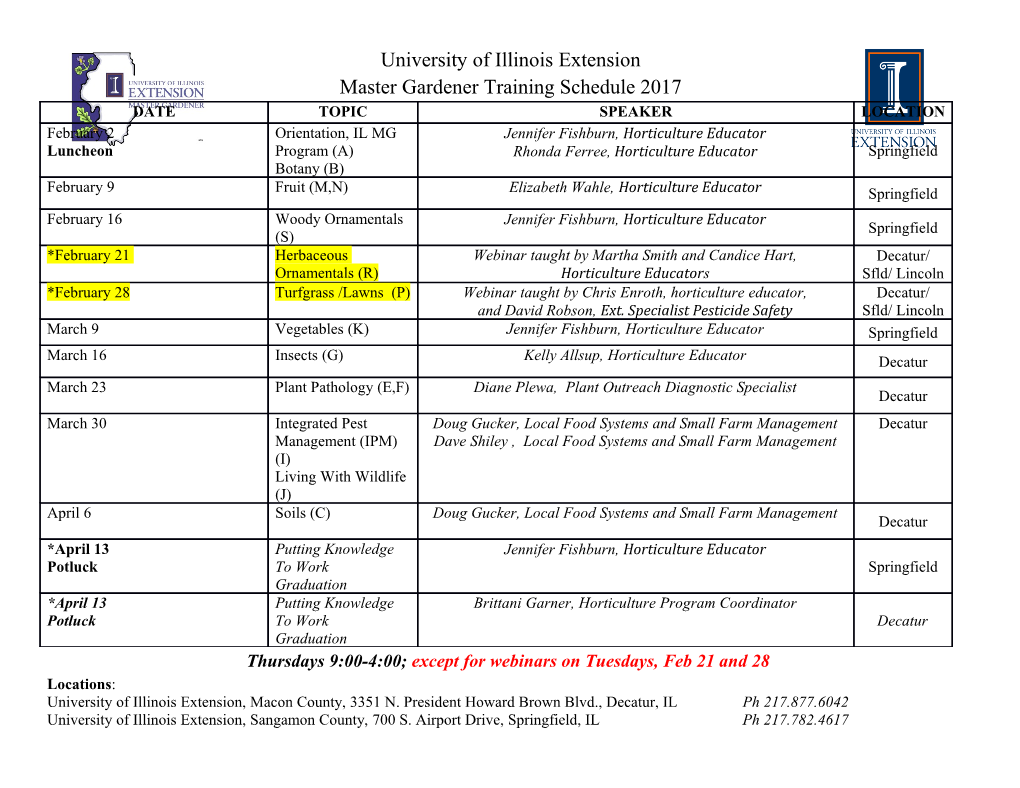
On the fractional stochastic integration for random non-smooth integrands Nikolai Dokuchaev School of Electrical Engineering, Computing and Mathematical Sciences, Curtin University Submitted: October 5, 2015. Revised: April 19, 2020 Abstract The paper suggests a way of stochastic integration of random integrands with respect to fractional Brownian motion with the Hurst parameter H > 1/2. The integral is defined initially on the processes that are ”piecewise” predictable on a short horizon. Then the inte- gral is extended on a wide class of square integrable adapted random processes. This class is described via a mild restriction on the growth rate of the conditional mean square error for the forecast on an arbitrarily short horizon given current observations; differentiability of H¨older property of any kind or degree is not required for the integrand. The suggested integration can be interpreted as foresighted integration for integrands featuring correspond- ing restrictions on the forecasting error. This integration is based on Itˆo’s integration and does not involve Malliavin calculus or Wick products. In addition, it is shown that these stochastic integrals depend continuously on H at H =1/2 + 0. Key words: stochastic integration, fractional Brownian motion, random integrands, Hurst parameter, forecast error. Mathematics Subject Classification (2010): 60G22 arXiv:1510.01207v8 [math.PR] 19 Apr 2020 1 Introduction The paper considers stochastic integration of random integrands with respect to fractional Brow- nian motion. These integrals can be defined using different approaches; see review and discussion in [1, 2, 10, 13, 14, 15, 16, 17, 19, 26, 28, 29, 30, 35]. This integration has many applications in statistical modelling, especially for quantitative finance; see e.g. [3, 4, 6, 8, 9, 12, 21, 22, 23, 24, 26, 31, 32, 33]. Special statistical inference methods developed for these models; see e.g. [11, 18, 20, 27]. Naturally, the integral can be defined as a Riemann sum for piecewise constant in time integrands; the problem is an extension on more general classes of integrands. There is a special approach base on the so-called the Wick product rather than Riemann sums; see, e.g. [3, 4, 6, 9, 17]. This approach allows integrands of quite general type but the features the 1 Wick product makes the corresponding integrals quite distinctive from the integrals based on the Riemann sums. Currently, stochastic integrals with respect to the fractional Brownian motion BH with a Hurst parameter H (1/2, 1) are defined for random integrands in the following cases. ∈ (i) The integral is defined for the integrands that are pathwise H¨older with index p> 1 H; − see, e.g., Theorem 21 in [19] and [13, 35]. (ii) The integral is defined pathwise for integrands that has q-bounded variation with q < 1/(1 H); see, e.g., [34, 7]. − (iii) The integral is defined as a Skorohod integral for integrands γ such that γ is L -integrable ∇ p for p> (1/2 H)−1, where is the Gross-Sobolev derivative ( Theorem 3.6 [15] (2003) or − ∇ Theorem 6.2 [16]). This approach is based on anticipating integrals (see, e.g., [3, 9, 14, 17], and review in [16]). It can be noted that this requires certain differentiability of the integrand in the sense of existence of g or the fractional derivative [1]. ∇ We exclude from this list the integrals based on the Wick product and integrals for piecewise constant integrands. In this paper, we readdress stochastic integration of random integrands with respect to frac- tional Brownian motion. We suggests an integration scheme allowing to extend the class of admissible random integrands known in the literature. In particular, we show that stochastic integral with respect to the fractional Brownian motion B with H (1/2, 1) is well defined on H ∈ a wide class of L2-integrable processes with a mild restriction on the growth rate for conditional variance for a short term forecast. It is not required that the integrands g satisfy H¨older con- dition, or have finite p-variation, or γ is L - integrable, or a fractional derivative exists. The ∇ p description of this class does not require to use Malliavin calculus as in [15, 16] and does not use any kind of derivatives. We use a modification of the classical Riemann sums. Instead of the standard extension of the Riemann sums from the set of piecewise constant integrands, we used an extension of different sums from processes being ”piecewise predictable” on a short horizon that are not necessarily piecewise constant. More precisely, these integrands are adapted to the filtration generated by the observations being frozen at grid time points. In other words, this ”piecewise predictable” class includes all integrands that are predictable without error on a fixed time horizon that can be arbitrarily short. The corresponding stochastic integral is represented via sums of integrals of two different types: one type is a standard Itˆo’s integral, and another type is a Lebesgue integral for random integrands. In the second step, we extended this integral on a wide class of L2-integrable processes (Theorem 3.1 below); the resulting integrals is denoted as d B The corresponding condition · F H allows a simple formulation that does not require MalliavinR calculus used in [15, 16]. This 2 theorem implies prior estimates of the stochastic integral via a norm of a random integrand (Corollary 3.1). Furthermore, it is shown that the stochastic integrals depend continuously on H at H = 1/2 + 0 under some additional mild restrictions on the growth rate for the conditional variance of the future values given current observations (Theorem 4.1 below). The paper is organized as follows. Section 2 presents some definitions. In Section 3, we present the definition of the new type of integral and some convergence results and prior esti- mates. In Section 4, we show some continuity of the new integral with respect to a variable Hurst parameter. The proofs are given in Section 5. 2 Some definitions We are given a probability space (Ω, F, P), where Ω is a set of elementary events, F is a complete σ-algebra of events, and P is a probability measure. We assume that B (t) R is a fractional Brownian motion with the Hurst parameter { H }t∈ H (1/2, 1) defined as described in [26] such that B (0) = 0 and ∈ H t BH (t)= f(t, r)dB(r), (2.1) Z−∞ where t 0 and ≥ f(t, r) =∆ c (t r)H−1/2I + c ((t r)H−1/2 ( r)H−1/2)I . (2.2) H − r≥0 H − − − r<0 Here c = 1/Γ(H + 1/2), Γ is the Gamma function, I is the indicator function, and B(t) R H { }t∈ is a standard Brownian motion such that B(0) = 0; we denote by dB the standard Itˆo’s · integration. R Let d =∆ c (H 1/2). H H − For T > 0, τ [0, T] and g L (0, T), set ∈ ∈ 2 T G (τ, T, g) =∆ d (t τ)H−3/2g(t)dt. (2.3) H H − Zτ By the property of the Riemann–Liouville integral, there exists c> 0 such that G ( , T, g) c g . (2.4) k H · kL2 (s,T) ≤ k kL2(s,T) It can be noted that this c is independent on H (1/2, 1). ∈ Let G be the filtration generated by the process B(t). { t} Let T > 0 be given. 3 Let L22 be the linear normed space formed as the completion in L2-norm of the set of all Gt- 1/2 T 2 adapted bounded measurable processes γ(t), t [0, T ], with the norm γ L = E γ(t) dt) . ∈ k k 22 0 For ε> 0, let Xε be the set of all γ L22 such that there exists an integern> 0 and a set ∈ R of non-random times T = T n [0, T ], where T = 0, T = T , and 0 < T T ε, such { k}k=1 ⊂ 0 n k+1 − k ≤ that γ(t) is G -measurable for t [T , T ). Tk ∈ k k+1 In particular, the set X includes all γ L such that γ(t) is G -measurable for all ε ∈ 22 t−ε t [0, T ]. ∈ Let X =∆ X . ∪ε>0 ε For the brevity, we sometimes denote L (Ω, G , P) by L (Ω), p 1. p T p ≥ Let X be the set of all γ L such that there exists an integer n > 0 and a set of ε,PC ∈ 22 non-random times T = T n [0, T ], where n > 0 is an integer, T = 0, T = T , and { k}k=1 ⊂ 0 n T T ε, such that γ(t)= γ(T ) for t [T , T ). k+1 − k ≥ k ∈ k k+1 3 The main result: integration for random integrands For any γ X , it is naturally to define the stochastic integral with respect to B in ∈ ε,PC H L1(Ω, GT , P) as the Riemann sum n γ(T )(B (T ) B (T )). k H k+1 − H k Xk=0 If γ L is such that this sum has a limit in probability as n + , and this limit is ∈ 22 → ∞ independent on the choice of T n n , then we call this limit the integral T γ(t)d B (t). { k }k=1 0 RS H The classes of admissible deterministic integrands γ are known; see, e.g.R [28, 29]. However, there are some difficulties with identifying classes of admissible random γ. The present paper suggests a modification of the stochastic integral based on the extension from X, i.e.
Details
-
File Typepdf
-
Upload Time-
-
Content LanguagesEnglish
-
Upload UserAnonymous/Not logged-in
-
File Pages26 Page
-
File Size-