; 1 − b )j ≤ ": JJ II • Now, from the results above, by using the condition for J I the min operation, we conclude that Page 10 of 13 j min(max(a; b); max(1 − a; 1 − b)) Go Back − min(max(a0; b0); max(1 − a0; 1 − b0))j ≤ ": Full Screen • The statement is proven. Close Quit Need for Fuzzy . 10. Fuzzy \Exclusive Or" Operations f(a; b) Which A Crisp \Exclusive Or" . Are the Least Sensitive on Average Need for the Least . For t-Norms and t- . • Idea: select f so that on average, the change in a and b Definition of a Fuzzy . leads to the smallest possible change ∆c in c = f(a; b). Main Result • Assumption: ∆a and ∆b are independent random vari- Interpretation of the . 2 ables with 0 mean and small variance σ . Fuzzy \Exclusive Or" . • Objective: estimate ∆c = f(a + ∆a; b + ∆b) − f(a; b): Home Page • Since ∆a and ∆b are small, we can keep only linear Title Page terms in the Taylor series of ∆c w.r.t.
Details
-
File Typepdf
-
Upload Time-
-
Content LanguagesEnglish
-
Upload UserAnonymous/Not logged-in
-
File Pages13 Page
-
File Size-