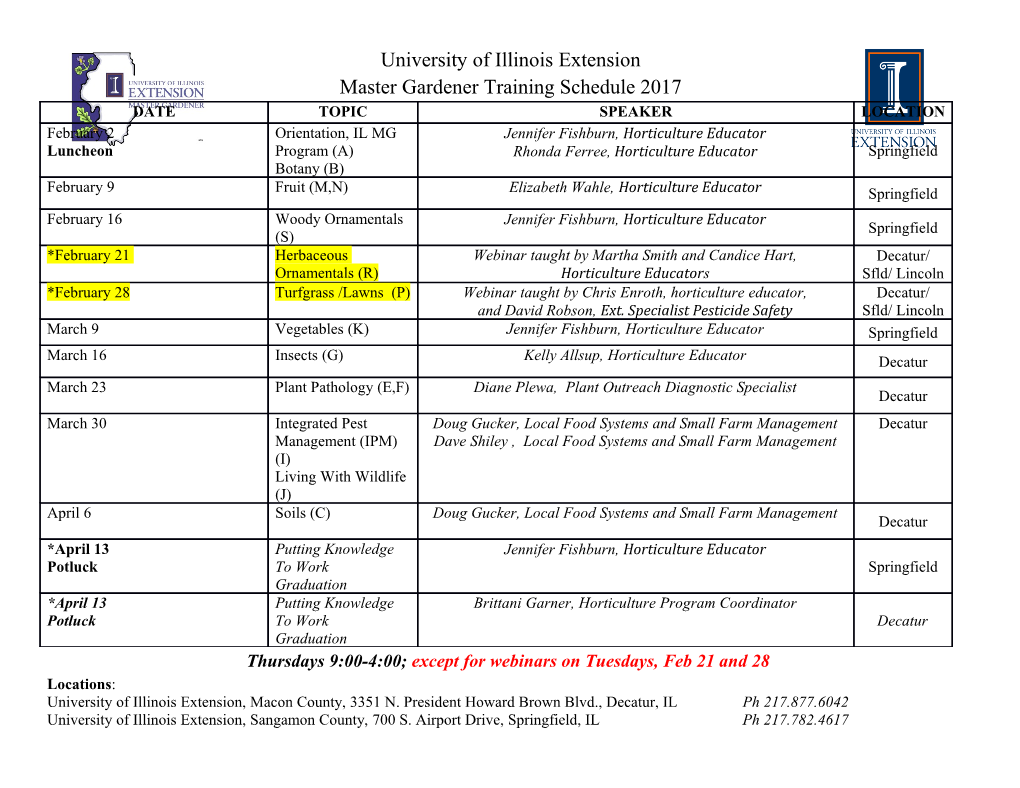
Spring 2020: Venu: Haag 313, Time: M/W 4-5:15pm ECE 5582 Computer Vision Lec 04: Filtering and Edge Features Zhu Li Dept of CSEE, UMKC Office: FH560E, Email: [email protected], Ph: x 2346. http://l.web.umkc.edu/lizhu slides created with WPS Office Linux and EqualX LaTex equation editor Z. Li: ECE 5582 Computer Vision, 2020 p.1 Outline Recap of Lec 03 . Perspective Projection &Homography . SVD and an example . HW-1 Image Filtering – A Quick Review . Image Filtering and Correlation . Edge Detection Edge Descriptors . HOG – Histogram of Oriented Gradients Z. Li: ECE 5582 Computer Vision, 2020 p.2 Camera Projection: Intrinsic + Extrinsic Parameters Camera Intrinsic + Extrinsic Parameters: éxù éuù éa 0 u0 ùér11 r12 r13 tx ùê ú ê ú ê úê ú y x = K[R t] X w v = 0 b v r r r t ê ú ê ú ê 0 úê 21 22 23 y úêzú ê ú ê úê ú ë1û ë0 0 1 ûër31 r32 r33 tz ûê ú ë1û . Intrinsic: camera center:[u0, v0], focus length/aspect ratio: . Extrinsic: Rotation R=RxRyRz, translation t=[tx, ty, tz] R,t jw X kw Ow x iw Z. Li: ECE 5582 Computer Vision, 2020 p.3 Homography In general, homography H maps 2d points according to, x’=Hx ′ = ℎ ℎ ℎ ′ ℎ ℎ ℎ . Up to′ a sℎcaleℎ, asℎ [x, y, w]=[sx, sy, sw], so H has 8 DoF Affine Transform: . 6 DoF: Contains a translation [t1, t2], and invertable affine matrix A[2x2] H = 1 4 Similarity 0Tran0sfo1rm, . 4DoF: a rigid transform that preserves distance if s=1: H = X − sin X cos Z. Li: ECE 5582 Compute0r Vision, 2020 0 1 p.4 Homography Estimation – SVD Pseudo Inv In matrix form, we have, Ah = 0 If we have more than 4, then the system is over-determined, we will find a solution by least squares, computationally via SVD Pseudo Inverse: . SVD: . Pseudo inverse (minimizing least square error) . Details: htt=ps://Xwww.ecse.rpi.edu/~qji/CV/svd_review.pdf + + Z. Li: ECE 5582 Computer Vision, 202=0 X p.5 HW-1 Compute HSV color histogram for CIFAR 100 image retrieval . rgb2hsv . uniform quantize HSV space . euclidean distance hist ranking . try other metrics, like KL distance as bonus Compute Homography Z. Li: ECE 5582 Computer Vision, 2020 p.6 Outline Recap of Lec 03 . SVD and an example Image Filtering – A Quick Review . Image Filters . Edge Detection Edge Feature Z. Li: ECE 5582 Computer Vision, 2020 p.7 What is an Image ? The famous Lenna…. https://www.wired.com/story/finding-lena-the-patron-saint-of- jpegs/ Lenna, 1971 Lena at ICIP 2015, Quebec City Lenna, 2019 Z. Li: ECE 5582 Computer Vision, 2020 p.8 What is an image? We can think of a (grayscale) image as a function, f, from R2 to R (or a 2D signal): . f (x,y) gives the intensity at position (x,y) f (x, y) x y . A digital image is a discrete (sampled, quantized) version of this function Z. Li: ECE 5582 Computer Vision, 2020 p.9 Image as a function: I=f(x,y); A grid (matrix) of intensity values 92 [R(x,y), G(x,y), B(x,y)]=[90, 0, 54] 221 [249, 215, 203] 144 [220, 15, 77] (common to use one byte per value: 0 = black, 255 = white) Z. Li: ECE 5582 Computer Vision, 2020 p.10 Motivation: Why Filtering ? Some use cases… Edge detection Deep Learning: VGG16 Z. Li: ECE 5582 Computer Vision, 2020 p.11 Image filtering – Looking at pixel neighbors Modify the pixels in an image based on some function of a local neighborhood of each pixel . Discrete form: 10 5 3 Some function 4 5 1 7 1 1 7 Local image data Modified image data Z. Li: ECE 5582 Computer Vision, 2020 p.12 Linear filtering One simple version: linear filtering (cross-correlation, convolution) . Replace each pixel by a linear combination of its neighbors The prescription for the linear combination is called the “kernel” (or “mask”, “filter”) 10 5 3 0 0 0 4 6 1 0 .5 0 8 1 1 8 0 1 .5 Local image kernel Modified image data data Source: L. Zhang Z. Li: ECE 5582 Computer Vision, 2020 p.13 Cross-correlation Cross-Correlation: . No flipping of kernel Let F be the image, H be the kernel (of size 2k+1 x 2k+1), and G be the output image This is called a cross-correlation operation: Z. Li: ECE 5582 Computer Vision, 2020 p.14 Convolution Same as cross-correlation, except that the kernel is “flipped” (horizontally and vertically), called convolution = ∗ Convolution is commutative and associative . F=G*h + G*f = G*(h+f) . F=G*h*f = G*(h*f) Z. Li: ECE 5582 Computer Vision, 2020 p.15 Mean filtering Find the average 0 0 0 0 0 0 0 0 0 0 0 0 0 0 0 0 0 0 0 0 0 10 20 30 30 30 20 10 0 0 0 90 90 90 90 90 0 0 0 20 40 60 60 60 40 20 1/9 11/9 11/9 0 0 0 90 90 90 90 90 0 0 0 30 60 90 90 90 60 30 11/9 11/9 1/9 0 0 0 90 90 90 90 90 0 0 0 30 50 80 80 90 60 30 0 0 0 90 0 90 90 90 0 0 0 30 50 80 80 90 60 30 1/19 11/9 11/9 = 0 0 0 90 90 90 90 90 0 0 0 20 30 50 50 60 40 20 * 0 0 0 0 0 0 0 0 0 0 10 20 30 30 30 30 20 10 0 0 90 0 0 0 0 0 0 0 10 10 10 0 0 0 0 0 0 0 0 0 0 0 0 0 0 0 Z. Li: ECE 5582 Computer Vision, 2020 p.16 Linear filters: examples Identity filter: 0 0 0 0 1 0 = * 0 0 0 Original Identical image Source: D. Lowe Z. Li: ECE 5582 Computer Vision, 2020 p.17 Linear filters: examples Shift Filter 0 0 0 1 0 0 = * 0 0 0 Original Shifted left By 1 pixel Source: D. Lowe Z. Li: ECE 5582 Computer Vision, 2020 p.18 Linear filters: examples Average/Blurring 1 1 1 1 1 1 = * 1 1 1 Original Blur (with a mean filter) Source: D. Lowe Z. Li: ECE 5582 Computer Vision, 2020 p.19 Linear filters: examples Sharpen: remove the average. 0 0 0 1 1 1 0 2 0 - 1 1 1 = * 0 0 0 1 1 1 Sharpening filter Original (accentuates edges) Source: D. Lowe Z. Li: ECE 5582 Computer Vision, 2020 p.20 Smoothing with box filter Box Filter: Box filter in integral image domain: . much faster, just 4 ADD operations. More details in CABOX coverage Source: D. Forsyth Z. Li: ECE 5582 Computer Vision, 2020 p.21 Gaussian Kernel Gaussian Kernel with scale Matlab: h=fspecial('gaussian', 5, 1.0); Z. Li: ECE 5582 Computer Vision, 2020 p.22 Gaussian Filters – A Scale Space Approximation Gaussian Blur Matlab: n=8;s = 1.25.^[1:n]; m=fix(6.*s); figure(30); for k=1:8 subplot(2,4,k); h = fspecial('gaussian', m(k), s(k)); imagesc(h); title(sprintf('s = %1.1f', s(k))); end figure(31); subplot(3,3,1); imshow(im); title('f_0(1.0)'); for k=1:n subplot(3,3,k+1); h = fspecial('gaussian', m(k), s(k)); f = imfilter(im, h); imshow(f); title(sprintf('f_%d(%1.1f)', k+1, s(k))); end Z. Li: ECE 5582 Computer Vision, 2020 p.23 Gaussian filter properties Successive Gaussian filtering . convolution of Gaussian is still Gaussian * = new kernel sigma: h1+ h2 Matlab: h1=fspecial('gaussian', 11, 1.2); h2=fspecial('gaussian', 11, 2.0); h3 = conv2(h1, h2); h4=fspecial('gaussian', 11, 2.0+1.2); Z. Li: ECE 5582 Computer Vision, 2020 p.24 Separatable Fitler Gaussian filter is separatable: u v h verify via SVD: . h = fspecial('gaussian', 11, 1.2); . [u,s,v]=svd(h); plot(diag(s)); Z. Li: ECE 5582 Computer Vision, 2020 p.25 Sharpening revisited What does blurring take away? – = original smoothed (5x5) detail Let’s add it back: + α = original detail sharpened Source: S. Lazebnik Z. Li: ECE 5582 Computer Vision, 2020 p.26 Sharpen filter - LoG Laplacian of Gaussian blurred image image unit impulse (identity) scaled impulse Gaussian Laplacian of Gaussian Z. Li: ECE 5582 Computer Vision, 2020 p.27 Sharpen filter unfiltered filtered Z. Li: ECE 5582 Computer Vision, 2020 p.28 Convolution in the real world Physical world convolutions Camera Shake = * Source: Fergus, et al. “Removing Camera Shake from a Single Photograph”, SIGGRAPH 2006 Bokeh: Blur in out-of-focus regions of an image. Source: http://lullaby.homepage.dk/diy-camera/bokeh.html Z. Li: ECE 5582 Computer Vision, 2020 p.29 Filtering in Matlab Area of support for the operations To give the same n1 x m1 output, need to padding the edge . Default is zero padding . Also replicate the last edge pixel . Or, mirroring (used in MPEG codec) Z. Li: ECE 5582 Computer Vision, 2020 p.30 Image Filtering, Sweet Deal with Matlab It is such a nice tool . Main filter operation: im2 = imfilter(im, h, ‘replicate’) . Design your filter: h= fspecial(‘filter_type’, kernel_size, options) Filter Design Examples: . Sobel . Laplacian, Laplacian of Gaussian . Gaussian, Difference of Gaussian (SIFT) Z. Li: ECE 5582 Computer Vision, 2020 p.31 Matlab Image Filtering Example %%%%%%%%%%%%%%%%%%%%%%%%%%%%%% % image filters %%%%%%%%%%%%%%%%%%%%%%%%%%%%%% im = imread('../pics/Lenna.png'); im = rgb2gray(im); im1 = double(im(201:320, 201:320)); % edge filters h{1} = fspecial('sobel'); h{2} = fspecial('laplacian', 0.25); h{3} = fspecial('log', 7, 0.25); % gaussians sigmas = [1.226, 1.554, 1.946]; for k=1:length(sigmas) h{3+k} = fspecial('gaussian',11, sigmas(k)); end % diff of gaussian h{7} = (h{6} - h{4}); h{7} = h{7}/sum(sum(h{7})); h{8} = h{5} - h{4}; h{8} = h{8}/sum(sum(h{8})); for k=1:8 fprintf('\n k=%d', k); figure(26); subplot(2,4,k); grid on; hold on; colormap('gray'); imagesc(h{k}); figure(27); subplot(2,4,k); imshow(imfilter(im, h{k}, 'replicate')); end Z.
Details
-
File Typepdf
-
Upload Time-
-
Content LanguagesEnglish
-
Upload UserAnonymous/Not logged-in
-
File Pages56 Page
-
File Size-