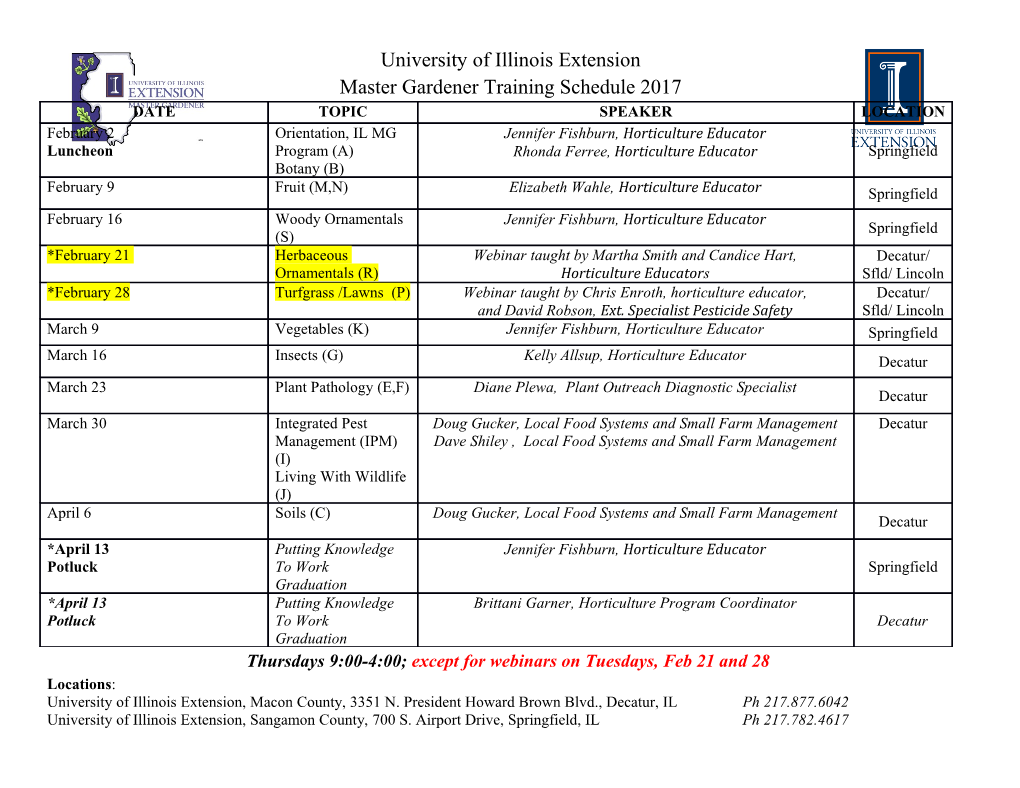
Computing Effect Sizes for Clustered Randomized Trials Terri Pigott, C2 Methods Editor & Co-Chair Professor, Loyola University Chicago [email protected] The Campbell Collaboration www.campbellcollaboration.org Computing effect sizes in clustered trials • In an experimental study, we are interested in the difference in performance between the treatment and control group • In this case, we use the standardized mean difference, given by YYTC− d = gg Control group sp mean Treatment group mean Pooled sample standard deviation Campbell Collaboration Colloquium – August 2011 www.campbellcollaboration.org 1 Variance of the standardized mean difference NNTC+ d2 Sd2 ()=+ NNTC2( N T+ N C ) where NT is the sample size for the treatment group, and NC is the sample size for the control group Campbell Collaboration Colloquium – August 2011 www.campbellcollaboration.org TREATMENT GROUP CONTROL GROUP TT T CC C YY,,..., Y YY12,,..., YC 12 NT N Overall Trt Mean Overall Cntl Mean T Y C Yg g S 2 2 Trt SCntl 2 S pooled Campbell Collaboration Colloquium – August 2011 www.campbellcollaboration.org 2 In cluster randomized trials, SMD more complex • In cluster randomized trials, we have clusters such as schools or clinics randomized to treatment and control • We have at least two means: mean performance for each cluster, and the overall group mean • We also have several components of variance – the within- cluster variance, the variance between cluster means, and the total variance • Next slide is an illustration Campbell Collaboration Colloquium – August 2011 www.campbellcollaboration.org TREATMENT GROUP CONTROL GROUP Cntl Cluster mC Trt Cluster 1 Trt Cluster mT Cntl Cluster 1 TT T T CC C C YY,...ggg Y ,..., Y YY11,... 1n ggg YCC ,..., Y { 11 1n } { mmnTT1 } { } { mmn1 } C Trt Cluster 1 Mean Trt Cluster mT Mean Cntl Cluster 1 Mean Cntl Cluster m Mean T T C C Y ••• Y T Y ••• Y C 1g m g 1g m g Overall Cntl Mean Overall Trt Mean T Y C Ygg gg Assume equal sample size, n, within clusters Campbell Collaboration Colloquium – August 2011 www.campbellcollaboration.org 3 The problem in cluster randomized trials • Which mean is the appropriate mean to represent the differences between the treatment and control groups? – The cluster means? – The overall treatment and control group means averaged across clusters? • Which variance is appropriate to represent the differences between the treatment and control groups? – The within-cluster variance? – The variance among cluster means? – The total variance? Campbell Collaboration Colloquium – August 2011 www.campbellcollaboration.org Three different components of variance • The next slides outline the three components of variance that exist in a cluster randomized trial • These components of variance correspond to the variances that we would estimate when analyzing a cluster randomized trial using hierarchical linear models Campbell Collaboration Colloquium – August 2011 www.campbellcollaboration.org 4 Pooled within-cluster sample variance • One component of variance is within-cluster variation • This is computed as the pooled differences between each observation and its cluster mean mnTC mn ()YYTT22 () YY CC ∑∑ij− igg+ ∑∑ ij− i S 2 = ij==11 ij == 11 W NM− M is number of clusters: M = mT + mC N is total sample size: N = n mT + n mC = NT + NC with equal cluster sample sizes, n Campbell Collaboration Colloquium – August 2011 www.campbellcollaboration.org TREATMENT GROUP CONTROL GROUP Cntl Cluster mC Trt Cluster 1 Trt Cluster mT Cntl Cluster 1 TT T T CC C C YY,...ggg Y ,..., Y YY11,... 1n ggg YCC ,..., Y { 11 1n } { mmnTT1 } { } { mmn1 } C Trt Cluster 1 Mean Trt Cluster mT Mean Cntl Cluster 1 Mean Cntl Cluster m Mean T T C C Y ••• Y T Y ••• Y C 1g m g 1g m g Overall Cntl Mean Overall Trt Mean T Y C Ygg gg 2 Within-cluster variance SW Campbell Collaboration Colloquium – August 2011 www.campbellcollaboration.org 5 Pooled within-group (treatment and control) sample variance of the cluster means • Another component of variation is the variance among the cluster means within the treatment and control groups • This is computed as the pooled difference between each cluster mean and its overall group (treatment and control) mean mmTC ()()YYTT22 YY CC ∑∑iiggg−+ ggg− S 2 = ii==11 B mmTC+−2 Campbell Collaboration Colloquium – August 2011 www.campbellcollaboration.org TREATMENT GROUP CONTROL GROUP Cntl Cluster mC Trt Cluster 1 Trt Cluster mT Cntl Cluster 1 TT T T CC C C YY,...ggg Y ,..., Y YY11,... 1n ggg YCC ,..., Y { 11 1n } { mmnTT1 } { } { mmn1 } C Trt Cluster 1 Mean Trt Cluster mT Mean Cntl Cluster 1 Mean Cntl Cluster m Mean T T C C Y ••• Y T Y ••• Y C 1g m g 1g m g Overall Cntl Mean Overall Trt Mean T Y C Ygg gg 2 Variance between cluster S B means Campbell Collaboration Colloquium – August 2011 www.campbellcollaboration.org 6 Total pooled within-group (treatment and control) variance • The total variance is the variation among the individual observations and their group (treatment and control) overall means mnTC mn ()YYTT22 () YY CC ∑∑ij−gg+ ∑∑ ij − gg S 2 = ij==11 ij == 11 T N − 2 Campbell Collaboration Colloquium – August 2011 www.campbellcollaboration.org TREATMENT GROUP CONTROL GROUP Cntl Cluster mC Trt Cluster 1 Trt Cluster mT Cntl Cluster 1 TT T T CC C C YY,...ggg Y ,..., Y YY11,... 1n ggg YCC ,..., Y { 11 1n } { mmnTT1 } { } { mmn1 } C Trt Cluster 1 Mean Trt Cluster mT Mean Cntl Cluster 1 Mean Cntl Cluster m Mean T T C C Y ••• Y T Y ••• Y C 1g m g 1g m g Overall Cntl Mean Overall Trt Mean T Y C Ygg gg 2 S Total pooled within-group variance T (treatment and control) Campbell Collaboration Colloquium – August 2011 www.campbellcollaboration.org 7 Just a little distributional theory • As usual, we will assume that each of our observations within the treatment and control group clusters are normally T C distributed about their cluster means, µ i and µ i with 2 common within-cluster variance, σ W, or TT2 T YNij~ (µ i ,σ W ), i== 1,..., mjn ; 1,..., CC2 C YNij~ (µ i ,σ W ), i== 1,..., mjn ; 1,..., Campbell Collaboration Colloquium – August 2011 www.campbellcollaboration.org Just a little more distributional theory • We also assume that our clusters are sampled from a population of clusters (making them random effects) so that the cluster means have a normal sampling distribution with T C 2 means µ • and µ • and common variance, σ B TT2 T µµiB~(Nimg ,σ ),1,...,= CC2 C µµiB~(Nimg ,σ ),1,...,= Campbell Collaboration Colloquium – August 2011 www.campbellcollaboration.org 8 The three component s of variance are related: 22 2 σσTW=+ σ B Campbell Collaboration Colloquium – August 2011 www.campbellcollaboration.org The intraclass correlation, ρ • This parameter summarizes the relationship among the three variance components • We can think of ρ as the ratio of the between cluster variance and the total variance • ρ is given by 22 σσBB ρ ==22 2 σσBW+ σ T Campbell Collaboration Colloquium – August 2011 www.campbellcollaboration.org 9 Why is the intraclass correlation important? • Later, we will see that we need the intraclass correlation to obtain the values of our standardized mean difference and its variance • Also, if we know the intraclass correlation, we can obtain the value of one of the variances knowing the other two. For example: 22 σρσρWB=(1− ) / 22 σρσWT=(1− ) Campbell Collaboration Colloquium – August 2011 www.campbellcollaboration.org So far, we have • Discussed the structure of the data • Seen how to compute three different sampling variances: 2 2 2 S W, S B, and S T • Discussed the underlying distributions of our clustered data • Seen how the variance components in the distributions of our data are related to one another • Next we will see how to estimate the variance components using our sampling variances • (Yes, we are building up to the effect sizes…) Campbell Collaboration Colloquium – August 2011 www.campbellcollaboration.org 10 2 Estimator of σ W 2 • As we might expect, we estimate σ W using our estimate of the within-cluster sampling variance, or, 22 σˆWW= S Campbell Collaboration Colloquium – August 2011 www.campbellcollaboration.org 2 Estimator of σ B 2 • Unfortunately, the estimate of σ B is not as straightforward as 2 for σ W 2 • This is due to the fact that S B includes some of the within- cluster variance. 2 • The expected value for S B is σ 2 σ 2 + W B n Campbell Collaboration Colloquium – August 2011 www.campbellcollaboration.org 11 2 Thus, an estimator for σ B is S 2 σˆ 22=S −W BBn Campbell Collaboration Colloquium – August 2011 www.campbellcollaboration.org 2 And, given prior information, an estimate of σ T is n −1 ˆ 22⎛⎞ 2 σTB=+SS⎜⎟ W ⎝⎠n Campbell Collaboration Colloquium – August 2011 www.campbellcollaboration.org 12 Now, we are ready for computing effect sizes • There are 3 possibilities for computing an effect size in a clustered randomized trial • These correspond to the 3 different components of variance we have been discussing • The choice among them depends on the research design and the information reported in the other studies in our meta- analysis Campbell Collaboration Colloquium – August 2011 www.campbellcollaboration.org The most common: δW • This effect size is comparable to the standardized mean difference computed from single site designs TC µµgg− δW = σW Campbell Collaboration Colloquium – August 2011 www.campbellcollaboration.org 13 We estimate δW using dW TC Trt & Cntl means YYgg− gg dW = SW Pooled within-cluster variance Campbell Collaboration Colloquium – August 2011 www.campbellcollaboration.org Variance of dW • Variance of dW depends on the intraclass correlation, sample sizes,
Details
-
File Typepdf
-
Upload Time-
-
Content LanguagesEnglish
-
Upload UserAnonymous/Not logged-in
-
File Pages36 Page
-
File Size-